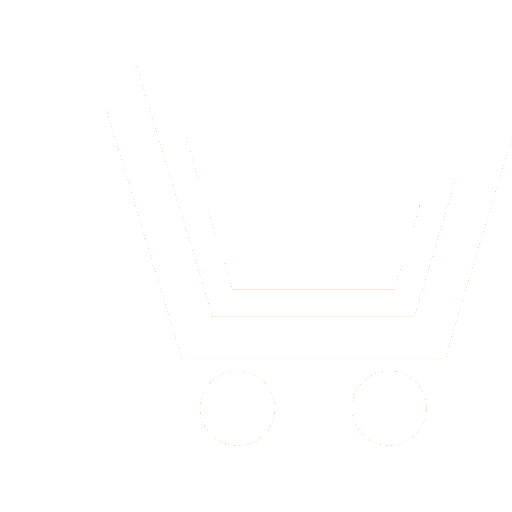
S.B. Zhironkin1, Yu.N. Kotenko2, V.O. Chervakov3, V.V. Prokhorenko4
1,2 Aerospace defense Military Academy named after Marshal of the Soviet Union Georgiy Zhukov (Tver, Russia)
3,4 Bauman Moscow State Technical University (Moscow, Russia)
1 iadrin@mail.ru, 3 vchervakov@bmstu.ru, 4 prokhorenkoww@bmstu.ru
In connection with the development of artificial neural network (ANN) technologies, it is of interest to compare the robustness of classifier systems using the optimal voting rule and ANN. Robust algorithms are required to have high (though not the highest) efficiency in the case of planned situations, and acceptable efficiency in case of predetermined deviations from the plan (model). The robustness of the optimal voting rule and the neural network to the inaccuracy of computing the posteriori probabilities of partial solutions of the combined classifiers was investigated. It was found that in calculating the posterior probabilities with the error the neural network has provided a better performance than the optimal voting rule, showing the property robustness to the inaccuracy of input data. The use of classifiers utilizing ANN allows to increase robustness of algorithms in the signal receivers.
Zhironkin S.B., Kotenko Yu.N., Chervakov V.O., Prokhorenko V.V. Robustness of classifier systems using the optimal voting rule and neural network. Electromagnetic waves and electronic systems. 2024. V. 29. № 3. P. 81−87. DOI: https://doi.org/10.18127/ j15604128-202403-08 (in Russian)
- Gorelik A.L., Barabash Y.L., Krivosheev O.V., Epstein S.S. Selection and recognition based on location information. Ed. by A.L. Gorelik. M.: Radio and Communications. 1990. 240 p. (in Russian)
- Barabash Yu.A. Collective statistical solutions for recognition. M.: Radio and communications. 1983. 224 p. (in Russian)
- Radio electronic systems: fundamentals of construction and theory: Handbook. Ed. by Ya.D. Shirman. Ed. 2nd ed. and additional. M.: Radio Engineering. 2007. 510 p. (in Russian)
- Efimenko V.S., Kharisov V.N., Strebkova E.G. Application of neural networks in optimal filtration problems. Radio Engineering. 2000. № 7. P. 56–61. (in Russian)
- Chervakov V.O., Zhironkin S.B., Zubarev A.S. Indicators of the current quality of statistical solutions in complex detection and recognition systems. Successes of modern radio electronics. 2016. № 11. P. 93–97. (in Russian)
- Zhironkin S.B., Chervakov V.O., Kurnykov A.R. Optimal accounting of current reliability in radio systems with two-stage decision-making. Bulletin of Aerospace Defense. 2020. № 2 (26). P. 67–73. (in Russian)
- Tikhonov V.I., Kharisov V.N. Statistical analysis and synthesis of radio engineering devices and systems. M.: Radio and communications. 1991. 608 p. (in Russian)
- Medvedev V.S., Potemkin V.G. Neural networks. MATLAB. M.: DIALOG-MIFI. 2002. 496 p. (in Russian)