350 rub
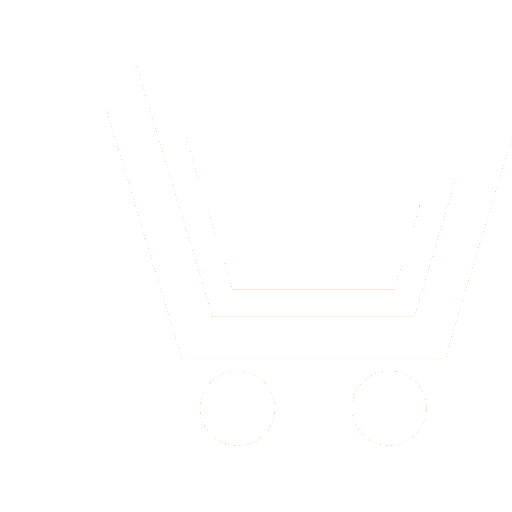
Journal Electromagnetic Waves and Electronic Systems №2 for 2017 г.
Article in number:
Methods of recognition and classification of images of structures of multicomponent materials for electronic systems
Keywords:
neural network paradigm
image recognition
nonlinear filtering
discrete cosine transform
learning sample
the reduction of dimension
Kohonen self-organizing maps
Authors:
B.M. Loginov - Dr. Sc. (Phys.-Math.), Professor, Kaluga branch of the Bauman MSTU
E-mail: bmloginov@kaluga.ru
A.P. Korzhavyi - Dr. Sc. (Eng.), Professor, Kaluga branch of the Bauman MSTU
E-mail: fn2kf@list.ru
Yu.S. Belov - Ph. D. (Phys.-Math.), Associate Professor, Kaluga branch of the Bauman MSTU
E-mail: ybs82@mail.ru
R.V. Liberov - Assistant, Kaluga branch of the Bauman MSTU
E-mail: bmloginov@kaluga.ru
Abstract:
Currently, software and computational tools capable of automating procedures to examine and identify the important features of me-tallographic polished sections, especially multicomponent materials for advanced electronic systems, if they are poorly structured image. This is due to the fact that, as a rule, these images are indistinct grain boundaries, and entire surface areas by virtue of their specific photographic connected, in turn, to the light conditions, the thermal noise of the digital camera of the matrix, its resolution, the focus area, and also with the quality of the surface preparation of the section of the test, etc.
In the present method of recognition and classification of samples is implemented model of image analysis systems, including five consecutive procedural steps, and provides specific criteria for assessing the effectiveness of the recognition system. Set out and used nonlinear filtering methods used for pretreatment of the studied images and set a rational size of the filter window.
It is confirmed that the application in the discrete cosine transform is advisable to reduce the learning sample dimension, since the reduc-tion of such samples did not affect the information content of the signal, including the effect that in the training vector remained signifi-cant frequency components, and the selected transformation giving 6-10 times to reduce the dimension of the training vectors.
Testing of the proposed methodology has shown that it is appropriate as classifier to use Kohonen self-organizing map, which is con-firmed in the given specific example of processing of images of the surface of the section of the multicomponent material used for the manufacture of components of electronic systems.
Pages: 4-12
References
- Magdeev R.G., Tashlinskijj A.G. EHffektivnost identifikacii obektov na binarnykh izobrazhenijakh s ispolzovaniem procedur psevdogradientnojj adaptacii // Radiotekhnika. 2014. № 7. S. 96−102.
- Prokhorov M.E., Ryndin JU.G. Metod ocenki kachestva raspoznavanija kosmicheskikh obektov // EHlektromagnitnye volny i ehlektronnye sistemy. 2010. № 12. S. 40−42.
- Mengchen Liu, Jiaxin Shi, Zhen Li, Chongxuan Li, Jun Zhu, Shixia Liu. Towards Better Analysis of Deep Convolutional Neural Networks // IEEE Transactions on Visualization and Computer Graphics. 2017. V. 23. № 1. P. 91−100.
- Vinokurov I.V. Metodika nejjrosetevogo raspoznavanija ehlementov registracionnogo nomera avtomobilja // EHlektromagnitnye volny i ehlektronnye sistemy. 2013. № 10. S. 40−45.
- Lorenzo Seidenari, Giuseppe Serra, Andrew D. Bagdanov, Alberto Del Bimbo. Local Pyramidal Descriptors for Image Recognition // IEEE Transactions on Pattern Analysis and Machine Intelligence. 2014. V. 36. № 5. P. 1033−1040.
- Guang-Bin Huang, Hongming Zhou, Xiaojian Ding, Rui Zhang. Extreme Learning Machine for Regression and Multiclass Classification // IEEE Transactions on Systems, Man, and Cybernetics, Part B (Cybernetics). 2012. V. 42. № 2. P. 513−529.
- Stankevich L.A. Raspoznavanie posledovatelnykh obrazov na nejjrologicheskikh setjakh // Nejjrokompjutery: razrabotka, primenenie. 2010. № 2. S. 52−58.
- Novikova N.M., Dudenkov V.M. Raspoznavanie izobrazhenijj s pomoshhju svertochnojj nejjronnojj seti i nechetkogo gibridnogo klassifikatora // Nejjrokompjutery: razrabotka, primenenie. 2015. № 2. S. 43−47.
- Esikov O.V., Sukharev E.M., Altukhov A.V., Tarasov E.A. Ocenka ehffektivnosti metodov i algoritmov obrabotki graficheskojj informacii i raspoznavanija obektov monitoringa // Naukoemkie tekhnologii. 2011. № 4. S. 54−61.
- Ulasen A.F., Kocur G.A. Metodika ocenki ehffektivnosti sistemy raspoznavanija obektov po ikh opticheskim izobrazhenijam // Naukoemkie tekhnologii. 2011. № 5. S. 45−49.
- Zongyong Cui, Zongjie Cao, Jianyu Yang, Jilan Feng, Hongliang Ren. Target recognition in synthetic aperture radar images via non-negative matrix factorization // IET Radar, Sonar & Navigation. 2015. V. 9. № 9. P. 1376−1385.
- Konurkin V.A. Raspoznavanie obektov v uslovijakh ogranichennykh resursov // Informacionno-izmeritelnye i upravljajushhie sistemy. 2010. № 6. S. 50−52.
- Jan Flusser, Sajad Farokhi, Cyril Höschl, Tomáš Suk, Barbara Zitová, Matteo Pedone. Recognition of Images Degraded by Gaussian Blur // IEEE Transactions on Image Processing. 2016. V. 25. № 2. P. 790−806.
- Ulasen A.F., Kiselev K.V. Sposob polimodelnogo raspoznavanija obektov po izobrazhenijam s primeneniem iskusstvennykh nejjronnykh setejj // Naukoemkie tekhnologii. 2011. № 1. S. 31−35.
- Hayet Boughrara, Mohamed Chtourou, Chokri Ben Amar, Liming Chen. Face recognition based on perceived facial images and multilayer perceptron neural network using constructive training algorithm // IET Computer Vision. 2014. V. 8. Is. 6. P. 729−739.
- Hongsheng He, Zhenzhou Shao, Jindong Tan. Recognition of Car Makes and Models From a Single Traffic-Camera Image // IEEE Transactions on Intelligent Transportation Systems. 2015. V. 16. № 6. P. 3182−3192.
- Bing Li, Weihua Xiong, Ou Wu, Weiming Hu, Stephen Maybank, Shuicheng Yan. Horror Image Recognition Based on Context-Aware Multi-Instance Learning // IEEE Transactions on Image Processing. 2015. V. 24. № 12. P. 5193−5205.
- Sergin A.V. Kompjuternaja model raspoznavanija: koncepcija obemljushhikh sensornykh kharakteristik // Nejjrokompjutery: razrabotka, primenenie. 2015. № 8. S. 33−39.
- Yu Zhang, Jianxin Wu, Jianfei Cai. Compact Representation of High-Dimensional Feature Vectors for Large-Scale Image Recognition and Retrieval // IEEE Transactions on Image Processing. 2016. V. 25. № 5. P. 2407−2419.
- Savchenko A.V., Milov V.R. Nejjrosetevye metody raspoznavanija kusochno-odnorodnykh obektov // Nejjrokompjutery: razrabotka, primenenie. 2014. № 11. S. 10−20.
- Kozlov P.V., JUzhakov A.A. Primenenie nejjronnojj seti na osnove kognitronov dlja raspoznavanija obrazov // Nejjrokompjutery: razrabotka, primenenie. 2014. № 12. S. 57−64.
- Baranov V.G., Milov V.R., Zaripova JU.KH., EHpshtejjn A.JU. Intellektualizacija sistemy raspoznavanija obrazov na osnove sravnenija ehffektivnosti metodov klassifikacii // Informacionno-izmeritelnye i upravljajushhie sistemy. 2010. № 2. S. 35−39.
- Koljuchkin V.JA., Nguen Kong Min, CHan Tien KHajj. Algoritmy obrabotki izobrazhenijj v sistemakh mashinnogo zrenija robotizirovannykh proizvodstvennykh linijj // Nejjrokompjutery: razrabotka, primenenie. 2014. № 3. S. 44−51.
- M. Abedini, N.C.F. Codella, J.H. Connell, R. Garnavi, M. Merler, S. Pankanti, J.R. Smith, T. Syeda-Mahmood. A generalized framework for medical image classification and recognition // IBM Journal of Research and Development. 2015. V. 59. № 2/3. P. 1:1−1:18.
- Ilias Maglogiannis, Haralambos Sarimveis, C.T. Kiranoudis, Aristotelis A. Chatziioannou, Nikos Oikonomou, Vassilis Aidinis. Radial Basis Function Neural Networks Classification for the Recognition of Idiopathic Pulmonary Fibrosis in Microscopic Images // IEEE Transactions on Information Technology in Biomedicine. 2008. V. 12. № 1. P. 42−54.
- Solovjova Z.O., Ilin V.K., Nosovskijj A.M. Tekhnologii obrabotki dannykh pri analize izobrazhenijj mikrobnykh kletok // Tekhnologii zhivykh sistem. 2010. № 2. S. 38−43.
- Solovjova Z.O., Skedina M.A., Ilin V.K., Verdenskaja N.V. Raspoznavanie obraza mikrobnykh kletok v sisteme avtomaticheskogo analiza // Tekhnologii zhivykh sistem. 2011. № 4. S. 50−55.
- Korzhavyjj A.P., Loginov B.M., Loginova M.B., Belov JU.S. Issledovanie svojjstv polimernykh kompozicionnykh materialov na osnove uglerodnykh volokon i nanotrubok // Naukoemkie tekhnologii. 2014. T. 15. № 2. S. 47−59.
- Fedoseev I.V., Korzhavyi A.P., Maramygin K.V. Formation of diamonds and other carbon phases upon the destruction of palladium carbonyl clusters // Russian Journal of Inorganic Chemistry. 2013. V. 58. № 12. P. 1443−1445.
- Bokut L.V. Raspoznavanie i klassifikacija obektov polutonovykh izobrazhenijj na osnove modificirovannogo korreljacionnogo otnoshenija // EHlektromagnitnye volny i ehlektronnye sistemy. 2009. № 7. S. 70−81.