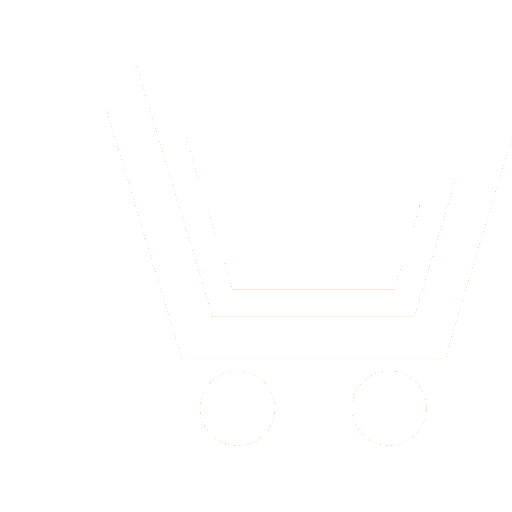
I.Yu. Eremeev1, K.A. Neretina2, V.V. Pechurin3
1−3 Military Space Academy named after A.F. Mozhaisky (Saint-Petersburg, Russia)
Trends in the development of modern communication systems and data transmission are characterized by the mass application of OFDM signals in various radio networks operating on Wi-Fi and WiMax standards. The multi-signal and multi-parameter nature of the uncertainty of the current signal environment, its high dynamics, diversity and variability of operating conditions necessitate the improvement of radio control methods for timely and accurate detection of illegitimate users. The existing approaches based on application of artificial neural networks of type YOLOv5, are intended for the decision of problems of detection and recognition of objects on images. And the issues of substantiation of procedures for preprocessing data from the output of radio control means in order to coordinate with input layers of artificial neural network of the detector-recognizer at present have not yet found their solution. In this regard, there is a need to study different variants of matching spectral analysis data with the input layer of the artificial neural network YOLOv5 on the numerical indicators that characterize the quality of solving the problem of OFDM signal detection in the real signal environment.
The performance of the artificial neural network was evaluated by images of the amplitude, phase and modified phase spectra of the combined OFDM signal and background noise at signal-to-noise ratios from -20 dB to 20 dB. The best results of the artificial neural network obtained by signal detection in the images of the amplitude and modified phase spectra. With a relatively equal number of detections, the accuracy of determining the localization area of the signal is higher for the amplitude spectrum images. However, the detection of OFDM signal on the modified phase spectrogram is 1.7 times faster at relatively small values of frequency and time shifts. The proposed modification of the complex spectrogram is based on the coincidence of the postfix and prefix of the OFDM word in the time domain.
The obtained results indicate the promising use of artificial neural networks to solve the problem of OFDM signal detection. Further research can be aimed at recognition by artificial neural networks of signals with different types and parameters of modulation. It will allow to solve a problem of radio control on timely detection of illegitimate users of a resource.
Eremeev I.Yu., Neretina K.A., Pechurin V.V. Investigation of the possibility of using the YOLOv5 convolutional neural network in radio monitoring complexes for detecting OFDM signals. Electromagnetic waves and electronic systems. 2023. V. 28. № 3. P. 18−27. DOI: https://doi.org/10.18127/j15604128-202303-03 (in Russian)
- Rembovsky A.M., Ashikhmin A.V., Kozmin V.A. Automated radio monitoring systems and their components. M.: Hotline-Telecom. 2018. 424 p. (in Russian)
- Rembovsky A.M., Ashikhmin A.V., Kozmin V.A. Radio monitoring – tasks, methods, means. M.: Hotline-Telecom. 2010. 624 p. (in Russian)
- Vargauzin V.A., Tsikin I.A. Methods for improving the energy and spectral efficiency of digital radio communications: textbook. allowance. St. Petersburg: BHV-Petersburg. 2013. 352 p. (in Russian)
- IEEE 802.16.3c-01/59r2. OFDM mode for the IEEE 802.16a PHY draft standard, Date Submitted 2001.05.17. 2001. 59 p.
- Puksa A.O., Shevchenko A.A. Development prospects and problems of OFDM technology. Sat. Art. winners of the IX International scientific and practical. conf. "World science: problems and innovations". 2017. P. 99–101. (in Russian)
- Fazel K., Kaiser S. Multi-carrier and spread spectrum systems: from OFDM and MC-CDMA to LTE and WiMAX, 2nd ed. Wiley Telecom. 2008. 374 p.
- Povetko P.V., Marchuk T.M., Karpushkin E.M. Features of the formation of a radio signal to control an unmanned aerial vehicle. Materials of the Republican scientific and practical conference "Information radio systems and radio technologies". Minsk: BSUIR. 2020. P. 313–315.
- Proletarsky A.V., Baskakov I.V., Chirkov D.N. Wireless Wi-Fi networks: a tutorial. M.: Intuit KNOW. 2016. 284 p.
- Vishnevsky V.M., Portnoy S.L., Shakhnovich I.V. WiMAX Encyclopedia. M.: Technosphere. 2009. 472 p. (in Russian)
- Erokhin D.Yu., Ershov M.D. Modern Convolutional Neural Networks for Object Detection and Recognition. Digital Signal Processing. 2018. No. 3. P. 64–69. (in Russian)
- Shtanko A.N. Analysis of patented inventions and utility models using convolutional neural networks. Neurocomputers: development, application. 2022. T. 24. No. 4. P. 5-17. DOI 10.18127/j19998554-202204-01. (in Russian)
- Adamova A.A., Zaikin V.A., Gordeev D.V. Methods and technologies of machine learning and neural network technologies in computer vision tasks. Neurocomputers: development, application. 2021. T. 23. No. 4. P. 25–39. DOI 10.18127/j19998554-202104-03. (in Russian)
- Lyons R. Digital Signal Processing: Second Edition. M.: Binom-Press LLC. 2006. 656 p.