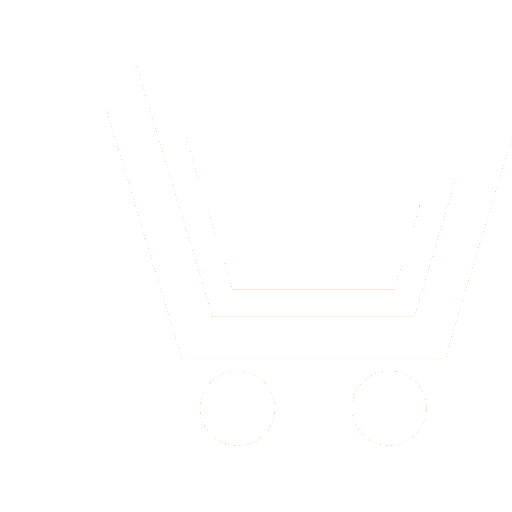
K.S. Starchenkova1, L.A. Manilo2
1,2 St. Petersburg State Electrotechnical University «LETI» (Saint Petersburg, Russia)
1 karinasmbatovna@gmail.com, 2 lmanilo@yandex.ru
Sleep apnea is a common sleep disorder that causes an increased risk of mortality and affects quality of life. Identifying cases of sleep apnea is vital to promptly treating the condition. The goal of the work is to develop a software package for automatically detecting episodes of obstructive sleep apnea syndrome. The paper presents an approach based on the study of multifractal properties of the electroencephalogram (EEG) signal for detecting sleep apnea events. The results of tests conducted using the PhysioNet CAP Sleep database indicate excellent quality of detection of sleep apnea against the background of other diseases: using the Hurst index – sensitivity (90.00%) and specificity (91.50%), using the multifractal detrended fluctuation method analysis (by spectrum width) - sensitivity (90.00%) and specificity (90.50%).The use of a sleep apnea episode recognition system ensures timely detection of the disease at an early stage and thereby improves the effectiveness of the treatment of this disease.
Starchenkova K.S., Manilo L.A. Application of multifractal analysis for recognition of sleep apnea using electroencephalogram. Biomedicine Radioengineering. 2024. V. 27. № 5. P. 17–22. DOI: https:// doi.org/10.18127/j15604136-202405-02 (In Russian).
- Gorshkov Yu.G. Mnogourovnevy`j vejvlet-analiz zvukov dy`xaniya i xrapa pri vy`yavlenii sindroma obstruktivnogo apnoe` sna. Biomedicinskaya radioe`lektronika. 2016. № 7. S. 73–78.
- Yıldız, Abdulnasır & Akin, Mehmet & Poyraz, Mustafa. (2011). An expert system for automated recognition of patients with obstructive sleep apnea using electrocardiogram recordings. Expert Syst. Appl. 38. 12880–12890. 10.1016/j.eswa.2011.04.080.
- Al-Angari HM, Sahakian AV. Automated recognition of obstructive sleep apnea syndrome using support vector machine classifier. IEEE Trans InfTechnol Biomed. 2012 May; 16(3):463-8. doi: 10.1109/TITB.2012.2185809. Epub 2012 Jan 24. PMID: 22287247; PMCID: PMC4487628.
- MG Terzano, L Parrino, A Sherieri, R Chervin, S Chokroverty, C Guilleminault, M Hirshkowitz, M Mahowald, H Moldofsky, A Rosa, R Thomas, A Walters. Atlas, rules, and recording techniques for the scoring of cyclic alternating pattern (CAP) in human sleep. SleepMed 2001 Nov; 2(6):537–553.
- Kirichenko L.O. Sravnitel`ny`j mul`tifraktal`ny`j analiz vremenny`x ryadov metodami detrendirovannogo fluktuacionnogo analiza i maksimumov modulej vejvlet-preobrazovaniya / Vseukr. mezhved. nauch.-texn. sb. ASU i pribory` avtomatiki. X.: Izd-vo XNURE`. 2011. Vy`p. 157. C. 66–77.
- Antipov O.I., Neganov V.A. Primenenie metoda normirovannogo razmaxa Xersta k analizu stoxasticheskix vremenny`x ryadov v impul`sny`x stabilizatorax napryazheniya. Fizika volnovy`x processov i radiotexnicheskie sistemy`. 2009. T. 12. № 3. S. 78–85.
- Raimundo M, Okamoto J. Application of Hurst Exponent (H) and the R/S Analysis in the Classification of FOREX Securities. Int. J. Model. Optim 2018; 8: 116–124.
- Gneiting T, Schlather M. Stochastic models that separate fractal dimension and the Hurst effect. SIAM review 2004; 46(2): 269-282.
- Maureira F. El exponente de Hurst comoparámetro de análisis de señales de EEG paracomprender la cogniciónhumana: unarevisión. Revista de PsicologíaIztacala 2020; 23(4): 1573–1591.
- Díaz H, Maureira F, Córdova F, Palominos F. Long-range linear correlation and nonlinear chaos estimation differentially characterizes functional conectivity and organization of the brain EEG. ProcediaComputerScience 2017; 122: 857–864.
- Sánchez M, Segovia T, García J. Some comments on Hurst exponent and the long memory processes on capital markets. Physica A 2008; 387, 5543–5551.
- Starchenkova K.S. and Manilo L.A. Multifractal Analysis of Heart Rate Variability for Arrhythmia Diagnostics. 2023 XXVI International Conference on Soft Computing and Measurements (SCM), Saint Petersburg, Russian Federation, 2023. R. 308–311. doi: 10.1109/SCM58628.2023.10159079.
- Tarasenko F.P. Vvedenie v kurs teorii informacii. Tomsk: TGU. 1963.
- Kantelhardt J.W. Fractal and Multifractal Time Series. 2008.
- Kublanov V.S., Borisov V.I., Dolganov A.Yu. K88 Analiz biomedicinskix signalov v srede MATLAB: Uchebnoe posobie. Ekaterinburg: Izd-vo Ural`skogo un-ta. 2016. 120 s.
- Starchenkova K.S., Manilo L.A. Starshij pokazatel` Lyapunova i e`ntropii dinamicheskoj sistemy` v zadache raspoznavaniya apnoe` sna po E`E`G. Biotexnosfera. 2021. № 1. S. 53–57. DOI 10.25960/bts.2021.1.53; Starchenkova K.S., Manilo L.A. The senior Lyapunov exponent and the entropy of a dynamic system in the problem of recognizing sleep apnea by EEG. Biotechnosfera. 2021. № 1. R. 53–57. DOI 10.25960/bts.2021.1.53
- Smbatovna S.K., Alekseevna M.L. Recognition of Sleep Apnea by EEG Using Nonlinear Dynamics Methods / 2021 Ural Symposium on Biomedical Engineering, Radioelectronics and Information Technology (USBEREIT), Yekaterinburg, Russia, 2021. R. 0009–0011. doi: 10.1109/USBEREIT51232.2021.9455089.