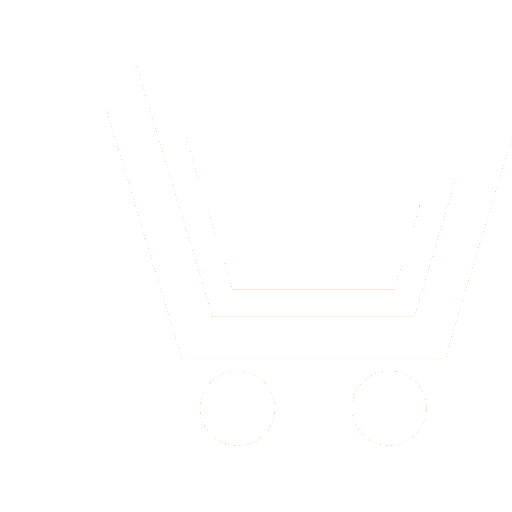
A.V. Gulyaev1, S.V. Pivneva2
1,2 Russian State Social University (Russia, Moscow)
Gulyaev81@gmail.com
When studying a time series, it is traditionally customary to use a generalized mathematical model where a trend component, a cyclic component that describes the repeatability of a cyclic process over time intervals and a random component or noise – this component is responsible for factors hidden from the observer.
It is assumed that the main trend and the cyclical component can be accurately described because they are formed by known factors that can be taken into account within the framework of models.
The discrete wavelet transform is traditionally used to decompose BP into additive components. The first discrete wavelet transform was invented by the Hungarian mathematician Alfred Haar (1910-1920).
In parallel with this method, in the 70s and 80s, the idea of a time series analysis method called SSA (Singular Spectrum Analysis) arose in Russia, the method was called "Caterpillar". During the existence of the method, it has received an extension of its application – automation of the allocation of trend and cyclic components of BP has been developed.
Using the example of a study of the real time series of TRAFFAT, two methods of decomposition time series into additive components are considered. The complexity of determining the initial parameters is compared, the applicability to the decomposition of real time series is tested, and the quality of decomposition by both methods of one reference time series is compared. An assessment of the quality of the decomposition of the time series into components was made, as well as a comparison of the two methods in terms of the complexity of the analyst's application and the possibility of automating the selection of parameters.
Gulyaev A.V., Pivneva S.V. Application of wavelet transformation and singular spectral analysis in time series decomposition. Highly Available Systems. 2024. V. 20. № 2. P. 76−84. DOI: https://doi.org/10.18127/j20729472-202402-06 (in Russian)
- Aleksandrov F.I., Golyandina N.E. Vybor parametrov pri avtomaticheskom vydelenii trendovyh i periodicheskih sostavlyayushchih vremennogo ryada v ramkah podhoda “Gusenica”-SSA. Trudy IV MK «Identifikaciya sistem i zadachi upravleniya». M. 2005. S. 1849–1864 (in Russian).
- Burnaev E.V. Primenenie vejvlet-preobrazovaniya dlya analiza ekonomicheskih vremennyh ryadov. M.: Ekomod. 2006. S. 130–143 (in Russian).
- Golyandina N.E. Avtomatizaciya vydeleniya trendovyh i periodicheskih sostavlyayushchih vremennogo ryada v ramkah metoda «Gusenica» SSA. Metody Algoritmy Programmy. 2004. № 3–4(7–8). S. 58–61 (in Russian).
- Micel' A.A. Analiz zatrat predpriyatij s pomoshch'yu vejvlet-preobrazovaniya. Ekonomicheskij analiz. Teoriya i praktika. 2013. № 46 (349). S. 52–60 (in Russian).
- Porshnev S.V. Issledovanie osobennostej primeneniya metoda singulyarnogo spektral'nogo analiza v zadache analiza i prognozirovaniya vremennyh ryadov: Monografiya. Ul'yanovsk. 2016. S. 21–24 (in Russian).
- Sychev S.V. Metodika vybora optimal'nogo materinskogo vejvleta na osnove kriteriev energii i entropii.. Izvestiya Tul'skogo gosudarstvennogo universiteta. Tekhnicheskie nauki. 2017. № 7. S. 33–41 (in Russian).
- Liang X., Guo Z., Zhang Q., Yang M., Wang S. An analysis and decomposition ensemble prediction model for air passenger demand based on singular spectrum analysis. Xitong gongcheng lilun yu shijian. 2020.V. 40 №7. P. 1844–1855.
- Swart S.B., Den Otter A.R., Lamoth C.J.C. Singular spectrum analysis as a data-driven approach to the analysis of motor adaptation time series. Biomedical signal processing and control. 2022. V. 71. P.103.
- Golyandina N. Particularities and commonalities of singular spectrum analysis as a method of time series analysis and signal processing. Wiley interdisciplinary reviews: computational statistics. 2020. V. 12. № 4. P. e1487.
- Golyandina N., Zhigljavsky A. Singular spectrum analysis for time series. Springer, 2013.
- Singh Ju., Singla M. Image steganography technique based on singular value decomposition and discrete wavelet transform. International journal of electrical and electronics research. 2022. V.10. № 2. P. 122–125.
- Dou H., Wang G. Data denoising and compression of intelligent transportation system based on two-dimensional discrete wavelet transform. International journal of communication systems. 2021. P. 1–15.
- Grodzenskij S.Ya., Kalacheva E.A. Informacionnye tekhnologii: istoriya razvitiya i stanovleniya. Nelinejnyj mir. 2016. № 5. S. 74–79 (in Russian).
- Grodzenskij S.Ya., Chesalin A.N. Ispol'zovanie apparata nechetkoj logiki dlya ocenki nadezhnosti avtomatizirovannyh sistem. Nelinejnyj mir. 2017. T. 15. № 4. S. 17–24 (in Russian).
- Grodzenskij S.Ya., Emanakov I.V., Ovchinnikov S.A. Metodika vyyavleniya poter' v proizvodstvennoj sisteme. Naukoemkie tekhnologii. 2018. T. 19. № 1. S. 10–13 (in Russian).
- Grodzenskij S.Ya., Grodzenskij Ya.S, Mareshchenkov P.V. Statistiko-fizicheskij analiz nadezhnosti izdelij radioelektroniki na osnove smesi raspredelenij. Uspekhi sovremennoj radioelektroniki, 2021. T. 75. № 12. S. 63–68 (in Russian).