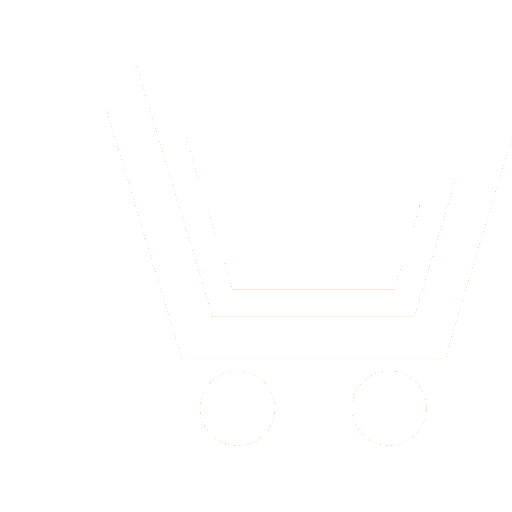
A.N. Briko1, P.E. Chibizov2, V.S. Mazeina3, V.V. Kapravchuk4, A.V. Kobelev5, S.I. Shchukin6
1–6 Moscow Bauman State Technical University (Moscow, Russia)
1 briko@bmstu.ru
Today, among the well-known methods of recording muscle activity, the most widely used method is the sEMG method. Despite the generally accepted method for analyzing neuromuscular activity, its capabilities for anthropomorphic control of bioelectric devices are limited. This is primarily due to the need for high-quality contact of the electrode system with the skin, sensitivity to sweating and external electromagnetic interference. This problem can be solved by using multimodal registration systems. The simultaneous use of several types of muscle activity signals can provide a number of potential advantages, such as increased accuracy and reliability, an expanded set of recognizable gestures, adaptability to the individual characteristics of the operator, reduction of the influence of external interference, increased functionality and comfort of use. To evaluate the efficiency of the combined use of EMG, EI, FMG signals for the analysis of various types of performed forearm actions.
The analysis of various combinations of EMG, EI, and FMG signals showed that the combination of all three provides the highest accuracy of gesture recognition. It was found that the combination of EMG and EI allows to achieve comparable results without significant loss in accuracy and efficiency.
The results of this study can be applied to the development of prosthetic control systems, virtual and augmented reality interfaces and sports medicine.
Briko A.N., Chibizov P.E., Mazeina V.S., Kapravchuk V.V., Kobelev A.V., Shchukin S.I. Evaluation of the efficiency of synthesis of bionic control systems based on the combination of EMG, EI, FMG signals. Biomedicine Radioengineering. 2024. V. 27. № 6. P. 20–29. DOI: https:// doi.org/10.18127/j15604136-202406-02 (In Russian)
- Prociow P., Wolczowski A., Amaral T.G., Dias O.P. and Filipe J. Identification of hand movements based on MMG and EMG Signals. BIOSIGNALS. 2008. V. 2. P. 534–539.
- Ke A. et al. An ultra-sensitive modular hybrid EMG–FMG sensor with floating electrodes. Sensors (Switzerland). MDPI AG. 2020. V. 20. № 17. P. 1–15.
- Rabe K.G., Fey N.P. Evaluating Electromyography and Sonomyography Sensor Fusion to Estimate Lower-Limb Kinematics Using Gaussian Process Regression. Front Robot AI. Frontiers Media S.A. 2022. V. 9. P. 1–14.
- Khushaba R.N. et al. Combined influence of forearm orientation and muscular contraction on EMG pattern recognition. Expert Syst Appl. Elsevier Ltd. 2016. V. 61. P. 154–161.
- Shahzad W. et al. Enhanced performance for multi-forearm movement decoding using hybrid IMU–SEMG interface. Front Neurorobot. Frontiers Media S.A. 2019. V. 13. P. 1–12.
- Kimoto A., Fujiyama H., Machida M. A Wireless Multi-Layered EMG/MMG/NIRS Sensor for Muscular Activity Evaluation. Sensors. MDPI. 2023. V. 23. № 3. P. 1–10.
- Briko A.N., Kobelev A.V., Shchukin S.I. Electrodes interchangeability during electromyogram and bioimpedance joint recording. Proceedings – 2018 Ural Symposium on Biomedical Engineering, Radioelectronics and Information Technology, USBEREIT 2018. Institute of Electrical and Electronics Engineers Inc. 2018. P. 17–20.
- Briko A. et al. A way of bionic control based on EI, EMG, and FMG signals. Sensors. MDPI. 2022. V. 22. № 1. P. 1–8.
- Van Der Maaten L., Hinton G. Visualizing Data using t-SNE. Journal of Machine Learning Research. 2008. V. 9. P. 2579–2605.
- Watanabe S. Information Theoretical Analysis of Multivariate Correlation. IBM Journal. 1960. P. 66–82.
- Kapravchuk V. et al. An Approach to Using Electrical Impedance Myography Signal Sensors to Assess Morphofunctional Changes in Tissue during Muscle Contraction. Biosensors (Basel). Multidisciplinary Digital Publishing Institute (MDPI). 2024. V. 14. № 2. P. 1–12.