350 rub
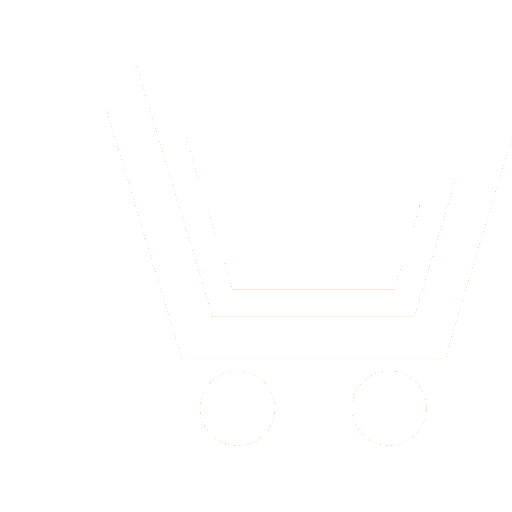
Journal Information-measuring and Control Systems №4 for 2009 г.
Article in number:
On the estimation of a signal covered by background random Poisson noise
Authors:
V. A. Egorov, N. S. Kondybaev
Abstract:
We consider noised observations falling into a rectangular screen. The initial noise model is the trajectory of a random process , where , is a sequence of independent identically distributed random vari-ables, is a sequence of normalizing constants. This trajectory can be regarded as a new model of strong white noise. Note that the part of the trajectory which belongs to given rectangular screen is a random set. It follows from N. N. Lyshenko ([4]?[6]) that this set can weakly converge to some random set , only if , is a Poisson random point process of the density of intensity measure where are parameters of the limit Poisson point random process. Here the density do not depend of . It follows that the random process is a natural model for strong noise. Our observations are the sum of a signal and the noise of this type. Thus the density of intensity measure of our observations is , where is a signal, is a vector of parameters of a signal. The estimation problem of a signal and noise parameters is investigated in this work. Here we use the maximum likelihood method. We apply this method to grouped observations. Initially we divide the screen into some rectangles. We group the observations in accordance with this rectangles. Consistency of derived estimates is considered. In particular, we show that the expectation of the logarithm of likelihood function is maximal for the true value of parameter.
Being investigated algorithm of estimation is analyzed and optimized for concrete type of signal with help of simulation by the Monte Carlo method. We simulate Poisson point random processes using known their representations as a sum of random number of independent identically distributed random variables
Pages: 75
References
- Матерон Ж. Случайные множества и интегральная геометрия. М.: «Мир». 1978. 318 с.
- Molchanov I. Theory of Random Sets, Berlin, Springer, 2005. 488 p.
- Davydov Y., Egorov V. On convergence of empirical point processes // Statistical&Probability Letters. 2006, 76. Рр. 1836-1844.
- Ляшенко Н. Н. Оценивание параметров пуассоновских случайных множеств // Записки научных семинаров ЛОМИ. 1984. Т. 136. С 121-141.
- Ляшенко Н. Н. Слабая сходимость ступенчатых процессов в пространстве замкнутых множеств // Проблемы теории вероятностных распределений. 1983. VIII. С 122-129.
- Ляшенко Н. Н. Геометрическая сходимость случайных процессов и статистика случайных множеств. Известия высших учебных заведений // Математика. 1983. 11. С. 74-81.
- Лидбеттер М. , Ротсен Х., Линдгрен Г. Экстремумы случайных последовательностей и процессов. М.: «Мир». 1989. 391 с.
- Khoshnevisan D. Intersections of Brownian motions. // Expos. Math. 2003. 21. Рр. 97-114.
- Kutoyants Yu. A. Statistical Inference for Spatial Poisson Processes, Universitе - du Maine, Le Mans, France. 1998. 275 p.
- Resnick S.I. Extreme Values, Regular Variation, and Point Processes, Springer - Verlag, Berlin. 1989. 320 p.