350 rub
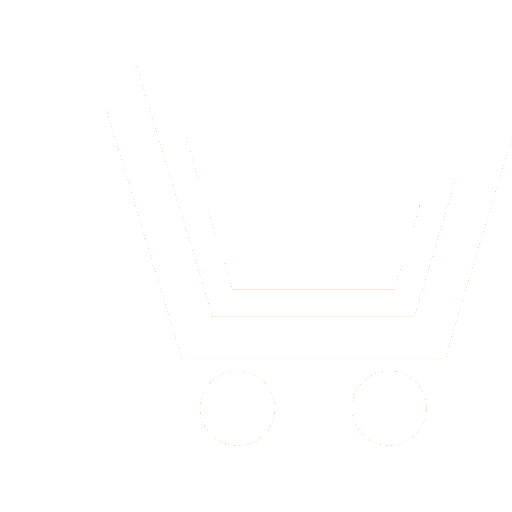
Journal Electromagnetic Waves and Electronic Systems №7 for 2009 г.
Article in number:
Recognition and Classification of Gray Image Objects on the Basis of the Modified Correlation Relation
Keywords:
system for pattern recognition
classification of gray image objects
measure of similarity
invariant information signs
Authors:
L. V. Bokut, A. Ya. Kuleshov
Abstract:
Recognition and classification of gray image objects on their contour representation are actual investigations for solving different scientific and applied problems of national economy. Now strict and complete theory of pattern recognition is not constructed yet. The structure of the recognition process is multilevel, it includes the following operations: preliminary processing, segmentation on homogeneous areas, identification, automatic classification of the investigated objects on their contour representation and visualization of the received results. At each level signs are selected, extracted or calculated, which are used to build model of the image. It should be noted that there are no effective gray image analysis algorithms and methods of clear selection of signs yet, without which no complicating decision rules will not allow the desired effect. The most informative part of gray image is the contour representation. In the absence of training samples to classify objects measures of similarity or proximity are widely applied. So our article deals with the issues of hierarchical approach application to problems of recognition, pattern recognition without using the training sample, determining the similarity measure of recognized objects and the experimental results. Definition of similarity measure and classification of objects on their contour representation by using the modified theoretical correlation relation of their vector models are proposed. In general in cluster analysis and automatic classification a given set of objects is decomposed on subsets according to the properties of objects. In doing so they require that subset include objects, which are in some ways more similar to objects from the same subset, than objects from other subsets. A concept of the object homogeneity is determined by the rule to specify value which characterizes or the distance between objects, or the degree of proximity (the similarities) of the same objects. As a measure of proximity (similarity) of contour representation of the investigated objects the authors suggested the modified theoretical correlation relation. It is determined by ratio of the sum of the intergroup variance and investigated objects to the sum of their total variance. As invariant information signs we use the weight values of the direction vectors for normalized vector model , the differences of which are multiplied by a factor of location of deducted weight value of the investigated object. It identifies a very similar forms of contour representations of the investigated objects the modified theoretical correlation relation of which is close to the unit. To classify the investigated objects on their contour representation relatively standard or the investigated object, which is considered as standard, the authors used similarity measure threshold indicators which are determined by error integral values and the calculated values of the modified theoretical correlation relation. The proposed modified theoretical correlation relation, serving as a proximity measure of the investigated objects, was examined on its correspondence to the basic mathematical requirements. The modified theoretical correlation relation has properties of symmetry, the maximum similarity of the object with itself, monotonous decrease, simple computational procedures and can be used as a measure of the similarity of the investigated gray image objects for automatic classification. Using the absolute value of the sum of intergroup variance of standard and the investigated object increases robustness of the algorithm for determining the proximity measure to the investigated objects of different configurations and orientation.
Pages: 70-81
References
- Гуревич И.Б. Проблема распознавания изображений // Распознавание, классификация, прогноз. Математические методы и их применение. М.: Наука, 1989. С. 280-329.
- Бокуть Л.В., Кулешов А.Я. Тематическая обработка полутоновых изображений // Электромагнитные волны и электронные системы. 2003. Т. 8. № 4. С. 33-43.
- Цифровая обработка сигналов и изображений в радиофизических приложениях / под ред. В.Ф. Кравченко. М.:Физматлит. 2007.
- Айвазян С.А., Бухштабер К.М., Енюков И.С., Мешалкин Л.Д. Прикладная статистика. Классификация и снижение размерности. Справочное издание / под ред. С.А. Айвазяна. М.: Финансы и статистика. 1989.
- Рязанов В.В. О построении оптимальных алгоритмов распознавания и таксономии (классификации) при решении прикладных задач // Распознавание, классификация, прогноз. Математические методы и их применение. М.: Наука. 1989. С. 229-279.
- Дасаратхи Б.В., Шила Б.В. Построение сложной системы распознавания образов: Теория и методика // Труды института инженеров по электротехнике и радиоэлектронике. 1979. Т. 67. № 5. C. 5-12.
- Павлидис Т. Иерархические методы в структурном распознавании образов // Труды института инженеров по электротехнике и радиоэлектронике. 1979. Т. 67. № 5. C. 39-49.
- Янковская А.Е., Гедике А. И., Аметов Р.В., Блейхер А.М. Инструментальное средство ИМСЛОГ-2002 для поддержки информационных технологий тестового распознавания образов // Тр. 6-й Междунар. конф. «Распознавание образов и анализ изображений: новые информационные технологии» (РОАИ-6-2002). В 2 т./ / отв. за вып. Е.И. Зайцева. НовГУ им. Ярослава Мудрого. Великий Новгород, 2002. T. 2. С. 636-640.
- Эндрюс Г. Применение вычислительных машин для обработки изображений. М.: Энергия. 1977.
- Журавлев Ю.И. Об алгебраических методах в задачах распознавания и классификации // Распознавание, классификация, прогноз. Математическиеметодыиихприменение. М.: Наука. 1989. С. 9-16.
- Samoshkin M.A., Kuleshov A.Ya., and Ablameiko S.V. Automation of the Input and Generation of Digital Models of Gray-Level Images // Pattern Recognition and image analysis. 1994. V. 4. № 1. P. 48-66.
- Абламейко С.В., Боричев С.П., Крючков А.Н. Алгоритм фильтрации изображений, использующий параллельные операции сдвига и сложения при свертке изображений с масками преобразования // Анализ цифровых изображений. Минск: ОИПИ НАН Беларуси. 2002. Вып. 1. С. 7-13.
- Коулмэн Г.Б., Эндрюс Х.С. Сегментация изображений при помощи автоматической классификации // Труды института инженеров по электротехнике и радиоэлектронике. 1979. Т. 67. № 5. С. 82-98.
- Дуда Р., Харт П. Распознавание образов и анализ сцен. М.: Мир. 1976.
- Лапа В.Г. Математические основы кибернетики. Киев: Вища школа. 1971.
- Венецкий И.Г., Кильдишев Г.С. Основы теории вероятности и математической статистики. М.: Статитстика. 1968.
- Бокуть Л.В., Кулешов А.Я. Корреляционный анализ весовых значений векторов направлений //Теоретическая и прикладная механика. Минск: БНТУ. 2007. Вып. 22. С. 187-196.
- Абламейко С.В., Кулешов А.Я. Формирование параметрической модели объектов полутоновых изображений // Цифровая обработка изображений. Минск: ИТК НАН Беларуси. 1997. Вып. 1. С. 64-78.
- Кулешов А.Я. Автоматическая классификация объектов полутоновых изображений по весовым значениям векторов направлений, сглаженных методом скользящего среднего // Весцi Нацыянальнай акадэмii навук Беларусi. Сер. фiз.-тэхн. навук. 2000. № 1. С. 98-106.
- Кулешов А.Я. Нормализация векторной модели произвольно ориентированного и масштабированного объекта полутонового изображения // Весцi Нацыянальнай акадэмii навук Беларусi. Сер. фiз.-тэхн. навук. 1998. № 2. С. 80-85.
- Кулешов А.Я. Романчик В.Д. Классификация объектов полутоновых изображений при отсутствии обучающих выборок // Анализ цифровых изображений. Минск: Объединенный институт проблем информатики НАН Беларуси. 2002. Вып.1. С. 59-68.
- Абламейко С.В., Боричев С.П., Крючков А.Н., Кулешов А.Я. Идентификация контурных объектов на космических снимках // Материалы Первого Белорусского космического конгресса (28-30 октября 2003 г., Минск). Минск: Объединенный институт проблем информатики Национальной академии наук Беларуси. 2003. C. 143-145.
- Раушенбах Г.В. Меры близости и сходства // Анализ нечисловой информации в социологических исследованиях. М.: Наука. 1985. C. 169-203.
- Абламейко С.В., Кулешов А.Я. Определение меры сходства контуров объектов, аппроксимированных дугами окружностей //. Тез. докл. 2-й Всероссийской с участием стран СНГ конференции «Распознавание образов и анализ изображений». М. 1995. Ч. 2. С. 53-56.
- Бокуть Л.В., Кулешов А.Я. Адаптивный алгоритм классификации объектов полутоновых изображений по весовым значениям векторов направлений, сглаженных методом скользящего среднего // Электромагнитные волны и электронные системы. 2001. Т. 6. № 2. С. 107-112.