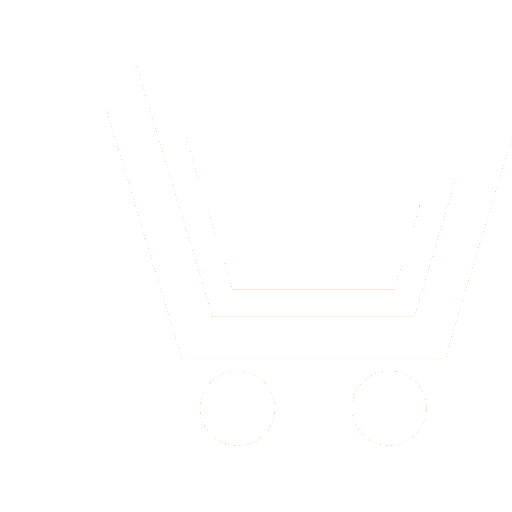
N.V. Dorofeev1, A.V. Grecheneva2
1,2 Vladimir State University named after A.G. and N.G. Stoletovs (Vladimir, Russia)
2 Russian State Agrarian University – Moscow Agricultural Academy named after K.A. Timiryazev (Moscow, Russia)
The use of mobile devices data is gaining popularity to measure human biometric. In practice, various factors affect the quality of the assessment of biometric parameters when measured using wearable devices. This feature of the measurements is associated with a random movement of the measuring part, a change in the conditions and the biometric parameters themselves of the observed. The aim of the work is to investigate the influence of various factors on the results of the assessment of gait parameters when registering gait based on the accelerometer of a mobile phone. The paper describes the main approaches used at the stage of pre-processing of accelerometric data. The paper presents the results of a study of the influence of various factors on the assessment of gait parameters using an accelerometer built into a mobile phone. A feature of the measurements is the absence of a rigid attachment of a mobile phone. . The following factors were considered as factors in the work: type of clothing, type of footwear, physiological factors. As a result of the study, it was found that the normalized form of the signal of the accelerometric sensor of a mobile phone changes by 0.3 (according to the correlation coefficient) when moving from tight-fitting pants to spacious ones, by 0.12 when changing shoes with thin soles to heels. Changes in human physiology are reflected in the accelerometer signals in the form of a change in the signal shape by 0.1 (according to the correlation coefficient).The results can be used in algorithms for compensating negative factors and in algorithms for assessing changes in individual physiological characteristics of a person Possible areas of application of the obtained results: control and security systems based on biometric gait parameters, including an authentication system, systems for medical diagnostics of human health indicators, rehabilitation systems, sports and gaming industries.
Dorofeev N.V., Grecheneva A.V. Gait variability in mobile phone accelerometer data // Biomedicine Radioengineering. 2023. V. 26. № 4. Р. 85-92. DOI: https://doi.org/10.18127/j15604136-202304-09 (In Russian)
- Moshkova A.A., Samorodov A.V., Vojnova N.A., Volkov A.K., Ershova M.V., Ivanova E.O., Fedotova E.YU. Razrabotka i issledovanie metoda avtomatizirovannogo raspoznavaniya bolezni Parkinsona na osnove kombinacii priznakov dvigatel'noj aktivnosti ruk, mimicheskoj aktivnosti i licevoj vyrazitel'nosti Biomedicinskaya radioelektronika. 2021. T. 24. № 2. S. 30–38.
- Sprager S., Juric M.B. Inertial Sensor-Based Gait Recognition: A Review. Sensors. 2015. V. 15. P. 22089–22127. doi: 10.3390/ s150922089
- Connor P., Ross A. Biometric recognition by gait: A survey of modalities and features. Comput. Vis. Image Underst. 2018. V. 167. P. 1–27.
- Guelta B., Tlemsani R., Chouraqui S., Benouis M. An Improved Behavioral Biometric System based on Gait and ECG signals. International Journal of Intelligent Engineering & Systems. 2019. V. 12. № 6. P. 147–156. doi: 10.22266/ijies2019/1231.14
- Ren Y., Chuah M.C. User Verification Leveraging Gait Recognition for Smartphone Enabled Mobile Healthcare Systems. IEEE Transactions on mobile computing. 2015. V. 14. № 9. P. 1961–1974.
- Khabir K.M., Siraj Md. S., Ahmed M., Ahmed M.U. Prediction of gender and age from inertial sensor-based gait dataset. Joint 2019 8th International Conference on Informatics, Electronics & Vision (ICIEV) & 3rd International Conference on Imaging, Vision & Pattern Recognition (IVPR). 2019. P. 371–376.
- Shema-Shiratzaky S., Beer Y., Mor A., Elbaz A. Smartphone-based inertial sensors technology – Validation of a new application to measure spatiotemporal gait metrics. Gati & Posture. 2022. V. 93. P. 102–106.
- Garufov D., Bours P. User authentication based on foot motion. SIViP. 2011. V. 5. P. 457–467. doi: 10.1007/s11760-011-0249-1
- Reyes O.C., Vera-Rodriguez R., Scully P.J., Ozanyan K.B. Analysis of Spatio-Temporal Representations for Robust Footstep Recognition with Deep Residual Neural Networks. IEEE Transaction on pattern analysis and machine intelligence. P. 1–13. DOI:10.1109/ TPAMI.2018.2799847
- Sokolova A.I., Konushin A.S. Metody identifikacii cheloveka po pohodke v video. Trudy ISP RAN. 2019. № 1. S. 1–16.
- Hel'vas A.V., Belyajkina N.G., Gilya-Zetinov A.A., CHernikova D.D., SHabunin V.M., YApryncev E.O. Raspoznavanie zhestov
s pomoshch'yu nejronnoj seti i primenenie etogo podhoda dlya sozdaniya igrovyh gadzhetov novogo pokoleniya. Trudy MFTI. 2017. № 2 (34). S. 1–8. - Teh P.S., Zhang N., Tan S.-Y., Shi Q., Khoh W.H., Nawaz R. Strengthen user authentication on mobile devices by using user’s touch dynamics pattern. Journal of Ambient Intelligence and Humanized Computing. 1 October 2020. V. 11. Is. 10. P. 4019–4039.
- Zhang X., Yao L., Huang C., Gu T., Yang Z., Liu Y. DeepKey: A Multimodal Biometric Authentication System via Deep Decoding Gaits and Brainwaves. ACM Transactions on Intelligent Systems and Technology. July 2020. V. 11. Is. 4. 3393619.
- Grecheneva A.V., Dorofeev N.V., Goryachev M.S. Estimation of human biomechanics during registration with a wearable device. Journal of Physics Conference Series. 2021. 2096(1). 012117. doi: 10.1088/1742-6596/2096/1/012117
- Lu H., Huang J., Saha T., Nachman L. Unobtrusive gait verification for mobile phones. Proceedings of the 2014 ACM International Symposium on Wearable Computers, Seattle, WA, USA. 13–17 September 2014; P. 91–98.
- Oguz A., Ertugrul O.F. Human identification based on accelerometer sensors obtained by mobile phone data. Biomedical Signal Processing and Control. 2022. V. 77. 103847. P. 1–10. doi: https://doi.org/10.1016/j.bspc.2022.103847
- Tandon R., Javid P., Giulio I.D. Mobile phone use is detrimental for gait stability in young adults. Gati & Posture. 2021. V. 88. P. 37–41.
- Pierce A., Ignasiak N.K., Eiteman-Pang W.K., Rakovsky C. Mobile phone sensors can discern medication-related gait quality changes in Parkinson’s patients in the home environment. Computer methods and programs in biomedicine update. 2021. V. 1. 100028. P. 1–8.
- Lunardini F., Malavolti M., Pedrocchi A.L.G., Borghese N.A., Ferrante S. A mobile app to transparently distinguish single-from dual-task walking for the ecological monitoring of age-related changes in daily-life gait. Gait & Posture. 2021. V. 86. P. 27–32.
- Aksenov A.YU., Klishkovskaya T.A. Osobennosti vliyaniya razlichnyh konstrukcij obuvi na nagruzku medial'noj chasti kolennogo sustava pri ispol'zovanii videoanaliza. Biomedicinskaya radioelektronika. 2016. № 8. S. 80–90.