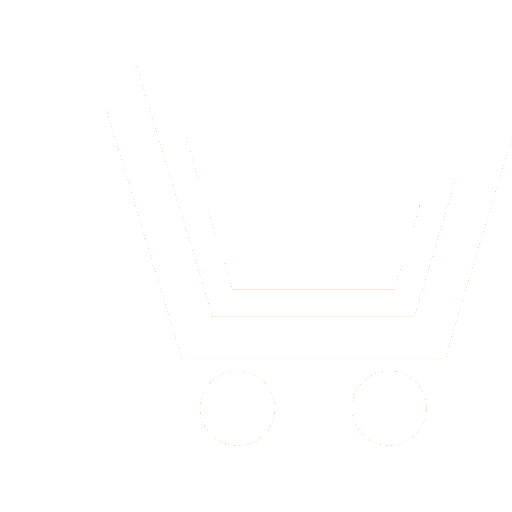
O.V. Vasiliev1, E.A. Bolelov2, K.I. Galaeva3, E.S. Boyarenko4
1–4 Moscow State Technical University of Civil Aviation (Moscow, Russia)
1 vas_ov@mail.ru, 2 edbolelov@mail.ru, 3 ks.galaeva@mail.ru, 4 boyarenko.elvira@mail.ru
Cumulonimbus clouds and accompanying meteorological phenomena such as rainfalls, thunderstorms, hail are dangerous weather phenomena for aviation. These phenomena pose the greatest danger during takeoff and landing operations of aircraft. The existing classification of such dangerous meteorological phenomena in weather radars is based on data on the altitude distribution of the radar reflectivity of the atmosphere, air temperature and has its drawbacks. It is characteristic that all legislatively accepted criteria for classifying meteorological phenomena from clouds to tornadoes, naturally based on long-term observations and in this sense inspiring respect, are developed for each phenomenon separately and are of a somewhat heuristic nature. Purpose: to develop a method for classifying dangerous meteorological phenomena of cumulonimbus clouds in weather radars, which will increase the reliability of the classified meteorological phenomena. The paper shows that significant shortcomings of the criteria used to classify dangerous meteorological phenomena in weather radars include: the absence of information on atmospheric turbulence in the classification features and the use of its own criterion for each phenomenon. It is obvious that the criteria for classifying hazardous meteorological phenomena should be optimized in the following areas: the use of information on the altitude distribution of maximum values of not only reflectivity, but also atmospheric turbulence in the classification criteria; the classification should be based on a single selected criterion for distinguishing statistical hypotheses, which is implemented in the article. To solve the problem of classifying hazardous meteorological phenomena of cumulonimbus clouds, the Bayesian approach is substantiated under conditions of complete a priori uncertainty. A classification method is proposed based on the maximum likelihood criterion using sufficient statistics of reflectivity and atmospheric turbulence. Based on the analysis of the information content of classification features for one-dimensional and multidimensional densities, it was concluded that the required reliability of classification is achieved with the simultaneous use of all four features: maximum values of reflectivity and atmospheric turbulence, as well as their distribution by height. For a specific statement of the problem, an algorithm for classifying dangerous meteorological phenomena of cumulonimbus clouds was developed. The selected criterion for distinguishing statistical hypotheses and the introduction of information on atmospheric turbulence into the criteria for classifying dangerous meteorological phenomena associated with cumulonimbus clouds will improve the reliability of the classified meteorological phenomena in weather radars.
Vasiliev O.V., Bolelov E.A., Galaeva K.I., Boyarenko E.S. Bayesian method of radar classification of dangerous meteorological phenomena of cumulonimbus clouds. Electromagnetic waves and electronic systems. 2025. V. 30. № 1. P. 55−67. DOI: https://doi.org/10.18127/j15604128-202501-06 (in Russian)
- Basov I.A., Dmitrieva O.A., Dorofeev E.V. Methodical instructions for the production of meteorological radar observations on DMRL-S on the Roshydromet network for the purposes of storm warning and meteorological support for aviation. St. Petersburg: FGBU "GGO". 2013. 137 p. (In Russian)
- Guidelines for the use of information from the Doppler meteorological radar DMRL-S in synoptic practice. M.: Ministry of Natural Resources and Ecology of the Russian Federation. 2019. 129 p. (In Russian)
- Zhukov V.Yu., Shchukin G.G. Status and prospects of the network of Doppler meteorological radars. Meteorology and hydrology. 2014. № 2. P. 92–100. (In Russian)
- Dyaduchenko V.N., Vylegzhanin I.S., Pavlyukov Yu.B. Doppler radars in Russia. Science in Russia. 2014. № 1. P. 23–27. (In Russian)
- Lekhovitsky D.I., Rachkov D.S., Semenyaka A.V., Atamansky D.V., Pushkov A.A. Estimation of doppler velocities spectrum width of meteorological formations in coherent pulsed weather radars with arbitrary intervals of sounding. Achievements of modern radioelectronics. 2012. № 8. P. 47–68. (In Russian)
- Batalov K.A., Kulakov M.V., Chekhov I.A. Research of the cooperation technology between the air traffic control service and the aerodrome service. Civil aviation high technologies. 2021. V. 24. № 4. P.8–19. (In Russian)
- Weather radar-Part 1: System performance and operation. World Meteorological Organization. 2018. 97 p.
- Mazurov G.I., Akselevich V.I. Use of information obtained with the help of DMRL-s in meteorology. Abstracts of reports of the I Russian scientific conference «Radiophysics, photonics and study of the properties of matter». Omsk: Omsk Research Institute of Instrument Engineering. 2020. P. 83–84. (In Russian)
- Vasiliev O.V., Bolelov E.A., Gevak N.V., Zyabkin S.A., Galaeva K.I., Kolesnikov E.S., Peshko A.S., Sinitsyn I.A. The Design and Operation Features of the Near-airfield Zone Weather Radar Complex 'Monocle'. 18th Technical Scientific Conference on Aviation Dedicated to the Memory of N.E. Zhukovsky. Moscow. 2021. P. 64–72. DOI 10.1109/TSCZh53346.2021.9628352.
- Kumjian R.M. Weather Radars. Remote Sensing of Clouds and Precipitation. Springer International Publishing. 2018. P. 15–63. DOI 10.1007/978-3-319-72583-3_2.
- Kononova N.K., Lupo A.R. An Investigation of circulation regime variability and dangerous weather phenomena in Russia in the 21stcentury. IOP Conference Series: Earth and Environmental Science: Causes, Risks, Consequences, Problems of Adaptation and Management. Moscow. 2020. P. 012023. DOI 10.1088/1755-1315/606/1/012023.
- Nanding N., Rico-Ramirez M.A. Precipitation Measurement with Weather Radars. ICT for Smart Water Systems: Measurements and Data Science/Springer Nature. 2019. V. 102. P. 235–258. DOI 10.1007/698_2019_404.
- Vasiliev O.V., Korotkov S.S., Galaeva K.I., Boyarenko E.S. Decision criteria for the classification of meteorological phenomena in the weather radar complex of the near-airfield zone. Civil Aviation High Technologies. 2023. V. 26. № 2. P. 49–60. DOI 10.26467/2079-0619-2023-26-2-49-60. (In Russian)
- Boyarenko E.S., Bolelov E.A., Vasiliev O.V., Korotkov S.S. Experimental statistical analysis of radar signals reflected from weather hazards. Civil Aviation High Technologies. 2023. V. 26. № 5. P.19–29. DOI 10.26467/2079-0619-2023-26-5-19-29. (In Russian)
- Vasiliev O.V., Boyarenko E.S., Galaeva K.I. Substantiation of source data on the parametric algorithms for the classification of weather hazards. Civil Aviation High Technologies. 2023. V. 26. № 6. P. 8–21. DOI 10.26467/2079-0619-2023-26-6-8-21. (In Russian)
- Guidelines for the production of observations and the use of information from non-automated radars MRL-1, MRL-2, MRL-5. RD 52.04.320-91. St. Petersburg.1993. 342 p. (In Russian)
- Duda R., Hart P. Pattern Recognition and Scene Analysis. Moscow: Mir. 1976. 507 p. (In Russian)
- Gorelik A.L., Skripkin V.A. Recognition methods. Moscow: Higher school. 1989. 231 p. (In Russian)
- Рepin V.G., Tartakovsky G.P. Statistical synthesis under a priori uncertainty and adaptation of information systems. Moscow: Soviet radio. 1977. 432 p. (In Russian)
- Fomin Ya.A., Tarlovsky G.R. Statistical theory of pattern recognition. Moscow: Radio and Communications. 1986. 263 p. (In Russian)
- Tikhonov V.I., Bakaev Yu.N. Statistical theory of radio engineering devices. Moscow: Publishing house of VVIA named after prof. N.E. Zhukovsky. 1978. 420 p. (In Russian)
- Bekryaev V.I. Fundamentals of the Theory of Experiment. Study Guide. St. Petersburg: Publishing House of the Russian State Medical University. 2001. 266 p. (In Russian)
- Degtyarev A.S., Drabenko V.A., Drabenko V.A. Statistical methods of processing meteorological information. Textbook. St. Petersburg: Andreevsky Publishing House. 2015. 225 p. (In Russian)
- Approximation based on typical distributions. [Electronic resource] – Access mode: https://poznayka.org/s97706t1.html, date of reference 12.02.2024. (In Russian)
- Kremer N.Sh. Probability Theory and Mathematical Statistics: Textbook. 2nd edition. Moscow: UNITY-DANA. 2004. 573 p. (In Russian)
- Ayvazyan S.A., Enyukov I.S., Meshalkin L.D. Applied statistics: Fundamentals of modeling and primary data processing. Reference publication. Moscow: Finance and statistics. 1983. 471 p. (In Russian)