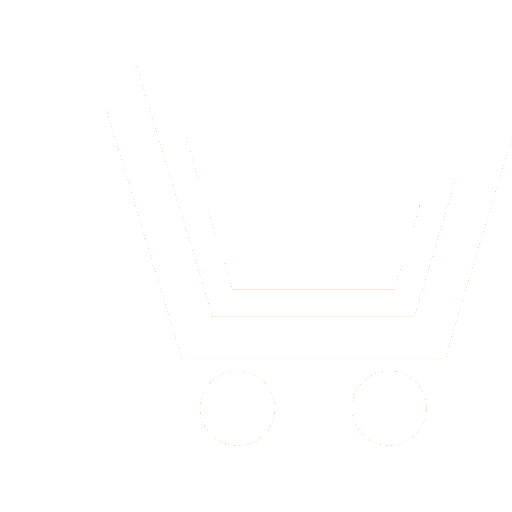
V.V. Khryashchev1, N.V. Kotov2, A.A. Tikhomirov3, I.S. Nenakhov4
1–4 P.G. Demidov Yaroslavl State University (Yaroslavl, Russia)
1 v.khryashchev@uniyar.ac.ru, 2 nik-kotov-98@inbox.ru, 3 atikhomirov.00@gmail.com, 4 ilya.nenakhov@gmail.com
The value of the colonoscopy procedure as an effective screening diagnostic method depends on the quality of the examination of the colon mucosa. In this case, it is necessary to bring the colonoscope to the dome of the cecum in more than 95% of cases. The presence of the dome area in the saved images and video sequences of the study indicates compliance with one of the important regulations for the quality of the colonoscopic procedure. The goal of this paper is to study algorithms based on deep machine learning methods for detecting the dome of the cecum in video images of a colonoscopic examination of the intestine. A database of 43 patients consisting of 4618 images of the dome of the cecum was collected for training and testing the algorithms. The developed combined ADCT-OP algorithm shows the advantage achieved through the use of the optical flow analysis algorithm, which is 0.14 according to the F1 metric. It is shown that the proposed algorithm can be used not only for post-processing of archived video data, but also for its application in real time. The proposed algorithm will allow for an objective procedure for quality control of colonoscopy examination in terms of reaching the dome of the cecum in accordance with medical regulations.
Khryashchev V.V., Kotov N.V., Tikhomirov A.A., Nenakhov I.S. Combined image analysis algorithm for quality control of colonoscopy procedure. Biomedicine Radioengineering. 2025. V. 28. № 1. P. 64–74. DOI: https:// doi.org/10.18127/j15604136-202501-05 (In Russian)
- Gudfellou Ya., Bendzhio I., Kurvill` A. Glubokoe obuchenie. DMK Press. 2017. 652 s.
- Zhou S.K., Greenspan H., and Shen D. Deep Learning for Medical Image Analysis. Elsevier Science. 2017.
- Il`yasova N.Yu., Lyakin M.Ya., Demin N.S. Vy`yavlenie simptoma matovogo stekla na izobrazheniyax komp`yuternoj tomografii lyogkix na osnove analiza grupp teksturny`x priznakov. Biomedicinskaya radioe`lektronika. 2024. T. 27. № 2. S. 13–17.
- Bajdin G.S., Bukreev D.D. Metodika raspoznavaniya zlokachestvenny`x novoobrazovanij v zheludke cheloveka na snimkax komp`yuternoj tomografii s ispol`zovaniem algoritmov obrabotki izobrazhenij. Biomedicinskaya radioe`lektronika. 2019. № 5. S. 5–14.
- Abdullaev N.T., Musevi U.N., Pashaeva K.Sh. Primenenie razlichny`x metodov optimizacii pri raschete pogreshnosti nejronnoj seti dlya diagnostirovaniya parazitarny`x zabolevanij zheludochno-kishechnogo trakta. Biomedicinskaya radioe`lektronika. 2021. № 1. S. 29–34.
- Palevskaya S.A., Korotkevich A.G. E`ndoskopiya zheludochno-kishechnogo trakta. M.: GE`OTAR-Media, 2020. 752 s.
- Munzer B., Schoeffmann K., Boszormenyi L. Content-based processing and analysis of endoscopic images and videos: A survey. Multimed. Tools Appl. 2018. 77. Р. 1323–1362.
- Kuvaev R.O., Nikonov E.L., Kashin S.V., Kapranov V.A., Gvozdev A.A. Kontrol` kachestva e`ndoskopicheskix issledovanij, perspektivy` avtomatizirovannogo analiza e`ndoskopicheskix izobrazhenij. Kremlevskaya medicina. Klinicheskij vestnik. 2013. T. 2. S. 51–56.
- Kashin S.V., Nikonov E.L., Nexajkova N.V., Lileev D.V. Standarty` kachestvennoj kolonoskopii (posobie dlya vrachej). Dokazatel`naya gastroe`nterologiya. 2019. 8(1-2):3–32.
- Leiman D.A., Metz D.C., Ginsberg G.G., Howell J.T., Mehta S.J., Ahmad N.A. 2016. A novel electronic medical record–based workflow to measure and report colonoscopy quality measures. Clinical Gastroenterology and Hepatology. 14(3):333–337.
- Akbar M., Shima R., Soroushmehr S.M.R., Karimi N., Samavi S., and Najarian K. Classification of informative frames in colonoscopy videos using convolutional neural networks with binarized weights. International conference of the IEEE Engineering in Medicine and Biology Society. 2018. Р. 65–68.
- Bisschops R., Areia M., Coron E., Dobru D., Kaskas B., et al. Performance measures for upper gastrointestinal endoscopy: a European Society of Gastrointestinal Endoscopy (ESGE) Quality Improvement Initiative. Endoscopy. 2016. 48(9). Р. 843–864.
- Ballesteros C., Trujillo M., Mazo C., Chaves D., Hoyos J. Automatic classification of non-informative frames in colonoscopy videos using texture analysis. In: Iberoamerican congress on pattern recognition. Cham: Springer. 2016. 401–408.
- Bernstein C., Thorn M., Monsees K., Spell R., O’Connor J.B. A prospective study of factors that determine cecal intubation time at colonoscopy. Gastrointestinal Endoscopy. 2005. 61(1):72–75.
- Cho M., Kim J.H., Kong H.J., Hong K.S., Kim S. A novel summary report of colonoscopy: timeline visualization providing meaningful colonoscopy video information. International Journal of Colorectal Disease. 2018. 33(5):549–559.
- Xryashhev V.V., Zav`yalov D.V., Anderzhanova A.S. Klassifikaciya e`ndoskopicheskix izobrazhenij ust`ya cherveobraznogo otrostka na osnove metodov glubokogo mashinnogo obucheniya. Cifrovaya obrabotka signalov. 2023. № 1. S. 35–38.
- Xryashhev V.V. Ispol`zovanie metodov glubokogo mashinnogo obucheniya v zadache obnaruzheniya kupola slepoj kishki na videodanny`x kolonoskopicheskogo issledovaniya. Modeli, sistemy`, seti v e`konomike, texnike, prirode i obshhestve. 2023. № 4. S. 133–141.
- Kotov N.V., Xryashhev V.V., Tixomirov A.A. Issledovanie nejrosetevoj arxitektury` YOLO dlya obnaruzheniya izobrazheniya kupola slepoj kishki v kolonoskopii. Cifrovaya obrabotka signalov i ee primenenie (DSPA-2024) / Dokl. 26-j mezhdunar. konf. M., 2024. S. 151–155.
- Zhang Y., Sun P., Jiang Y., Yu D., Weng F., Yuan Z., Luo P., Liu W., Wang X. Bytetrack: Multi-object tracking by associating every detection box. European Conference on Computer Vision. Springer. 2022. Р. 1–21.
- Horn B., Schunck B. Determining optic flow. Artificial intelligence. 1981. V. 17. Р. 185–203.
- Klette R. Komp`yuternoe zrenie. Teoriya i algoritmy` / Per. s angl. A.A. Slinkin. M.: DMK Press. 2019. 506 s.
- Cho M., Kim J.H., Hong K.S., Kim J.S., Kong H.-J., Kim S. 2019. Identification of cecum time-location in a colonoscopy video by deep learning analysis of colonoscope movement. PeerJ 7:e7256.
- Priorov A.L., Xryashhev V.V., Topnikov A.I. Obrabotka i peredacha mul`timedijnoj informacii: uchebnoe posobie. Yaroslavl`: YarGU. 2022. 248 s.