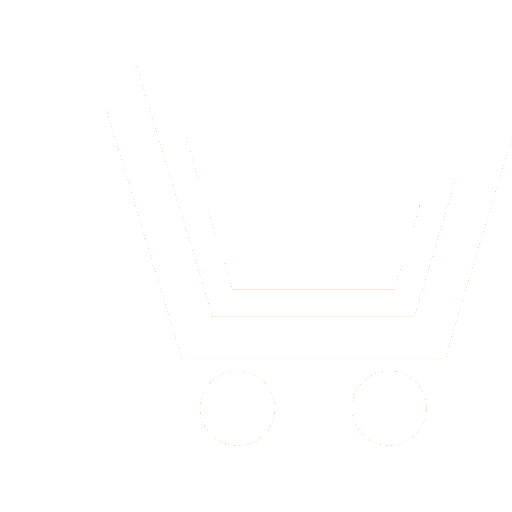
A.I. Ivanov1, I.N. Sinitsyn2
1 Penza State University (Penza, Russia)
2 FRC “Informatics and Computer Science” of the RAS (Moscow, Russia)
Nowadays bionic methods and means are widely applied in quality statistical analysis of complex information highly available systems at small samples. As it is known the classical statistical criteria for verification of normal (Gaussian) hypothesis give true solution with acceptable fiducial probability for large samples (200 and more). We consider the development of artificial binary neural networks multicriteria statistical analysis for small samples in case of triple neurons.
If we consider verification of normality hypothesis the usage of Pearson χ2-criterion for small samples in 16 experiments then we get true result with the fiducial probability 0.674. The results of computer experiments for responses χ2-criterion in case of normal data are presented on Fig.1. Let us consider the problem for several statistical criteria in the case of the small fixed sample. For example, it we use 20 classical statistical criteria then for every number k of experiments we get sufficiently different distributions of responses. For any combination of 20 classical criteria it is difficult to construct 20-dimentional table of fiducial probabilities. It is also difficult to unite k numbers of small samples experiment with the responses coupling correlation for various criteria.
The experience of parallel neural networks analysis for small samples was systemized in Handbook [8]. Here for linear random variables 21 software tools for classical statistical criteria and corresponding artificial binary neuron codes were presented. Special attention was paid to complex artificial neurons with multilevel output quantifications.
The main advantage of replacement of the known statistical criterion by equivalent artificial neurons is in simplification of parallel implementation. For example, if we construct 20 equivalent neurons for 20 known criteria then we have neural network with the output code having 20 multiple excessiveness. So technically complex problem of combination of 20 different tables for fiducial probabilities disappears. Implementation of 3 neural criteria reduces error probability till 12%. For fiducial probability 0.9 or error probability till 32,6% we need 8 or more criteria. For reaching fiducial probability 0.99 we need 100 criteria for parallel implementation. Relative simplicity of neural networks combination of statistical criteria no longer demands synthesis of “strong” and “very strong” criteria. “Strong” criterion may be replaced by several “weak” criteria. Nowdays we observe reinforced interest to combination of “weak” criteria by means of neural corresponding networks equivalents.
Applications and test examples are considered.
Ivanov A.I., Sinitsyn I.N. Development of neural networks statistical quality analysis of highly available systems for small samples. Highly Available Systems. 2023. V. 19. № 2. P. 38−45. DOI: https://doi.org/ 10.18127/j20729472-202302-03 (in Russian)
- Karpenko A.P., Sinicyn I.N. Bionika i sistemy vysokoj dostupnosti. Sistemy vysokoj dostupnosti. 2022. T. 18. № 2. S. 25–41. DOI: 10.18127/j20729472-202202-02 (in Russian).
- Kobzar' A.I. Prikladnaya matematicheskaya statistika. Dlya inzhenerov i nauchnyh rabotnikov. M.: FIZMATLIT, 2006 g., 816 s. (in Russian).
- Boll Rud, Konnel Dzh. H., Pankanti Sh., Ratha N. K., Sen'or E.U. i dr. Rukovodstvo po biometrii: Per. s angl. M.: Tekhnosfera, 2007. 368 s. (in Russian).
- Ivanov A.I. Biometricheskaya identifikaciya lichnosti po dinamike podsoznatel'nyh dvizhenij: Monografiya. Penza. Izd-vo Penzenskogo gos. un-ta. 2000. 178 s. (in Russian).
- Yazov Yu.K., Volchihin V.I., Ivanov A.I., Funtikov V.A., Nazarov I.G. Nejrosetevaya zashchita personal'nyh biometricheskih dannyh. Pod red. Yu.K. Yazova. M.: Radiotekhnika. 2012. 157 s. (in Russian).
- R 50.1.037-2002 Rekomendacii po standartizacii. Prikladnaya statistika. Pravila proverki soglasiya opytnogo raspredeleniya s teoreticheskim. Chast' I. Kriterii tipa χ2. M.: Gosstandart Rossii. 2001. 140 s. (in Russian).
- R 50.1.037-2002 Prikladnaya statistika. Pravila proverki soglasiya opytnogo raspredeleniya s teoreticheskim. Chast' II. Neparametricheskie kriterii. M.: Gosstandart Rossii. 2002. 123 s. (in Russian).
- Ivanov A.I. Nejrosetevoj mnogokriterial'nyj statisticheskij analiz malyh vyborok. Spravochnik. Penza: Izd-vo Penzenskogo gos. un-ta. 2022. 160 s. (in Russian).
- Ivanov A.P., Ivanov A.I., Malygin A.Yu., Bezyaev A.V., Kupriyanov E.N., Bannyh A.G., Perfilov K.A., Lukin V.S., Savinov K.N., Polkovnikova S.A., Serikova Yu.I. Al'bom iz devyati klassicheskih statisticheskih kriteriev dlya proverki gipotezy normal'nogo ili ravnomernogo raspredeleniya dannyh malyh vyborok. Nadezhnost' i kachestvo slozhnyh sistem. 2022. № 1. S. 20–29, DOI: 10.21685/2307-4205-2022-1-3 (in Russian).
- Pearson E. S. A further development of tests for normality. Biometrika. 1930. V. 22. P. 239–249.
- Geary R.C. The ratio of the mean deviation to the standard deviation as a test of normality. Biometrika. 1935. V. 27. P. 310–322.
- Ivanov A.I., Bannyh A.G., Serikova Yu.I. Uchet vliyaniya korrelyacionnyh svyazej cherez ih usrednenie po modulyu pri nejrosetevom obobshchenii statisticheskih kriteriev dlya malyh vyborok. Nadezhnost'. 2020. V. 20(2). P. 28–34. https://doi.org/10.21683/1729-2646-2020-20-2-28-34 (in Russian).
- Nikolls Dzhon, Martin Robert, Vallas Bryus, Fuks Pol. Ot nejrona k mozgu: Per. s angl. P.M. Balabana, A.V. Galkina, R.A. Giniatullina, R.N. Hazipova, L.S. Hiruga. M.: Editorial URSS. 2003. 672 s. (in Russian).
- Malygina E.A. Biometriko-nejrosetevaya autentifikaciya: perspektivy primeneniya setej kvadratichnyh nejronov s mnogourovnevym kvantovaniem biometricheskih dannyh: preprint. Penza: Izd-vo Penzenskogo gos. un-ta. 2020. 114 s. (in Russian).
- Sinicyn I.N., SHalamov A.S., Korepanov E.R., Belousov V.V., Sergeev I.V., Kuleshov A.A. Razvitie algoritmicheskogo i instrumental'nogo programmnogo obespecheniya dlya analiticheskogo veroyatnostnogo modelirovaniya i optimizacii processov material'no-tekhnicheskogo obespecheniya. Sbornik dokladov HIV Mezhdunar. nauch.-tekhn. konf. «Kibernetika i vysokie tekhnologii HKHI veka». 14–15 maya 2013 g. Voronezh: NPF SAKVOE. 2013. S. 375–384 (in Russian).
- Sinicyn I.N., SHalamov A.S., Korepanov E.R., Belousov V.V., Kuleshov A.A. Metody i sredstva ocenki zapasov i urovnya gotovnosti sistem integrirovannoj podderzhki, osnovannye na kanonicheskih razlozheniyah sluchajnyh funkcij. Materialy III Mezhdunar. nauch.-tekhn. seminara. 9–13 sentyabrya 29013 g., Sevastopol'. M.: IPIRAN, 2013. S. 115–126 (in Russian).
- Sinicyn I.N., SHalamov A.S. Lekcii po teorii sistem integrirovannoj logisticheskoj podderzhki. M.: TORUS PRESS. 2019. 1072 s. (in Russian).
- Sinicyn I.N. Kanonicheskie predstavleniya sluchajnyh funkcij. Teoriya i primeneniya. Izd. 2-e. M.: TORUS PRESS. 2023. 16 s. (in Russian).
- Jammalamadata K.S. Rao and Sen Gupta Topics in Circular Statistics World Scientific, Singapore. 2001.
- Sinicyn I.N. Matematicheskoe obespechenie dlya analiza nelinejnyh mnogokanal'nyh krugovyh stohasticheskih sistem, osnovannoe na parametrizacii raspredelenij. Informatika i ee primenenie. M.: TORUS PRESS. T. 6. Vyp. 1. S. 12–18 (in Russian).