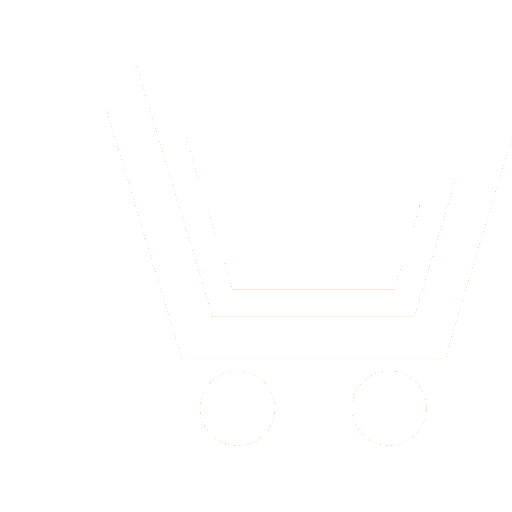
I.M. Lerner1, A.Kh. Marinosyan2, S.G. Grigoriev3, A.R. Yusupov4, M.A. Anikyeva5, G.A. Garifullina6
1 Kazan National Research Technical University named after A.N. Tupolev – KAI (Kazan, Russia)
2,3 Moscow City Pedagogical University (Moscow, Russia)
4 Bashkir State Pedagogical University named after M. Akmully (Ufa, Russia)
5 Siberian Federal University (Krasnoyarsk, Russia)
6 Kazan National Research Technological University (Kazan, Russia)
1 aviap@mail.ru, 2 marinos.andrey@yandex.ru, 3 grigorsg@yandex.ru, 4 azat.yusupov@bk.ru, 5 MAnikieva@sfu-kras.ru, 6 gulnarakdrv03@mail.ru
Today, in the era of transition to the information society, the issue of analysis and processing of scientific information is extremely acute. This is due to the fact that the use of results and processing directly and indirectly affect the country's strategy in the field of educational activities. Direct influence is manifested in the introduction of the results of scientific activity into the educational process in the form of educational material. At the same time, indirect influence involves a more complex mechanism, which was described by the authors earlier and has a more long-term effect due to the implementation of the effects of generational values that are formed at the age of 12–14 years under the influence of existing technological development, family values and the socio-cultural environment, which in turn forms patterns of behavior that affect the process of choosing and studying new information, developing new technical solutions, making key decisions, which directly influence the industrial potential of the country. Currently, there is a fairly large number of scientometric methods for studying scientific information, which, among other things, allow implementing in a limited form the idea of D. Price about the "invisible college", when in the course of analyzing scientific information it is possible to determine the scientific social structure consisting of universities, research institutes, scientific journals, conferences, scientists in individual fields of science. However, all methods use in one form or another formal analysis and a context-free approach to assessing citations, which does not allow for a qualitative assessment of the processing and transformation of information in the process of scientific activity, which is a necessary condition for the development of the country's industry.
Creating an algorithm for classifying scientific information by generating prompts for a large language model to ensure contextual analysis of citations in scientific papers and classifying scientific information based on deep semantic analysis.
Requirements have been formed for the selection of scientific information that provides the highest quality analysis results from the point of view of expert opinion. The presented algorithm for generating queries to a large language model facilities contextual analysis and classification of bibliographic references in scientific information. The proposed approach for clustering scientific information takes into account the multidisciplinary nature of research and ensures the continuity of research based on multidimensional bases. It is shown that the quality of contextual analysis of bibliographic references due to the developed algorithm has increased by 27% compared to using a large language model without this algorithm. Based on experimental studies, the possibility of predicting changes in the social sphere is shown.
The algorithm for generating queries to a large language model is presented, facilities for contextual analysis and classification of bibliographic references in scientific information. The proposed approach for clustering scientific information takes into account the multidisciplinary nature of research and ensures the continuity of research based on linguistic multidimensional bases.
Lerner I.M., Marinosyan A.Kh., Grigoriev S.G., Yusupov A.R., Anikyeva M.A., Garifullina G.A. An approach to the formation of intellectual academic genealogy using large language models. Electromagnetic waves and electronic systems. 2024. V. 29. № 4. P. 108−120. DOI: https://doi.org/10.18127/j15604128-202404-09 (in Russian)
- Shraiberg Ya.L. Information market, educational and library environment in the modern digital environment: new trends and expected results. The Eighth International professional forum "Book. Culture. Education. Innovations" Moscow: State Public Scientific and Technical Library of Russia. 2024. 48 p. DOI 10.33186/978-5-85638-274-6-2024. (in Russian)
- Lerner I.M., Karelina E.A., Grigoriev S.G., Baykov F.Yu., Dymkova S.S., Ilyin V.I. A model for selecting information resources based on the theory of generations, scientometry and factorial methods of personality research as a tool for the development of global digital platforms. Scientific and technical libraries. 2024. № 1. P. 15–50. (in Russian)
- Lerner I.M., Baykov F.Yu., Karelina E.A., Grigoriev S.G., Sychev A.S., Dymkova S.S. Building typical profiles of students of generation Z to improve the quality of the educational process. Informatics and education. 2023. V. 38. № 6. P. 5–13. (in Russian)
- Price D.J. Little science, big science and beyond. NY: Columbia University Press. 1986. 336 p.
- Garfield E. Citation analysis as a tool in journal evaluation. Science. 1972. V. 178. № 4060. P. 471–479. DOI 10.1126/science.178. 4060.471.
- Garfield E. Citation indexing: Its theory and application in science, technology, and humanities. NY: John Wiley & Sons. 1979. 274 p.
- Rousseau R., Zhang L. Betweenness centrality and Q-measures in directed valued networks. Scientometrics. 2008. V. 75. № 3. P. 575–590. DOI 10.1007/s11192-007-1772-2.
- Roth C., Wu J., Lozano S. Assessing impact and quality from local dynamics of citation networks. Journal of Informetrics. 2012. V. 6. № 1. P. 111–120. DOI 10.1016/j.joi.2011.08.005.
- Small H. Co-citation in the scientific literature: A new measure of the relationship between two documents. Journal of the American Society for Information Science. 1973. V. 24. № 4. P. 265–269. DOI 10.1002/asi.4630240406.
- Leydesdorff L. The Evolutionary Dynamics of Discursive Knowledge. Qualitative and Quantitative Analysis of Scientific and Scholarly Communication. Cham: Springer. 2021. 248 p.
- Daraio C., Di Leo S., Leydesdorff L. A heuristic approach based on Leiden rankings to identify outliers: evidence from Italian universities in the European landscape. Scientometrics. 2023. V. 128. № 1. P. 483–510. DOI 10.1007/s11192-022-04551-y.
- Leydesdorff L., Bornmann L. Disruption indices and their calculation using web-of-science data: Indicators of historical developments or evolutionary dynamics? Journal of Informetrics. 2021. V. 15. № 4. P. 101219. DOI 10.1016/j.joi.2021.101219.
- Funk R.J., Owen-Smith J. A dynamic network measure of technological change. Management Science. 2017. V. 63. № 3. P. 791–817. DOI 10.1287/mnsc.2015.2366.
- Wu S., Wu Q. A confusing definition of disruption. [Electronic resource] – Access mode: https://osf.io/preprints/socarxiv/d3wpk/, date of reference 21.06.2024.
- Zhang L., Leydesdorff L. The scientometric measurement of interdisciplinarity and diversity in the research portfolios of Chinese Universities. Journal of Data and Information Science. 2021. V. 6. № 4. P. 13–35. DOI 10.2478/jdis-2021-0027.
- Dymkova S.S. Methods and procedural model of increasing the publication activity of scientific organizations based on scientometric tools: dis. ... candidate of Technical Sciences. M. 2022. 170 p. (in Russian)
- David S.V., Hayden B.Y. Neurotree: A collaborative, graphical database of the academic genealogy of neuroscience. PloS One. 2012. V. 7. № 10. P. e46608. DOI 10.1371/journal.pone.0046608.
- Madeira G., Borges E.N., Lucca G., Santos H., Dimuro G. A tool for analyzing academic genealogy. Enterprise Information Systems. 2019. P. 443–456. DOI 10.1007/978-3-030-40783-4_21.
- Rossi L., Damaceno R.J., Mena-Chalco J.P., Freire I.L. Topological metrics in academic genealogy graphs. Journal of Informetrics. 2018. V. 12. № 4. P. 1042–1058. DOI 10.1016/j.joi.2018.08.004.
- Hirshman B.R., Tang J.A., Jones L.A., Proudfoot J.A., Carley K.M., Marshall L., Carter B.S., Chen C.C. Impact of medical academic genealogy on publication patterns: An analysis of the literature for surgical resection in brain tumor patients. Annals of Neurology. 2016. V.79. № 2. P. 169–177. DOI 10.1002/ana.24569.
- Shraiberg Ya.L. Special components of society digital transformation to influence technological and behavioral models of modern libraries. Scientific and technical libraries. 2023. № 8. P. 13–84. DOI 10.33186/1027-3689-2023-8-13-84. (in Russian)
- Borsuk N.A., Deryugina E.O., Hartman V.A. Development of the specialized library system. Electromagnetic waves and electronic systems. 2019. V. 24. № 3. P. 45–54. DOI 10.18127/j15604128-201903-08. (in Russian)
- Borsuk N.A., Deryugina E.O., Hartman V.A. Automation of book delivery process in specialized library systems. Electromagnetic waves and electronic systems. 2019. V. 24. № 7. P. 30–37. DOI 10.18127//j15604128-201907-05. (in Russian)
- Masyukov K.P., Konovalov D.Yu., Kulikov S.V. Features of forming the algorithm of the information processing system based on empirical data. Electromagnetic waves and electronic systems. 2020. V. 25. № 3. P. 57−64. DOI 10.18127/j15604128-202003-06 (in Russian).
- Smirnov I.V. Methods of multilevel analysis of texts in natural language and their applications in information retrieval systems and psycholinguistic research: dis. ... doctor of Technical Sciences. M. 2023. 335 p. (in Russian)
- Navarro G. A guided tour to approximate string matching. ACM Computing Surveys. 2001. V. 33. № 1. P. 31–88. DOI 10.1145/375360. 375365.
- Wentzel E.S. Theory of probability. M.: Nauka. 1969. 575 p. (in Russian)