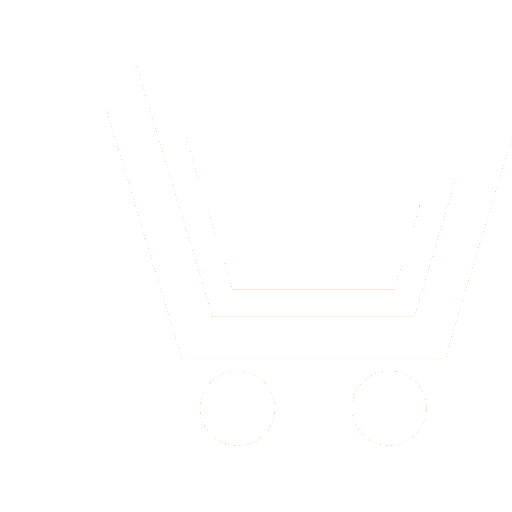
A.O. Schiriy1
1 Pushkov Institute of Terrestrial Magnetism, Ionosphere and Radio Wave Propagation of the Russian Academy of Sciences (Moscow, Troitsk, Russia)
1 andreyschiriy@gmail.com
According to ionospheric radiosonding data, short-wave signals can provide information about the processes in ionospheric plasma, its structure and condition; these data are also extremely important for radio engineering systems operating in the short-wave range. The most important problem in the automatic processing of ionograms (results of ionospheric radiosonding) is the allocation of tracks of various modes of the radio signal. The existing methods of track selection do not provide an acceptable quality of selection (especially for inclined sensing ionograms). Therefore, it was necessary to use a semi-automatic method of isolating the signal on the ionogram. The paper proposes a method of using neural networks to isolate tracks of radio signal modes on ionograms, taking into account the specifics of ionograms: when constructing a training sample of labeled ionograms, ionospheric radiosonde ionograms are selected for normal conditions of radio wave propagation in the ionosphere (absence of solar and geomagnetic disturbances) and when marking them, they are marked with a binary sign "non-abnormal", additionally selected and ionograms for abnormal propagation conditions are added to the sample and marked with the binary sign "abnormal" at the marking stage, and at the stage of model training, not only the bit masks of the selected tracks are used as features, but also the metadata of the ionograms. To fully test and evaluate the effectiveness of the method, it is necessary to build large samples of labeled ionograms. The requirement for large volumes of training samples is caused by a large number of internal parameters of deep learning models (and there must be much more objects for training than the number of model parameters, otherwise the model will simply "retrain" for specific data). For more effective marking, we are developing specialized software for highlighting various objects on ionograms. The difference between such a specialized marking tool from existing image marking programs is that means must be implemented not only to highlight the boundaries of the radio signal mode tracks, but also the possibility of marking all the information that a person "sees" on an ionogram: upper and lower rays, magnetoion rays, areas of increased diffusivity, concentrated (station) interference, background interference. and others . Tracks can be accompanied by additional marking attributes: upper beam, magneto-ionic splitting, diffusivity region, equipment failure. In addition to signal tracks, noise objects with appropriate markings can also be highlighted: station noise and background noise; moreover, for station interference, another way of highlighting is implemented – with whole vertical lines. Also, the further development of the markup tool described above will consist in adding the functionality of marking traces of ionospheric inhomogeneities, as well as cross-monitoring the results of third-party markups. To fully test and evaluate the effectiveness of the method, it is necessary to build large samples of labeled ionograms, which will be the focus of further work.
Schiriy A.O. Neural networks usage for upgrade of the software part of equipment for radio sounding of the ionosphere. Electromagnetic waves and electronic systems. 2024. V. 29. № 5. P. 55−60. DOI: https://doi.org/10.18127/j15604128-202405-08 (in Russian)
- Fabrizio G.A. High frequency over-the-horizon radar: fundamental principles, signal processing, and practical applications. McGraw-Hill Education. 2013.
- Shhiry`j A.O. Razrabotka i modelirovanie algoritmov avtomaticheskogo izmereniya xarakteristik ionosferny`x korotkovolnovy`x radiolinij: Avtoref. dis. … kand. texn. nauk. SPb., 2007. 19 c.
- Shhiry`j A.O. Razvitie sredstv avtomatizacii nazemnogo radiozondirovaniya ionosfery`. Fundamental`ny`e problemy` ra-dioe`lektronnogo priborostroeniya. 2014. T. 14. № 5. S. 170–173.
- Shhiry`j A.O. Arxitektura programmnoj chasti apparatno-programmnogo kompleksa distancionnogo nazemnogo radiozondirovaniya ionosfery`. Novy`e informacionny`e texnologii v avtomatizirovanny`x sistemax. 2015. № 18. S. 144–152.
- Shhiry`j A.O. Algoritmy` i programmnoe obespechenie avtomatizacii processov izmerenij i obrabotki danny`x operativnoj diagnostiki ionosfery` i ionosferny`x radiolinij. Zhurnal radioe`lektroniki. 2022. № 10. https://doi.org/10.30898/1684-1719.2022.10.4
- Filipp N.D., Blaunshtejn N.Sh., Eruximov L.M., Ivanov V.A., Uryadov V.P. Sovremenny`e metody` issledovaniya dinamicheskix processov v ionosfere. Kishinev: Shtiincza. 1991. 286 s.
- Ponomarchuk S.N., Grozov V.P., Kotovich G.V., Mixajlov S.Ya. Obrabotka i interpretaciya ionogramm vertikal`nogo i naklonnogo zondirovaniya dlya diagnostiki ionosfery` na baze LChM-ionozonda. Vestnik Sibirskogo gosudarstvennogo ae`rokosmicheskogo universiteta im. akad. M.F. Reshetneva. 2013. № 5(51). S. 163–166.
- Patent RF №2697433. Cybulya K.G. Sposob avtomaticheskogo opredeleniya parametrov ionosferny`x sloev po ionogrammam. Data zayavki: 26.10.2018. Data publikacii: 14.08.2019.
- Egoshin A.B. Avtomatizirovannaya sistema adaptivnoj obrabotki signalov so sverxbol`shoj bazoj dlya radiozondirovaniya ionosferny`x radiolinij. Avtoref. dis. … kand. texn. nauk. Marijskij gos. texn. un-t. Joshkar-Ola, 2003. 25 c.
- Kolchev A.A., Shhiry`j A.O. Algoritm avtomaticheskogo vy`deleniya spektral`ny`x komponentov signala na ionogramme / Mater. desyatogo nauchno-prakt. seminara «Novy`e informacionny`e texnologii». M.: Moskovskij gos. in-t e`lektroniki i matematiki. 2007. S. 102–107.
- Nedopekin A.E. Metod obnaruzheniya signala LChM-ionozonda v chastotnoj oblasti s uchetom ushireniya prinimaemy`x mod ionosfernogo rasprostraneniya. Zhurnal Radioe`lektroniki: e`lektronny`j zhurnal. 2015. № 10. URL: http://jre.cplire.ru/jre/ oct15/5/text.pdf
- Dolgacheva S.A., Makarova L.N., Nikolaev A.V. Obrabotka ionogramm vy`sokoshirotny`x stancij vertikal`nogo zondirovaniya s ispol`zovaniem nejronny`x setej: Es i F2 sloi. Physics of Auroral Phenomena. 2020. T. 43. № 1. S. 105–108.
- Guo L., Xiong J. Multi-Scale Attention-Enhanced Deep Learning Model for Ionogram Automatic Scaling. Radio Science. 2023. V. 58. № 3. p.e2022RS007566. https://doi.org/10.1029/2022RS007566
- Lu Z., Hua C., Wei N., Feng J., Lou P., Liu W. Research on classification of vertical ionogram based on deep convolution neural network // Progress in Geophysics. 2022. V. 37. № 5. P. 1834–1839. doi: 10.6038/pg2022GG0073
- Xiao Z., Wang J., Li J., Zhao B., Hu L., Liu L. Deep-learning for ionogram automatic scaling. Advances in Space Research. 2020. V. 66. № 4. P. 942–950. https://doi.org/10.1016/j.asr.2020.05.009
- De la Jara C., Olivares C. Ionospheric echo detection in digital ionograms using convolutional neural networks. Radio Science. 2021. V. 56. № 8. P. 1–15.
- Zayavka № 2023135724 na izobretenie. Sposob avtomaticheskogo vy`deleniya poleznogo signala na ionogramme radiozondiro-vaniya ionosfery`, osnovanny`j na mashinnom obuchenii (varianty`) / A.O. Shhiry`j; Institut zemnogo magnetizma, ionosfery` i rasprostraneniya radiovoln RAN im. N.V. Pushkova, Moskva; Zayavl. 26.12.2023.