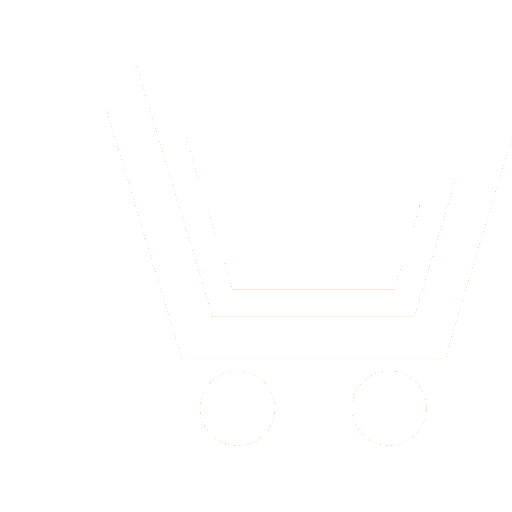
N.V. Dorofeev1, R.V. Sharapov2, M.S. Goryachev3
1–3 Vladimir State University named after A.G. and N.G. Stoletovs (Vladimir, Russia)
1 dorofeevnv@yandex.ru, 2 sharapov76@gmail.com, 3 maximgoryachev97@yandex.ru
The use of a smartphone to monitor human health has been of interest for more than a decade. However, currently its main functionality is reduced to tracking human movements (mainly gait) and evaluating statistical indicators. Using a smartphone to predict changes in the state of health by gait is a little-studied area. Existing research in this area is currently limited only to diagnostic tasks, which are solved based on machine learning methods in classifying statistical data on the gait of healthy and sick people. The aim of the work is to study the effectiveness of machine learning algorithms for predicting headaches based on the gait accelerogram in an automated medical decision support system based on measurements by a mobile device. The paper describes a technique for collecting and preprocessing gait data based on the use of a mobile phone.
The sample size for the study included 398 examples of accelerograms reflecting the double steps of one of the volunteers (314 for training and 84 for testing). The sample consisted of measurements that preceded the measurements (3–4 hours) with a note in the questionnaire about head pain and measurements in the absence of any changes in the well-being of the volunteer. The sample was balanced by the number of measurements in each class. To improve the quality of the selection of informative sections of the accelerogram, the obtained gait data were processed by the author's algorithm.
To predict the occurrence of headaches, the following classifiers were used in the implementation of the Scikit-learn package: support vectors, gradient boosting, adaptive boosting, bagging, random forest, voting regressor and multilayer perceptron (MLP). The best results were shown by the classifiers Random Forest (Accuracy = 0.8) and Adaptive boosting (Accuracy = 0.78). Next, predictions were made based on the direct distribution network from the Keras package of the TensorFlow environment. The prediction was carried out in several steps. When using ensembles of neural networks from 7 and more, the Accuracy value is 0.97.
The results of a study of the effectiveness of various machine learning algorithms for predicting headaches based on the analysis of gait accelerograms are presented. It is revealed that the most accurate predictions can be obtained using ensembles of neural networks.
The results obtained during the study, using the example of predicting headaches, show the possibility of conducting remote health monitoring by analyzing gait data using a mobile phone in real conditions. The application of the proposed algorithm in practice made it possible to increase the accuracy of predicting headaches according to the gait accelerogram to 0.97.
Dorofeev N.V., Sharapov R.V., Goryachev M.S. Using a smartphone to predict the appearance of a headache based on the gait accelerogram. Biomedicine Radioengineering. 2025. V. 28. № 1. P. 75–82. DOI: https:// doi.org/10.18127/j15604136-202501-06
(In Russian)
- Mathkar D.M., Mathkar N., Bassfar Z., Bantun F., Slama P., Ahmad F., Haque S. Multirole of the internet of medical things (IoMT) in biomedical systems for managing smart healthcare systems: An overview of current and future innovative trends. Journal of infection and public health. 2024. V. 17. № 4. P. 559–572. doi: https://doi.org/10.1016/j.jiph.2024.01.013.
- Gard M., Parihar A., Rahman M.S. Advanced and personalized healthcare through integrated wearable sensors (versatile). Materials advances. 2024. V. 5. № 2. P. 432–452. doi:10.1039/d3ma00657c.
- Guler U., Tufan T. B., Chakravarti A., Jin Y., Ghovanloo M. Implantable and wearable sensors for assistive technologies. Encyclopedia of sensors and biosensors. 2023. V. 3. P. 449–473. doi: https://doi.org/10.1016/B978-0-12-822548-6.00072-8.
- Leibold A., Ali D. M., Harrop J., Sharan A., Vaccaro A.R., Sivaganesan A. Smartphone-based activity tracking for spine patients: current technology and future opportunities. Word neurosurgery: X. 2024. V. 21. 100238. P. 1–7. doi: https://doi.org/10.1016/j.wnsx.2023.100238.
- Sikander S., Biswas P., Kulkarni P. Recent advancements in telemedicine: surgical, diagnostic and consultation devices. Biomedical engineering advances. 2023. V. 6. P. 1–15. doi: https://doi.org/10.1016/j.bea.2023.100096.
- Thapa R., Poudel S., Krukiewicz K., Kunwar A. A topical review on AI-interlinked biodomain sensors for multi-purpose applications. Measurement. 2024. V. 227. 114123. doi: https://doi.org/10.1016/j.measurement.2024.114123.
- Dorofeev N.V., Sharapov R.V., Goryachev M.S., Romanov R.V. Features of smartphone diagnostics of human health based on gait parameters. Proceedings of the 2023 IEEE 16th International scientific and technical conference actual problems of electronic instrument engineering (APEIE). Novosibirsk: Russian Federation. 2023. P. 280–283. doi: https://doi.org/10.1109/APEIE59731.2023.10347741.
- Jayasinghe U., Hwang F., Harwin W.S. Comparing loose clothing-mounted sensors with body-mounted sensors in the analysis of walking. Sensors. 2022. V. 22. № 17. 6605. P. 1–16. doi: https://doi.org/10.3390/s22176605.
- Jiao Y., Hart R., Reading S., Zhang Y. Systematic review of automatic post-stroke gait classification systems. Gait & Posture. 2024. V. 109. P. 259–270. doi: https://doi.org/10.1016/j.gaitpost.2024.02.011.
- Parashar A., Parashar A., Shabaz M., Gupta D., Sahu A.K., Khan M.A. Advancements in artificial intelligence for biometrics: A deep dive into model-based gait recognition techniques. Engineering applications of artificial intelligence. 2024. V. 130. 107712. doi: https://doi.org/10.1016/j.engappai.2023.107712.
- Aartolahti E. Comparison of spatiotemporal gait parameters in indoor and outdoor walking among older adults. Archives of physical medicine and rehabilitation. 2024. V. 105. № 4. P. e85–e86. doi: https://doi.org/10.1016/j.apmr.2024.02.240.
- Lin G., Zhao X., Wang W., Wilkinson T. The relationship between forward head posture, postural control and gait: a systematic review. Gait & Posture. 2022. V. 22. P. 316–329. doi: https://doi.org/10.1016/j.gaitpost.2022.10.008.
- Zawada MS S.J., Aissa MBBS N.H., Conte MD G.M., Pollock MS B.D., Athreya MS A.P., Erikson MD B.J., Demaerschalk MSc MD B.M.
In situ physiologic and behavioral monitoring with digital sensors for cerebrovascular Disease: A Scoping Review. Mayo Clinic Proceedings: Digital Health. 2023. V. 1. № 2. P. 139–160. doi: https://doi.org/10.1016/j.mcpdig.2023.03.007. - Liang A. Assessing gait dysfunction severity in Parkinson’s Disease using 2-Stream spatial-temporal neural network. Journal of biomedical informatics. 2024. 104679. doi: https://doi.org/10.1016/j.jbi.2024.104679.
- Dorofeev N., Sharapov R., Goryachev M., Kochetkova S., Kostyukhina A. Observing temporary changes in gait using a mobile phone. E3S Web of Conferences. 2024. V. 541. 03007. P. 1–6. doi: https://doi.org/10.1051/e3sconf/202454103007.
- Recenti M., Garguilo P., Chang M., Ko S.B., Kim T.J., Ko S.U. Predicting stroke, neurological and movement disorders using single and dual-task gait in Korean older population. Gait & Posture. 2023. V. 105. P. 92–98. doi: https://doi.org/10.1016/j.gaitpost.2023.07.282.
- Abujrida H., Agu E., Pahlavan K. DeepMed: Deep learning-based medication adherence of Parkinson’s disease using smartphone gait analysis. Smart Health. 2023. V. 30. 100430. doi: https://doi.org/10.1016/j.smhl.2023.100430.
- Gabriel C.L., Pires I.M., Coelho P.J., Zdravevski E., Lameski P., Mewada H., Madeira F., Garcia N.M., Carreto C. Mobile and wearable technologies for the analysis of ten meter walk test: A concise systematic review. Heliyon. 2023. V. 9. № 6. e16599. P. 1–29. doi: https://doi.org/10.1016/j.heliyon.2023.e16599.
- Dorofeev N.V., Grecheneva A.V. Algorithm for motion detection and gait classification based on mobile phone accelerometer data. Izvestiya of Saratov University. Mathematics. Mechanics. Informatics. 2023. V. 23. № 4. P. 531–543. doi: https://doi.org/10.18500/ 1816-9791-2023-23-4-531-543.
- Gabriel C.L., Pires I.M., Goncalves N.J., Coelho P.J., Zdravevski E., Lameski P., Albuquerque C., Garcia N.M., Carreto C. Ten meter walk test with mobile devices: A dataset with accelerometer, magnetometer, and gyroscope. Data in Brief. 2024. V. 52. 109867. P. 1–28. doi: https://doi.org/10.1016/j.dib.2023.109867.
- Regev K., Eren N., Yekutieli Z., Karinski K., Massri A., Vigiser I., Kolb H., Piura Y., Karni A. Smartphone-based gait assessment for multiple sclerosis. Multiple sclerosis and related disorders. 2024. V. 82. 105394. doi: https://doi.org/10.1016/j.msard.2023.105394.
- Avola D., Cinque L., Marsico M.D., Fagioli A., Foresti G.L., Mancini M., Mecca A. Signal enhancement and efficient DTW-based comparison for wearable gait recognition. Computers & Security. 2024. V. 137. 103643. P. 1–8. doi: https://doi.org/10.1016/ j.cose.2023.103643.
- Gadaleta M., Rossi M. IDNet: Smartphone-based gait recognition with convolutional neural networks. Pattern recognition. 2018. V. 74. P. 25–37. doi: https://doi.org/10.1016/j.patcog.2017.09.005.
- Shaban M. A novel variational mode decomposition based convolutional neural network for the identification of freezing of gait intervals for patients with Parkinson’s disease. Machine learning with applications. 2024. V. 16. 100553. P. 1–12. doi: https://doi.org/ 10.1016/j.mlwa.2024.100553.
- Sezavar A., Atta R., Ghanbari M. DCapsNet: Deep capsule networks for human activity and gait recognition with smartphone sensors. Pattern recognition. 2024. V. 147. 110054. doi: https://doi.org/10.1016/j.patcog.2023.110054.
- Shen S., Sun S.S., Li W.J., Wang R.C. A classifier based on multiple feature extraction blocks for gait authentication using smartphone sensors. Computer and electrical engineering. 2023. V. 108. 108663. doi: https://doi.org/10.1016/j.compeleceng.2023.108663.