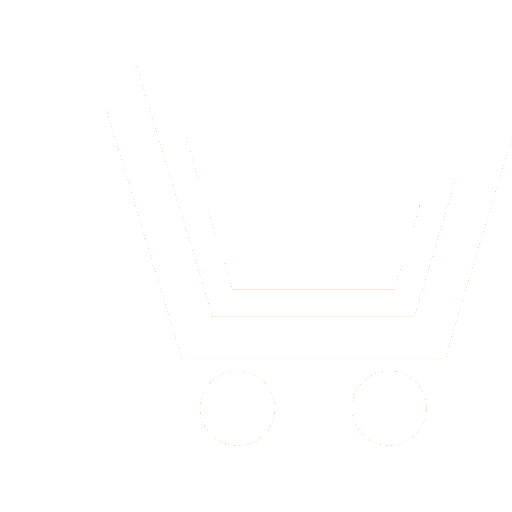
N.T. Abdullaev1, A.Sh. Khidirov2, Sh.I. Samedova3, Kh.Z. Samedova4
1,4 Azerbaijan Technical University (Baku, Azerbaijan)
2,3 Azerbaijan State University of Oil and Industry (Baku, Azerbaijan)
1 nabdullayev.46@mail.ru, 2 xidirov52@gmail.com, 3 shafaq.samedova@mail.ru, 4 hsamedova@mail.ru
Determining the boundaries of QRS complexes in ECG signals is of fundamental importance in the diagnosis of arrhythmias and in the recognition of signals in clinical manifestations in general. Description of the ECG in phase space coordinates is one of the modern methods for analyzing electrocardiographic data. The effectiveness of this method lies in the fact that it provides additional information contained in the speed characteristics of the conduction system of the heart. With various lesions of the heart, both the sequence of the path and the propagation velocity of the depolarization and repolarization wave change, the visualization of which in phase space makes it possible to detect deviations that are hidden in the analysis in the amplitude-time space. The development and software implementation of algorithms for processing and analyzing ECG signals for diagnosing arrhythmias based on modern computer technologies and innovative methods is one of the urgent tasks.
The aim of the work is to develop a system for determining the informative parameters of the ECG signal in phase space and in real time space for diagnosing ventricular extrasystoles and heart rate variability parameters.
A virtual system has been developed in the Labview software environment for processing and analyzing electrocardiographic signals in order to determine informative parameters and identify and recognize ventricular extrasystoles. The system provides for the processing of ECG signals both by classical methods and in the phase space. An algorithm for recognition of ventricular extrasystoles is proposed. The algorithm was tested, phase portraits and heart rate variability were analyzed using ECG files taken from international databases.
The developed system makes it possible to analyze the ECG signal in a complex way, evaluate informative parameters, recognize ventricular extrasystoles, and, based on the phase portrait, determine additional diagnostic features to improve the reliability of ECG diagnostics.
Abdullaev N.T., Khidirov A.Sh., Samedova Sh.I., Samedova Kh.Z. System for defining diagnostic of parameters of the ECG signal in the LabVIEW software environment. Biomedicine Radioengineering. 2024. V. 27. № 1. Р. 54-63. DOI: https://doi.org/10.18127/ j15604136-202401-07 (In Russian)
- Mironov N.Yu., Golitsyn S.P. Novyye klinicheskiye rekomendatsii Amerikanskoy assotsiatsii serdtsa. Amerikanskoy kollegii kardiologov. Obshchestva spetsialistov po narusheniyam ritma serdtsa (AHA / ACC / HRS) po lecheniyu bolnykh s zheludochkovymi aritmiyami i predotvrashcheniyu vnezapnoy serdechnoy smerti ot 2017 g. Kardiologiya. 2018. № 58(11). S. 94–100. (in Russian).
- Faynzilberg L.S. Kompyuternaya diagnostika po fazovomu portretu elektrokardiogrammy. Kiyev: «Osvita Ukrainy». 2013. 192 s. (in Russian).
- Minina E.N., Faynzilberg L.S. Fazovyy portret odnokanalnoy EKG v otsenke funktsionalnykh rezervov serdechno?sosudistoy sistemy. Vestnik novykh meditsinskikh tekhnologiy. 2014. T. 21. № 3. S. 22–27. (in Russian).
- Polosin V.G. Postroyeniye fazovykh trayektoriy v prostranstve entropiyno-parametricheskogo potentsiala dlya issledovaniya dinamicheskikh kharakteristik serdtsa. Izmereniye. Monitoring. Upravleniye. Kontrol. 2017. № 3 (21). S. 124–132. (in Russian).
- http://ecg.mit.edu/george/publications/mitdb-embs-2001.pdf
- https://physionet.org/content/edb/1.0.0/
- Clifford G.D. Signal processing methods for heart rate variability analysis. PhD Thesis michaelmas Term. 2002. 244 p.
- Rangayyan R.M. Analiz biomeditsinskikh signalov. Prakticheskiy podkhod. M.: Fizmatlit. 2007. 440 s.
- Luz E.J.S. et. al. ECG-based heartbeat classification for arrhythmia detection: A survey. Computer methods and programs in biomedicine. 2016. V. 127. R. 144–164.
- Khalaydzhi A.K., Muchnik I.B. Methods for classifying cardiac arrhythmias based on coding sequences of RR-intervals of the ECG signal. Proceedings of NSTU R.E. Alekseeva. 2021. № 1 (132). P. 38–53.
- Kadambe S., Murray R., Boudreaux-Bartels G.F. Wavelet transform-based QRS complex detector. IEEE Trans. Biomed. Eng. 1999. V. 46. P. 838–848.
- Li C., Zheng C., Tai C. Detection of ECG characteristic points using wavelet transforms. IEEE Trans. Biomed. Eng. 1995. V. 42. P. 21–28.
- Xiang et al. Automatic QRS complex detection using two-level convolutional neural network. BioMed EngOnLine https://biomedical-engineering-online.biomedcentral.com/track/pdf/10.1186/s12938-018-0441-4.pdf
- Hu Y.H., Tompkins W.J., UrrustiJ.L., Afonso V.X. Applications of artificial neuralnetworks for ECG signal detection and classification.
J. Electrocardiology. 1993. V. 26 (Suppl.). P. 66–73. - Tatarintsev P.B., Molchanova Zh.I., Radysh I.V., Ragozin O.N., Kokorina N.V. Evolyutsiya matematicheskikh metodov analiza variabelnosti serdechnogo ritma: dostizheniya i perspektivy. Tekhnologii zhivykh sistem. 2019. № 2. S. 40-50. (in Russian).
- Severin A.E., Torshin V.I., Mansur N., Berseneva I.A. Pokazateli variabelnosti serdechnogo ritma u lits iz raznykh klima-togeograficheskikh regionov pri lokalnom okhlazhdenii. Tekhnologii zhivykh sistem. 2015 №1. S. 69-71. (in Russian).
- Köhler B.-U., Hennig C., Orglmeister R. QRS detection using zero crossing counts. Applied genomics and proteomics. 2003. V. 2. № 2. P. 138–145.
- Bodin O.N., Kramm M.N., Krivonogov L.Yu., Polosin V.G., Shilov N.S. Novaya tekhnologiya podavleniya pomekh v elektrokardiosignalakh. Vestnik kibernetiki. 2017. № 4 (28). S. 122–130. (in Russian).
- Chupov A.A., Zhdanov A.E., Knyazev S.R., Rakhmatullov F.T., Rakhmatullov F.K., Dolganov A.Yu. Obrabotka signalov EKG s pomoshchyu veyvlet-analiza:diagnosticheskiye vozmozhnosti. UralRadio Engineering Journal. 2021. №5(4). S. 337–352. (in Russian).
- Leonova A.V., Ageychenko A.A. Modifikatsiya algoritma raspoznavaniya QRS kompleksov v realnom vremeni Pana-Tompkinsona. Inzhenernyy vestnik Dona. 2015. № 2. Ch. 2. http://ivdon.ru/ru/magazine/archive/n2p2y2015/3058 (in Russian).
- Khokhlov I.O., Kalinichenko A.N. Algoritm predskazaniya parametrov kardiokompleksov EKG dlya pomekhoustoychivogo obnaruzheniya R-zubtsa. Izvestiya SPbGETU «LETI». 2017. № 1. S. 72–77. (in Russian).
- Petrov S.P., Epishina E.V., Voronin V.V. Otsenka algoritmov raspoznavaniya obrazov dlya zadach avtomaticheskogo analiza elektrokardiogramm. Evraziyskiy Soyuz Uchenykh (ESU) Tekhnicheskiye nauki. 2014. T. VIII. S. 27–29. (in Russian).