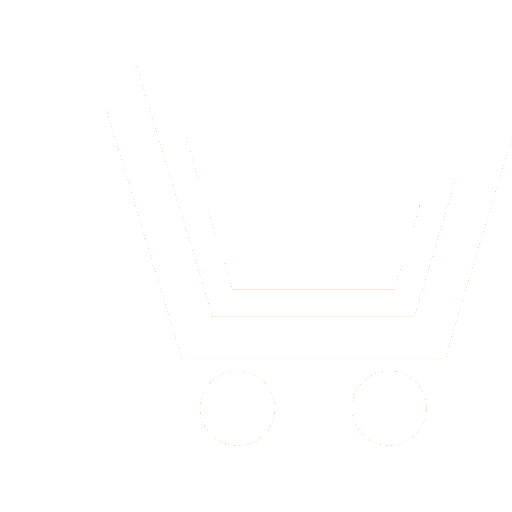
A.K. Gaysin1, A.A. Korobkov2, I.A. Safiullin3, I.P. Ashaev4, A.F. Nadeev5
1–5 Kazan National Research Technical University named after A.N. Tupolev – KAI (Kazan, Russia)
1 akgaysin@kai.ru, 2 aakorobkov@kai.ru, 3 iasafiullin@kai.ru, 4 ipashaev@kai.ru, 5 afnadeev@kai.ru
The paper presents the results of a study on the influence of user mobility patterns in the O-RAN (Open Radio Access Net-work) on the parameters of a multi-linear model. The considered multi-linear model is represented as three-dimensional tensors that describe the changes of received signal power or SINR (Signal-to-Interference-plus-Noise Ratio). Factor matrices after the CP (Canonycal Polyadic) decomposition are exploited to separate users by their mobility using the DBSCAN (Density-Based Spatial Clustering of Applications with Noise) algorithm. A model and a set of scenarios are developed for simulating the O-RAN segments and to study the influence of the user mobility on the parameters of the multi-linear model. Simulation results indicate that the number of users in groups with different mobility patterns does not affect the parameters of the multi-linear model and the performance of the DBSCAN algorithm. For the considered scenarios, the best classification results are achieved when the order of the multi-linear model equal to three. Moreover, it is revealed that the topology of a network segment effects on the order of a multi-linear model.
- Shafi M., Molisch A.F., Smith P.J., Haustein T., Zhu P., De Silva P., Tufvesson F., Benjebbour A., Wunder G. 5G: A tutorial overview of standards, trials, challenges, deployment, and practice. IEEE Journal on Selected Areas in Communications. 2017. V. 35. № 6. P. 1201–1221. DOI 10.1109/JSAC.2017.2692307.
- Agiwal M., Roy A., Saxena N. Next Generation 5G Wireless Networks: A Comprehensive Survey. IEEE Communications Surveys & Tutorials. 2016. V. 18. № 3. P. 1617–1655. DOI 10.1109/COMST.2016.2532458.
- Lerner I.М., Fayzullin R.R., Khairullin А.N., Shushpanov D.V., Il’in V.I., Ryabov I.V. Specify capacity increasing as a fundamental problem of communication theory. Strategy development in the post-Shannon era. Part 1. Retrospective review of methods for receiving and processing signals in frequency-selective communication channels at data transfer rates faster than Nyquist rate. Achievements of Modern Radioelectronics. 2023. V. 77. № 1. P. 37–50. DOI 10.18127/j20700784-202301-02. (in Russian)
- Lerner I.М., Fayzullin R.R., Khairullin А.N., Shushpanov D.V., Il’in V.I., Ryabov I.V. Specify capacity increasing as a fundamental problem of communication theory. Strategy development in the post-Shannon era. Part 2. Retrospective review of methods for receiving and processing signals in frequency-selective communication channels in the presence of ISI. Achievements of Modern Radioelectronics. 2023. V. 77. № 2. P. 16–33. DOI 10.18127/j20700784-202302-02. (in Russian)
- Lerner I.М., Fayzullin R.R., Shushpanov D.V., Il’in V.I., Ryabov I.V., Khairullin А.N. Specify capacity increasing as a fundamental problem of communication theory. Strategy development in the post-Shannon era. Part 3. Retrospective review of methods for capacity estimating of frequency-selective communication channels in the presence of ISI and using PSK-n and APSK-N-signal. Achievements of Modern Radioelectronics. 2023. V. 77. № 3. P. 24–33. DOI 10.18127/j20700784-202303-02. (in Russian)
- Lerner I.M., Fayzullin R.R., Ryabov I.V. High performance algorithm for capacity estimation of communication channels functioning on the basis of resolution time theory. Radiotekhnika. 2022. V. 86. № 4. P. 91–109. DOI 10.18127/j00338486-202204-13. (in Russian)
- Lerner I.M., Khairullin A.M. Resolution time theory in the topic of broadband communications. Algorithm for data dependent jitter and capacity estimations with polynomial time execution. T-Comm. 2023. V. 17. № 5. P. 48–57. DOI 10.36724/2072-8735-2023-17-5-48-57.
- Khairullin A.N., Lerner I.M., Ayupov T.A. Algorithm for capacity estimation based on time resolution theory with linear computational complexity for frequency-selective communication channels and PAM-n-signals. Radiotekhnika. 2024. V. 88. № 1. P. 31−43. DOI 10.18127/j00338486-202401-04. (In Russian)
- O-RAN Use Cases and Deployment Scenarios. [Electronic resource] – Access mode: https://mediastorage.o-ran.org/white-papers/O-RAN.WG1.Use-Cases-and-Deployment-Scenarios-White-Paper-2020-02.pdf, date of reference 18.06.2024.
- Singh S.K., Singh R., Kumbhani B. The Evolution of Radio Access Network Towards Open-RAN: Challenges and Opportunities. IEEE Wireless Communications and Networking Conference Workshops. 2020. P. 1–6. DOI 10.1109/WCNCW48565.2020.9124820.
- Zaitsev I.V., Molev A.A. Algorithm for generating the structure of a self-organizing radio communication system based on the hierarchy of the control system. Electromagnetic waves and electronic systems. 2021. V. 26. № 6. P. 57−70. DOI 10.18127/ j15604128-202106-06. (in Russian)
- Lvovich Ya.E., Preobrazhensky A.P., Preobrazhensky Yu.P., Choporov O.N. Optimization of characteristics of distributed telecommunication systems. Electromagnetic waves and electronic systems. 2022. V. 27. № 1. P. 39−46. DOI: 10.18127/j15604128-202201-05. (in Russian)
- Balasubramanian B., Daniels E.S., Hiltunen M., Jana R., Joshi K., Sivaraj R., Tran T.X., Wang C. RIC: A RAN Intelligent Controller Platform for AI-Enabled Cellular Networks. IEEE Internet Computing. 2021. V. 25. № 2. P. 7–17. DOI 10.1109/MIC.2021.3062487.
- Taleb T., Benzaïd Ch., Addad R.A., Samdanis K. AI/ML for beyond 5G systems: Concepts, technology enablers & solutions. Computer Networks. 2023. V. 237. P. 110044. DOI 10.1016/j.comnet.2023.110044.
- Boukhedouma H., Meziane A., Hammoudi S., Benna A. On the challenges of mobility prediction in smart cities. The International Archives of the Photogrammetry, Remote Sensing and Spatial Information Sciences. 2020. V. 44. P. 17–24. DOI 10.5194/ISPRS-ARCHIVES-XLIV-4-W2-2020-17-2020.
- Irio L., Ip A., Oliveira R., Luís M. An Adaptive Learning-Based Approach for Vehicle Mobility Prediction. IEEE Access. 2021. V. 9. P. 13671–13682. DOI 10.1109/ACCESS.2021.3052071.
- Ip A., Irio L., Oliveira R. Vehicle Trajectory Prediction based on LSTM Recurrent Neural Networks. IEEE 93rd Vehicular Technology Conference. 2021. P. 1–5. DOI 10.1109/VTC2021-Spring51267.2021.9449038.
- Solomon A., Livne A., Katz G., Shapira B., Rokach L. Analyzing movement predictability using human attributes and behavioral patterns. Computers, Environment and Urban Systems. 2021. V. 87. P. 101596. DOI 10.1016/J.COMPENVURBSYS.2021.101596.
- Miao Q., Li M., Lin W., Wang Zh., Shao H., Xie Ju., Shu N., Qiao Yu. Human Mobility Prediction with Calibration for Noisy Trajectories. Electronics. 2022. V. 11. № 20. P. 3362. DOI 10.3390/electronics11203362.
- Araújo F., Bastos L., Medeiros Ia., Rosso O.A., Aquino A.L.L., Rosário D., Cerqueira E. Characterization of human mobility based on Information Theory quantifiers. Physica A: Statistical Mechanics and its Applications. 2023. V. 609. P. 128344. DOI 10.1016/j.physa. 2022.128344.
- Jeong J., Roeland D., Derehag J., Johansson А.A., Umaashankar V., Sun G., Eriksson G. Mobility Prediction for 5G Core Networks. IEEE Communications Standards Magazine. 2021. V. 5. № 1. P. 56–61. DOI 10.1109/MCOMSTD.001.2000046.
- Mollel M.S., Abubakar A.I., Ozturk M., Kaijage S., Kisangiri M., Zoha A., Imran M.A., Abbasi Q.H. Intelligent handover decision scheme using double deep reinforcement learning. Physical Communication. 2020. V. 42. P. 101133. DOI 10.1016/J.PHYCOM. 2020.101133.
- Liu Q., Chuai G., Wang J., Pan J. Proactive Mobility Management with Trajectory Prediction Based on Virtual Cells in Ultra-Dense Networks. IEEE Transactions on Vehicular Technology. 2020. V. 69. № 8. P. 8832–8842. DOI 10.1109/TVT.2020.2995318.
- Ashaev I.P., Safiullin I.A., Gaysin A.K., Nadeev A.F., Korobkov A.A. An Approach for Using a Tensor-Based Method for Mobility-User Pattern Determining. Inventions. 2024. V. 9. № 1. P. 1. DOI 10.3390/inventions9010001.
- Korobkov A.A., Safiullin I.A., Ashaev I.P., Gaysin A.K., Nadeev A.F. Model order estimation method for user mobility classification in heterogeneous RAN systems. Radiotekhnika. 2024. V. 88. № 1. P. 44−58. DOI 10.18127/j00338486-202401-05. (In Russian)
- Korobkov A.A., Diugurova M.K., Haueisen J., Haardt M. Robust Multi-Dimensional Model Order Estimation Using LineAr Regression of Global Eigenvalues (LaRGE). IEEE Transactions on Signal Processing. 2022. V. 70. P. 5751–5764. DOI 10.1109/tsp.2022.3222737.
- Ester M., Kriegel H., Sander J., Xu X. A Density-Based Algorithm for Discovering Clusters in Large Spatial Databases with Noise. Knowledge Discovery and Data Mining. Proceedings of the Second International Conference on Knowledge Discovery and Data Mining. 1996. P. 226–231.
- Hata M. Empirical formula for propagation loss in land mobile radio services. IEEE Transactions on Vehicular Technology. 1980. V. 29. № 3. P. 317–325. DOI 10.1109/T-VT.1980.23859.
- Okumura Y., Ohmori E., Kawano T., Fukuda K. Field Strength and its variability in VHF and UHF land mobile radio service. Review of the Electrical Communication Laboratory. 1968. V. 16. № 9–10. P. 825–873.
- Fontan F.P., Espineira P.M. Modeling the Wireless Propagation Channel A Simulation Approach with MATLAB. John Wiley & Sons Ltd. 2008. 268 p.
- 3GPP TS 36.133 LTE. Evolved Universal Terrestrial Radio Access (E-UTRA). Requirements for support of radio resource management. Release 15. [Electronic resource] – Access mode: https://iteh-standards.uc.r.appspot.com/catalog/standards/etsi/919b696f-cd37-4b1a-8667-aaefdc51fd50/etsi-ts-136-133-v15-8-0-2019-10, date of reference 18.06.2024.