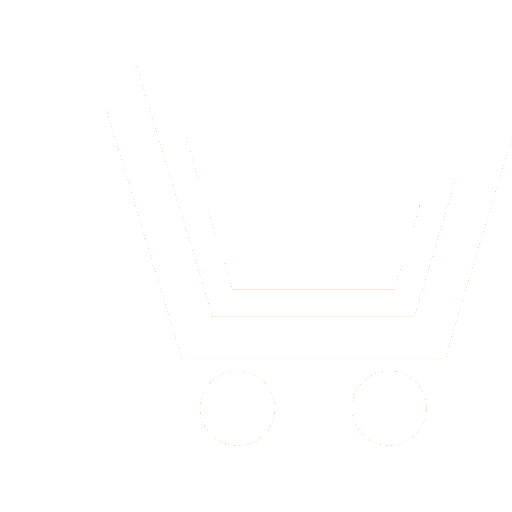
D.N. Klypin1, A.E. Sulavko2, A.E. Sulavko3, Yu.A. Varkentin4, A.M. Vulfin5
1,2,4,5 Omsk State Technical University (Omsk, Russia)
3 BUKOO Medical and Sanitary Unit No. 4 (Omsk, Russia)
1 lan@omgtu.ru, 2 sulavich@mail.ru, 3 borya.zhizha@mail.ru, 4 varkentinyuri@gmail.com, 5 vulfin.alexey@gmail.com
Automatic detection of long and short QT integral syndrome is an extremely urgent task. Long and short QT syndromes are predictors of life-threatening cardiac arrhythmias. Manual and automatic (based on classical signal accumulation algorithms) determination of the QT interval length does not always allow determining the presence of pathology. A hybrid model for determining long and short QT syndrome is proposed, which combines the use of classical algorithms for automatic detection of the expected location of the QT complex with subsequent classification of these complexes to search for pathologies using artificial neural networks. We proposed 2 architectures (convolutional and recurrent) of neural networks and achieved accuracy for neural network models by the metric F1 = 0.98-0.99. When classifying ECGs, we used an approach called Single Lead First. It consists in the fact that the neural network does not accept all 12 leads at once, as a single image, but analyzes each complex in each lead independently. This allows to reduce the dimensionality of the problem, simplifying the training, and also to increase the volume of the training sample several times. Further research will be aimed at analyzing the late potentials of the ventricles on high-resolution ECG, which are a predictor of a number of serious heart disorders, such as sudden death syndrome, myocardial infarction, ventricular tachyarrhythmia.
Klypin D.N., Sulavko A.E., Sulavko A.E., Varkentin Yu.A., Vulfin A.M. Neural network model for recognizing long and short QT syndrome on ECG. Biomedicine Radioengineering. 2025. V. 28. № 1. P. 38–52. DOI: https:// doi.org/10.18127/j15604136-202501-03
(In Russian)
- Ostroumova O.D., Goloborodova I.V. Lekarstvenno-inducirovannoe udlinenie intervala QT: rasprostranennost`, faktory` riska, lechenie i profilaktika. Consilium Medicum. 2019. T. 21. № 5. C. 62–67. doi: 10.26442/20751753.2019.5.190415
- Fomina I.G., Tarzimanova A.I., Vetluzhskij A.V. Sindrom korotkogo intervala QT. Kardiovaskulyarnaya terapiya i profilaktika. 2006; 5(7):83–85.
- Neumann B., Suzanne V.A., Hermans Ben J.M., Lieve Krystien V.V., Cömert D., Beckmann B.-M., Clur S.-A.B, Blom N.A., Delhaas T., Wilde A.M., Kääb S., Postema P.G, Sinner M.F. Manual vs. automatic assessment of the QT-interval and corrected QT, EP Europace. 2023. V. 25. Iss. 9. euad213, https://doi.org/10.1093/europace/euad213
- Sin`kov A.V. Sindrom udlinennogo i ukorochennogo intervala QT v klinicheskoj praktike. RMZh. 2014;23:1732.
- Kardiologiya: uchebnik / pod red. I.E. Chazovoj. M.: GE`OTAR-Media. 2024. 920 s.:il.
- Bos JM, Attia ZI, Albert DE, Noseworthy PA, Friedman PA, Ackerman MJ. Use of Artificial Intelligence and Deep Neural Networks in Evaluation of Patients With Electrocardiographically Concealed Long QT Syndrome From the Surface 12-Lead Electrocardiogram. JAMA Cardiol. 2021 May 1;6(5):532–538. doi: 10.1001/jamacardio.2020.7422. PMID: 33566059; PMCID: PMC7876623
- Aufiero S., Bleijendaal H., Robyns,T. et al. A deep learning approach identifies new ECG features in congenital long QT syndrome. BMC Med 20, 162 (2022). https://doi.org/10.1186/s12916-022-02350-z
- Alexander A. Suarez Leon, Carolina Varon, Rik Willems, Sabine Van Hiffel, Carlos R. Vazques Seisdedos. PyECG: A software tool for the analysis of the QT interval in the electrocardiogram. Revista de Ingenieria Electronica Automatica y Comunicaciones. 2018. V. 39 (2). Р. 54–69.
- Holmstrom, L., Chugh, H., Nakamura, K. et al. An ECG-based artificial intelligence model for assessment of sudden cardiac death risk. Commun Med 4, 17 (2024). https://doi.org/10.1038/s43856-024-00451-9
- Negar Raissi Dehkordi, Nastaran Raissi Dehkordi, Kimia Karimi Toudeshki, Mohammad Hadi Farjoo. Artificial Intelligence in Diagnosis of Long QT Syndrome: A Review of Current State, Challenges, and Future Perspectives. Mayo Clinic Proceedings: Digital Health. 2024. V. 2. Iss. 1. P. 21–31. doi: 10.1016/j.mcpdig.2023.11.003