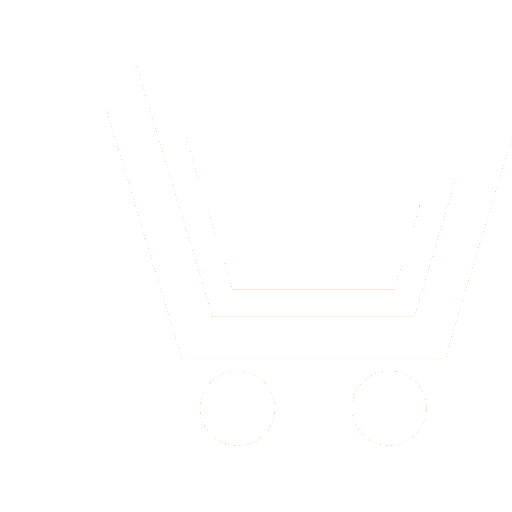
V.S. Lobanova1, D.S. Bezdetnyy2, V.V. Slizov3, E.S. Smirnova4, L.N. Anishchenko5
1–5 Bauman Moscow State Technical University (Moscow, Russia)
1 lobanova@bmstu.ru, 2 bezdetnyyyds@student.bmstu.ru, 3 v.slizov@gmail.com, 4 jine-sm@mail.ru, 5 anishchenko@rslab.ru
Changes in gait is a predictor of neurodegenerative diseases in the elderly. However, the lack of objective methods of gait analysis leads to the impossibility of early diagnosis and degradation in its quality, and the elderly are left without medical care and are at risk of falls, the second most common cause of death due to unintentional injuries. In this paper, a method for classification of gait type (normal/unstable) in the paradigm of sensor fusion (bioradars and a video surveillance camera) is proposed. This combination is due to patient comfort (both sensors are non-contact) and low cost compared to other types of sensors.
When developing the algorithm for gait recognition a data set obtained in the Remote Sensing Laboratory of Bauman Moscow State Technical University was used. During the experiments, the subject's movements were recorded by two bioradars and a video camera. Spectrograms were obtained from the bioradar signal using continuous wavelet transform. Next, fine-tuning of the AlexNet neural network was carried out. Using the BlazePose neural network, the coordinates of the key points of the skeleton were extracted from the frames of the video recordings, with the help of which a feature matrix was formed. Then it was fed to the input of a recurrent or convolutional neural network, predicting the class label. Combining information from bioradars and a video camera was carried out at the level of model predictions (a soft voting technique).
Since the combination of model predictions did not affect the quality of classification, and the video camera violates privacy and is sensitive to illumination, an appropriate technique for using the considered combination of sensors was proposed. A comparison of models for video analysis based on recurrent and convolutional architectures showed that, in terms of performance, a convolutional neural network is preferable, although the model for extracting skeleton key points makes the main contribution.
The presented results can be used in the development of a complex for the analysis of human motor activity. In the future, it is planned to evaluate the quality of gait analysis in difficult cases (poor illumination, presence of more than one person or large animals in the room).
Lobanova V.S., Bezdetnyy D.S., Slizov V.V., Smirnova E.S., Anishchenko L.N. Human gait analysis using bioradiolocation and video surveillance data in sensor fusion paradigm. Biomedicine Radioengineering. 2023. V. 26. № 5. Р. 6-16. DOI: https://doi.org/ 10.18127/j15604136-202305-02 (In Russian).
- Ageing. World Health Organization. 2023. – URL: https://www.who.int/health-topics/ageing#tab=tab_1 (data obrashcheniya 31.05.2023).
- UN Decade of Healthy Ageing. World Health Organization. 2023. – URL: https://www.who.int/initiatives/decade-of-healthy-ageing (data obrashcheniya 31.05.2023).
- Dementia. World Health Organization. 2023. – URL: https://www.who.int/news-room/fact-sheets/detail/dementia (data obrashcheniya 01.06.2023).
- Buckley C., Alcock L., McArdle R., Rehman R. Z. U., Del Din S., Mazzà C., Yarnall A.J., Rochester L. The Role of Movement Analysis in Diagnosing and Monitoring Neurodegenerative Conditions: Insights from Gait and Postural Control. Brain Sciences. 2019. V. 9. P. 34. DOI: 10.3390/brainsci9020034
- Tinetti M.E. Performance-oriented assessment of mobility problems in elderly patients. Journal of the American Geriatrics Society. 1986. V. 34. P. 119–126. DOI: 10.1111/j.1532-5415.1986.tb05480.x
- Anne S.-C., Marjorie H. Motor Control: Theory and Practical Applications. Lippincott, Williams & Wilkins: Philadelphia, PA, USA. 2000.
- Lobanova V.S., Anishchenko L.N., Slizov V.V., Smirnova E.S. Obnaruzheniye padeniy lyudey po dannym videonablyudeniya s primeneniyem metoda perenosa znaniya. Biomeditsinskaya radioelektronika. 2022. T. 25. S. 39–48. DOI: 10.18127/j15604136-202205-05 (in Russian).
- Anishchenko L.N. Glubokoye obucheniye v obnaruzhenii padeniy pri pomoshchi metoda bioradiolokatsii. Biomeditsinskaya radioelektronika. 2020. T. 23. S. 67–72. DOI: 10.18127/j15604136-202001-07 (in Russian).
- Singh A., Rehman S.U., Yongchareon S., Chong P.H.J. Sensor technologies for fall detection systems: a review. IEEE Sensors Journal. 2020. V. 20. P. 6889–6919. DOI: 10.1109/JSEN.2020.2976554
- Wang B.-H., Yu J., Wang K., Bao X.-Y., Mao K.-M. Fall detection based on dual-channel feature integration. IEEE Access. 2020. V. 16. P. 600. DOI: 10.1109/ACCESS.2020.2999503
- Gjoreski M., Gjoreski H., Lustrek M., Gams M. How accurately can your wrist device recognize daily activities and detect falls? Sensors. 2016. V. 8. P. 103443–103453. DOI: 10.3390/s16060800
- Chan H.-L., Ouyang Y., Chen R.-S., Lai Y.-H., Kuo C.-C., Liao G.-S., Hsu W.-Y., Chang Y.-J. Deep Neural Network for the Detections of Fall and Physical Activities Using Foot Pressures and Inertial Sensing. Sensors. 2023. V. 23. P. 495. DOI: 10.3390/s23010495
- He C., Liu S., Zhong G., Wu H., Cheng L., Lin J., Huang Q. A Non-Contact Fall Detection Method for Bathroom Application Based on MEMS Infrared Sensors. Micromachines. 2023. V. 14. P. 130. DOI: 10.3390/mi14010130
- Zhang C., Zu T., Hou Y., He J., Yang S., Dong R. Human activity recognition based on multi-modal fusion. CCF Transactions on Pervasive Computing and Interaction. 2023. DOI: 10.1007/s42486-023-00132-x
- Anishchenko L., Lobanova V., Slizov V. A Feasibility Study for Health and Life-Threatening Conditions Recognition via Sensor Fusion Approach. Proceedings of 2022 Ural Symposium on Biomedical Engineering, Radioelectronics and Information Technology (USBEREIT). Russia, 19–21 September 2022. P. 36–39. DOI: 10.1109/USBEREIT56278.2022.9923326
- Anishchenko L., Zhuravlev A. V., Razevig V. V., Chizh M. Lowcost Portable Bioradar System for Fall Detection. Proceedings of 2019 PhotonIcs & Electromagnetics Research Symposium — Spring (PIERS-Spring). Italy, 17–20 June 2019. P. 3566–3570. DOI: 10.1109/PIERS-Spring46901.2019.9017773
- Anishchenko L., Zhuravlev A., Chizh M. Fall Detection Using Multiple Bioradars and Convolutional Neural Networks. Sensors. 2019. V. 19. P. 5569. DOI: 10.3390/s19245569
- Krizhevsky A., Sutskever I., Hinton G.E. ImageNet classification with deep convolutional neural networks. Communications of the ACM. 2012. V. 60. P. 84–90. DOI: 10.1145/3065386
- Bazarevsky V., Grishchenko I., Raveendran K., Zhu T., Zhang F., Grundmann M. BlazePose: On device Real-time Body Pose tracking. Proceedings of CVPR Workshop on Computer Vision for Augmented and Virtual Reality. USA, June 2020. DOI: 10.48550/arXiv.2006.10204
- Rayman A. Soft Voting Algorithm Demonstration. GitHub. 2023. – URL: https://github.com/andrewrays/soft-voting-demo (data obrashcheniya 04.06.2023).
- Seifert A.K., Amin M.G., Zoubir A.M. Toward Unobtrusive In-Home Gait Analysis Based on Radar Micro-Doppler Signatures. IEEE transactions on bio-medical engineering. 2019. V. 66. P. 2629–2640. DOI: 10.1109/TBME.2019.2893528
- Viswakumar A., Rajagopalan V., Ray T., Parimi C. Human Gait Analysis Using OpenPose. Proceedings of the 2019 Fifth International Conference on Image Information Processing (ICIIP). India, 15–17 November 2019. P. 310–314. DOI: 10.1109/ICIIP47207.2019.8985781
- Stenum J., Rossi C., Roemmich R.T. Two-dimensional video-based analysis of human gait using pose estimation. PLoS Computational Biology. 2021. V. 17. P. e1008935. DOI: 10.1371/journal.pcbi.1008935
- Tsakanikas V., Ntanis A., Rigas G., Androutsos C., Boucharas D., Tachos N., Skaramagkas V., Chatzaki C., Kefalopoulou Z., Tsiknakis M., Fotiadis D. Evaluating Gait Impairment in Parkinson’s Disease from Instrumented Insole and IMU Sensor Data. Sensors. 2023. V. 23. P. 3902. DOI: 10.3390/s23083902