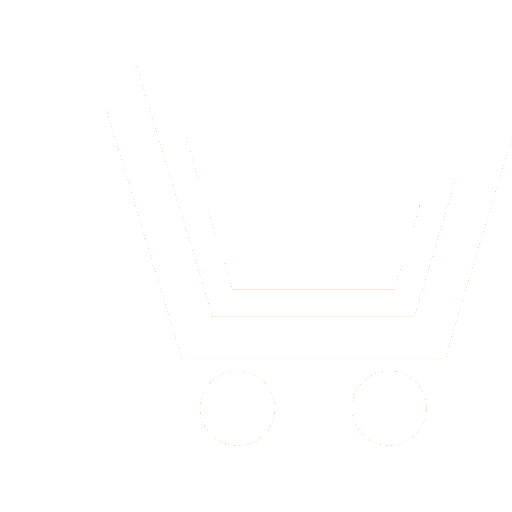
A.N. Kalinichenko1, L.A. Manilo2, A.P. Nemirko3, E.V. Sadykova4, I.V. Degterev5
1–5 St Petersburg Electrotechnical University (LETI) (Saint-Petersburg, Russian)
2 lmanilo@yandex.ru
The paper presents a neural network algorithm for cuffless blood pressure measurement based on computer analysis of the photoplethysmogram signal. An approach is proposed that allows you to significantly reduce the requirements for the size of the training sample required to build an effective machine learning model. The essence of the approach is the procedure for retraining a pre-trained neural network model using a limited amount of training data. At the same time, it is possible to achieve such values of the accuracy of estimating blood pressure values as in full-fledged training, but at the same time, the practical use of the method is significantly simplified.
Kalinichenko A.N., Manilo L.A., Nemirko A.P., Sadykova E.V., Degterev I.V. Improving the accuracy of blood pressure estimation using a photoplethysmogram due to additional training of an artificial neural network. Biomedicine Radioengineering. 2024. V. 27. № 5.
P. 23–28. DOI: https:// doi.org/10.18127/j15604136-202405-03 (In Russian).
- Jermana L. Moraes, Matheus X. Rocha, Glauber G. Vasconcelos, José E. Vasconcelos Filho, Victor Hugo C. de Albuquerque and Auzuir
R. Alexandria. Advances in Photopletysmography Signal Analysis for Biomedical Applications. Sensors. V. 18. June 9. 2018. P. 2–9. - Kengo Atomi, Haruki Kawanaka, Md. Shoaib Bhuiyan, and Koji Oguri1. Cuffless Blood Pressure Estimation Based on Data-Oriented Continuous Health Monitoring System. Hindawi Computational and Mathematical Methods in Medicine. Aprile 24. 2017. P. 3–4.
- Sagirova Z., Kuznetsova N., Gogiberidze N., Gognieva D., Suvorov A., Chomakhidze P., Omboni S., Saner H., Kopylov P. Cuffless Blood Pressure Measurement Using a Smartphone-Case Based ECG Monitor with Photoplethysmography in Hypertensive Patients. Sensors (Basel). 2021. May 19. 21(10):3525.
- Syed Ghufran Khalid, Jufen Zhang, Fei Chen, and Dingchang Zheng. Blood Pressure Estimation Using Photoplethysmography Only: Comparison between Different Machine Learning Approaches. Hindawi Journal of Healthcare and Engineering. October 23. 2018. P. 2–5.
- Moajjem Hossain Chowdhury, Md Nazmul Islam Shuzan, Muhammad E.H. Chowdhury, Zaid B. Mahbub, M. Monir Uddin, Amith Khandakar and Mamun Bin Ibne Reaz. Estimating Blood Pressure from the Photoplethysmogram Signal and Demographic Features Using Machine Learning Techniques. Sensors. June 1. 2020. P. 4–15.
- Kalinichenko A.N. Algoritm ocenki arterial`nogo davleniya po signalu fotopletizmogrammy` na osnove odnomernoj svertochnoj iskusstvennoj nejronnoj seti. Biotexnosfera. 2022. № 1(67). S. 21–27.
- Nemirko A.P., Kalinichenko A.N., Manilo L.A. Matematicheskij analiz biomedicinskix signalov i danny`x. M.: Fizmatlit. 2017. 248 s.