350 rub
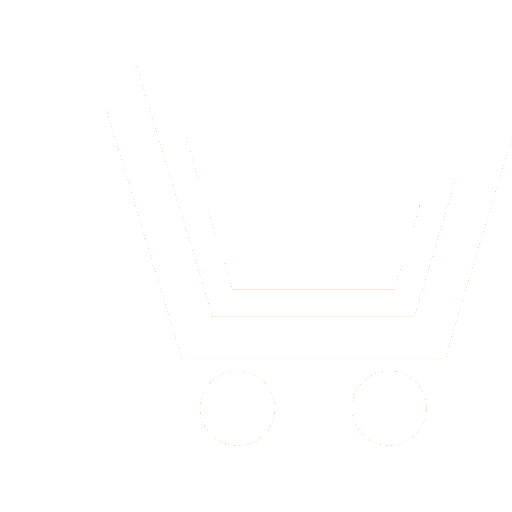
Journal Information-measuring and Control Systems №2 for 2010 г.
Article in number:
Intellectualization of the Pattern Recognition System Based on Classifier Performance Comparing
Authors:
V.G. Baranov, V.R. Milov, J.H. Zaripova, A.Yu. Epshtein
Abstract:
In recent years analytical information processing tools usually include the methods of data mining. Among those methods classi-fication procedures fill a highly important place. This article presents an approach to the intellectualization of classification pro-cedures. With that end in view the performance of applied classification procedures is estimated. Those estimations are formed by experts using either the results of solving the applied tasks or on basis of statistical modelling. Сlassification tasks are described by series of characteristics, including the number of classes, the number of attributes, the size of the learning sampling, etc. As a result of learning for different types of tasks the preferable types of classiers and their learning procedures are determined.
In this article the special attention is paid to the methods of classifier performance estimation. For the task of binary classification the dependences of alpha error likelihood and beta error likelihood from the threshold value in decision rule are obtained. Using those likelihoods receiver operating characteristics (ROC) graphs are plotted. For the classifier performance comparing the use of the area under an ROC Curve (AUC) is suggested. In addition to it, an integral parameter of classifier precision is suggested, based on the value of empirically determined risk, averaged by the optimal threshold value. The comparing of classifiers based on linear discriminant analysis, quadratic discriminant analysis, probabilistic neural network, and radial basis function network is carried out by modeling.
Pages: 35-39
References
- Хайкин С.Нейронные сети, полный курс. М.: Вильямс. 2006. 1104 с.
- Милов В.Р., Зарипова Ю.Х. Процедуры интеллектуального распознавания образов с применением нейронных сетей // Восьмой Международный симпозиум «Интеллектуальные системы» (INTELS'2008): Сб. науч. тр. Н.Новгород. 2008. C. 109 - 113.
- Рассел С., Норвиг П. Искусственный интеллект: современный подход. М.: Вильямс. 2006.
- Иган Дж.Теория обнаружения сигналов и анализ рабочих характеристик: пер. с англ. М.: Наука. Главная редакция физико-математической литературы. 1983. 216 с.
- Баранов В.Г., Кондратьев В.В., Милов В.Р., Зарипова Ю.Х. Нейросетевые алгоритмы распознавания образов // Нейрокомпьютеры: разработка, применение. 2007. № 11. С. 20 - 27.
- Баранов В.Г., Милов В.Р., Зарипова Ю.Х. Байесовская методология синтеза нейросетевых классификаторов // Труды НГТУ: Системы обработки информации и управления. Н.Новгород: НГТУ. 2007. Т. 65. Вып. 14. С. 5 - 12.
- Баранов В.Г., Милов В.Р., Зарипова Ю.Х., Суслов Б.А., Шалашов И.В.Свидетельство об официальной регистрации программы для ЭВМ № 2008612308. Программный комплекс статистического моделирования процедур нейросетевой классификации // Программы для ЭВМ. Базы данных. Топологии интегральных микросхем: Официальный бюллетень Российского агентства по патентам и товарным знакам. 2008.
- Милов В.Р., Деревянкин А.В., Зарипова Ю.Х. Алгоритмы структурно-параметрического синтеза нейросетевых классификаторов на основе байесовской методологии // XI Всероссийская научно-техническая конференция «Нейроинформатика-2009»: Сб. науч. тр. М.: МИФИ. 2009. С. 124 - 131.