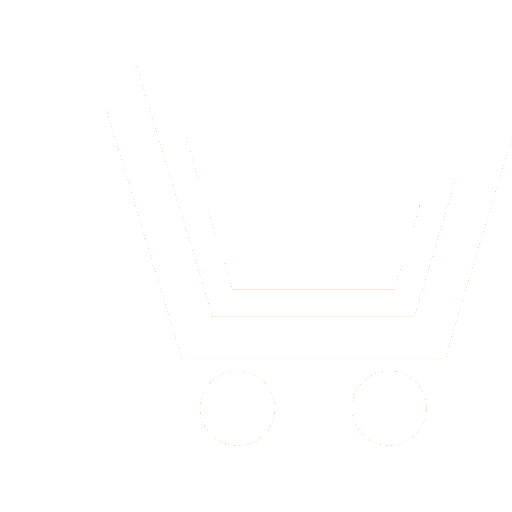
D.V. Zhuravlev1, A.N. Golubinsky2, A.A. Tolstykh3, A.A. Reznichenko4
1, 4 Voronezh State Technical University (Voronezh, Russia)
2 A.A. Harkevich Institute of Information Transmission Problems of the Russian Academy of Sciences (Moscow, Russia)
3 RTK LLC (Moscow, Russia)
1 ddom1@yandex.ru, 2 annikgol@mail.ru, 3 tolstykh.aa@yandex.ru, 4 andrei.reznichencko2017@yandex.ru
The classification of motor imagery based on electroencephalogram signals is a complex applied task. Especially when it is implemented in portable small-sized brain-computer interfaces. Currently, there is no single solution that allows the classification of motor imagery with sufficient reliability and accuracy so that this technology can be used everywhere. Among the numerous models of artificial neural networks used to classify motor imagery, a new and not sufficiently studied direction is the use of diffusion neural networks for such operations. In relation to the brain-computer interfaces, to conduct a study of the effectiveness of using various models of diffusion neural networks in the tasks of classifying motor imagery based on electroencephalogram signals. To show the versatility of diffusion networks for working with dictator-dependent and dictator-independent datasets. Also, to conduct a study of the effect of pre-filtering of signals on the quality of classification. The efficiency of classifying motor images of both real and imaginary hand movements was studied using classifiers based on models of diffusion neural networks TimeGrad, ScoreGrad and DVa. Using the standard metrics "accuracy" and ROC-AUC, an analysis of the effectiveness of classifications, both dictator-dependent and dictator-independent data sets, was carried out. The maximum value of the "accuracy" metric was 60.93%, and the ROC-AUC metric was 0.813832 for a third-party dataset. The effect of filtering electroencephalogram signals on classification accuracy is also studied. The efficiency of diffusion neural network models is compared with the classical convolutional neural network model. This study makes a significant practical contribution to the work on improving technologies in the field of developing brain-computer interfaces, as new options for constructing classifiers based on models of diffusion neural networks have been explored. Thanks to the conducted computational experiments and their analysis, a number of conclusions and recommendations were made that will allow us to develop better classifiers of motor images in brain-computer interfaces.
Zhuravlev D.V., Golubinsky A.N., Tolstykh A.A., Reznichenko A.A. Investigation of the effectiveness of classifiers of motor images constructed using diffusion models of artificial neural networks. Biomedicine Radioengineering. 2025. V. 28. № 1. P. 18–37. DOI: https:// doi.org/10.18127/j15604136-202501-02 (In Russian)
- Oficial`ny`j sajt proekta Neuralink. URL: https://neuralink.com/ (data obrashheniya: 16.09.2024).
- Pfurtscheller G., Neuper C. Motor imagery and direct brain-computer communication. Proceedings of the IEEE. 2001. V. 89. № 1. P. 1123–1134.
- Brodu N., Lotte F., Lécuyer A. Exploring Two Novel Features for EEG-based Brain-Computer Interfaces: Multifractal Cumulants and Predictive Complexity. Neurocomputing. 2010. V. 79. № 2. P. 87–94.
- Nambiar A., Sivakumar R., Subramaniam S. Detection of Four Class Motor Imagery from EEG Signal for Brain-Computer Interface Applications. Applied Mathematics, Modeling and Computer Simulation. 2023. V. 42. P. 881–894.
- Kabir M.H., Akhtar N.I., Tasnim N., Miah A.S.M., Lee H.-S., Jang S.-W., Shin J. Exploring Feature Selection and Classification Techniques to Improve the Performance of an Electroencephalography-Based Motor Imagery Brain-Computer Interface System. Sensors. 2024. V. 24. № 15. 4989.
- Lu Y., Wang W., Lian B., He C. Feature Extraction and Classification of Motor Imagery EEG Signals in Motor Imagery for Sustainable Brain–Computer Interfaces. Sustainability. 2024. V. 16. № 15. 6627.
- Xue K., Yang J., Yao F. Optimal Linear Discriminant Analysis for High-Dimensional Functional Data. Journal of the American Statistical Association. 2023. V. 119. P. 1055–1064.
- Gupta D., Gupta U., Sarma H. Functional iterative approach for Universum-based primal twin bounded support vector machine to EEG classification (FUPTBSVM). Multimedia Tools and Ap-plications. 2023. V. 83. № 8. P. 1–33.
- Hazarika D., Gupta D., Kumar B. EEG Signal Classification Using a Novel Universum-Based Twin Parametric-Margin Support Vector Machine. Cognitive Computation. 2023. V. 16. P. 2047–2062.
- Ganaie M., Tanveer M., Jangir J. EEG signal classification via pinball universum twin support vector machine. Annals of Operations Research. 2022. V. 328. P. 451–492.
- Echtioui A., Mlaouah A., Zouch W., Ghorbel M., Mhiri C., Hamam H. A Novel Convolutional Neural Network Classification Approach of Motor-Imagery EEG Recording Based on Deep Learning. Appl. Sci. 2021. V. 11. № 21. 9948.
- Uyulan C. Development of LSTM&CNN based hybrid deep learning model to classify motor imagery tasks. Communications in Mathematical Biology and Neuroscience. 2021. Article ID. 4 P. 1–26.
- Aung H.W., Li J.J., An Y., Su S.W. EEG_RL-Net: Enhancing EEG MI Classification through Reinforcement Learning-Optimised Graph Neural Networks. arXiv.org. 2024. URL: http://arxiv.org/abs/2405.00723 (data obrashheniya: 17.09.2024).
- Tayeb Z., Fedjaev J., Ghaboosi N., Richter C., Everding L., Qu X., Wu Y., Cheng G., Conradt J. Validating Deep Neural Networks for Online Decoding of Motor Imagery Movements from EEG Signals. Sensors. 2019. V. 19. № 1. 210.
- Kurkin S.A., Picik E.N., Xramov A.E. Ispol`zovanie iskusstvenny`x nejronny`x setej dlya klassifikacii e`lektricheskoj aktivnosti golovnogo mozga v processe voobrazheniya dvizhenij u netrenirovanny`x ispy`tuemy`x. Informacionno-upravlyayushhie sistemy`. 2019. № 6. C. 77–84.
- Ai Y., Rajendran B. A Convolutional Spiking Network for Gesture Recognition in Brain-Computer Interfaces. arXiv.org. 2023. URL: http://arxiv.org/abs/2304.11106 (data obrashheniya: 19.09.2024).
- Uyulan C. Development of LSTM&CNN based hybrid deep learning model to classify motor imagery tasks. Communications in Mathematical Biology and Neuroscience. 2021. Article ID. 4. P. 1–26.
- Aung H.W., Li J.J., An Y., Su S.W. EEG_RL-Net: Enhancing EEG MI Classification through Reinforcement Learning-Optimised Graph Neural Networks. arXiv.org. 2024. URL: http://arxiv.org/abs/2405.00723 (data obrashheniya: 17.09.2024).
- Dhariwal P., Nichol A. Diffusion Models Beat GANs on Image Synthesis. In: M. Ranzato, A. Beygelzimer, Y. Dauphin, P.S. Liang, J. Wortman Vaughan (eds.) NIPS '21: Proceedings of the 35th International Conference on Neural Information Processing Systems, 6–14 December 2021, NY, United States. 2024. Curran Associates Inc. Article No. 672. P. 8780–8794.
- Saharia C., Chan W., Saxena S., Lit, L., Whang J., Denton, E., Ghasemipour S.-K.-S., Ayan B.-K., Mahdavi S.-S., Gontijo-Lopes R., Salimans T., Ho J., J Fleet D., Norouzi M. Photorealistic Text-to-Image Diffusion Models with Deep Language Understanding. In: S. Koyejo, S. Mohamed, A. Agarwal, D. Belgrave, K. Cho, A. Oh (eds.) NIPS'22: 36th International Conference on Neural In-formation Processing Systems, 28 November – 9 December 2022, New Orleans, LA, USA. 2024. jmlr.org. Article No. 2643. P. 36479–36494.
- Scheider F. ArchiSound: audio generation with diffusion. Master's thesis. ETH Zurich. 2023.
- Liu H., Chen Z., Yuan Y., Mei X., Liu X., Mandic D., Wang W., Plumbley M.-D. AudioLDM: Text-to-Audio Generation with Latent Diffusion Models. In: A. Krause, E. Brunskill, K. Cho, B. Engelhardt, S. Sabato, J. Scarlett (eds.) ICML'23: Proceedings of the 40th International Conference on Machine Learning, 23–29 July 2023, Honolulu, Hawaii, USA. 2023. jmlr.org. Article № 886. P. 21450–21474.
- Rasul K., Seward C., Schuster I., Vollgraf R. Autoregressive Denoising Diffusion Models for Multivariate Probabilistic Time Series Forecasting. In: M. Meila, T. Zhang (eds.) Proceedings of the 38th International Conference on Machine Learning (ICML 2021), 18–24 July 2021. Virtual. P. 8857–8868.
- Tosato G., Dalbagno C. M., Fumagalli F. EEG Synthetic Data Generation Using Probabilistic Diffusion Models. arXiv.org. 2023. URL: http://arxiv.org/abs/2303.06068 (data obrashheniya: 17.09.2024).
- Schalk G., J McFarland D., Hinterberger T., Birbaumer N., R Wolpaw J. BCI2000: a general-purpose brain-computer interface (BCI) system. In: IEEE Trans Biomed Eng, Jun 2004. V. 51. № 6. P. 1034–1043. DOI: 10.1109/TBME.2004.827 072
- Oficial`ny`j sajt proekta OpenBCI. URL: https://openbci.com (data obrashheniya: 11.09.2024).
- Ho J., Jain A., Abbeel P. Denoising Diffusion Probabilistic Models. In: H. Larochelle, M. Ranzato, R. Hadsell, M.F. Balcan, H. Lin (eds.) NIPS'20: 34th International Conference on Neural Information Processing Systems, 6–12 December 2020, Vancouver, Canada. Curran Associates Inc. Article № 574. P. 6840–6852.
- Kingma D.P., Welling M. Auto-Encoding Variational Bayes. arXiv.org. 2022. URL: http://arxiv.org/abs/1312.6114 (data obrashheniya: 17.09.2024).
- Nichol A., Dhariwal P. Improved Denoising Diffusion Probabilistic Models. arXiv.org. 2021. URL: https://arxiv.org/abs/2102.09672v1 (data obrashheniya: 10.09.2024).
- Sutter T.M., Daunhawer I., Vogt J.E. Generalized Multimodal ELBO. arXiv.org. 2021. URL: https://arxiv.org/abs/2105.02470 (data obrashheniya: 10.01.2024).
- Gudfellou Ya., Bendzhio I., Kurvill` A. Glubokoe obuchenie. M.: «DMKPress». 2017. 652 c.
- Sohl-Dickstein J. et al. Deep Unsupervised Learning using Nonequilibrium Thermodynamics. arXiv.org. 2015. URL: https://arxiv.org/abs/1503.03585 (data obrashheniya: 10.09.2024).
- Kingma D.P., Ba J. Adam: A Method for Stochastic Optimization. In: Y. Bengio, Y. LeCun (eds.) International Conference on Learning Representations, ICLR 2014, 14–16 April 2014, San Diego, USA. arXiv. P. 1–15. DOI: 10.48550/arXiv.1412.6980
- Xajkin L.A. Nejronny`e seti. Polny`j kurs. 2-e izd. Moskva: Izdatel`skij dom «Vil`yams», 2006. 1104 c.
- Yan T., Zhang H., Zhou T., Zhan Y., Xia Y. ScoreGrad: Multivariate Probabilistic Time Series Fore-casting with Continuous Energy-based Generative Models. To be published in CoRR. arXiv.org. 2021. URL: https://arxiv.org/abs/2106.10121 (data obrashheniya: 23.09.2024).
- Koa K.-J.-L., Ma Y., Ng R., Chua T.-S. Diffusion Variational Autoencoder for Tackling Stochasticity in Multi-Step Regression Stock Price Prediction. In: I. Frommholz, F. Hopfgartner, M. Lee, M. Oakes (eds.) CIKM '23: Proceedings of the 32nd ACM International Conference on Information and Knowledge Management, 21–25 October 2023, Birmingham United Kingdom. Association for Computing Machinery. P. 1087–1096. https://doi.org/10.1145/3583780.3614844
- Vaswani A., Shazeer N., Parmar N., Uszkoreit J., Jones L., Gomez A.-N., Kaiser Ł., Polosukhin I. Attention is All you Need. In: I. Guyon, U. Von Luxburg, S. Bengio, H. Wallach, R. Fergus, S. Vishwanathan, R. Garnett (eds.) Advances in Neural Information Processing Systems 30 (NeurIPS 2017), 2017. Curran Associates Inc. P. 1–11.
- Oord van den A., Dieleman S., Zen H., Simonyan K., Vinyals O., Graves A., Kalchbrenner N., Senior A., Kavukcuoglu K. WaveNet: A Generative Model for Raw Audio. In: Alan W. Black (eds.) The 9th ISCA Speech Synthesis Workshop, SSW 2016, Sunnyvale, CA, USA, September 13–15, 2016. Article № 125. P. 1–15. DOI: 10.48550/arXiv.1609.03499
- Kong Z., Ping W., Huang J., Zhao K., Catanzaro B. DiffWave: A Versatile Diffusion Model for Audio Synthesis. In: ICLR 2021 Conference, 12 Jan 2021, Virtual. Article № 1087. P. 1–17. DOI: 10.48550/arXiv.2009.09761
- Vahdat A., Kautz J. NVAE: A Deep Hierarchical Variational Autoencoder. In: H. Larochelle, M. Ranzato, R. Hadsell, M.F. Balcan, H. Lin (eds.) Advances in Neural Information Processing Systems 33 (NeurIPS 2020). 2020. Curran Associates Inc. P. 19667–19679.
- Popov E.Yu., Fomenkov S.A. Detektirovanie soby`tij dvizheniya ruki v signalax E`E`G golovnogo mozga s pomoshh`yu svertochny`x nejronny`x setej. Izvestiya Volgogradskogo gosudarstvennogo texnicheskogo universiteta. 2016. № 1 (33). S. 62–67.