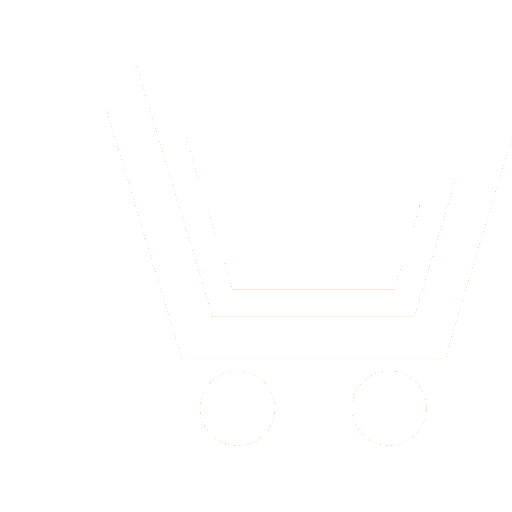
A.K. Alimuradov1, A.Yu. Tychkov2, M.I. Yuskaev3, D.S. Dudnikov4, M.A. Tyurin5, P.P. Churakov6, Z.M. Yuldashev7
1–6 Penza State University (Penza, Russia)
7 St. Petersburg State Electrotechnical University "LETI" (Saint Petersburg, Russia)
Problem statement. In practice, an accuracy of classifying human emotional states from speech has always been dependent on emotional speech database, speech signal processing algorithms, and classification methods. Numerous static and dynamic classification methods are used to recognize emotional states.
The aim of the work is to develop a method for classifying natural positive, negative and neutral human emotional states based on a five-layer recurrent neural network (RNN). The method novelty is due to various neuron activation functions employed for each network layer stemming from the peculiarities of natural emotional speech informative parameters used as input data for the neural network.
Results. Local and global informative speech parameters relevant to human emotional states are outlined, and the known classification methods are surveyed. The proposed classification method is described, and the developed Russian database of natural emotional speech is presented.
Practical significance. The research results compared with the widely used classification methods have evidenced 95% accuracy of the developed method, which can be effectively tested in systems for detecting and classifying human natural emotional states from speech.
Alimuradov A.K., Tychkov A.Yu., Yuskaev M.I., Dudnikov D.S., Tyurin M.A., Churakov P.P., Yuldashev Z.M. RNN-based method to classify human natural emotional states from speech // Biomedicine Radioengineering. 2023. V. 26. № 4. Р. 73-84. DOI: https://doi.org/10.18127/j15604136-202304-08 (In Russian)
- L.R. Rabiner, R.W. Schafer. Digital Processing of Speech Signals. London (UK): Pearson Education, 1978.
- Ververidis D., Kotropoulos C., Pitas I. Automatic emotional speech classification. 2004 IEEE international conference on acoustics, speech, and signal processing. IEEE, 2004. Т. 1. P. 593.
- Hu H., Xu M. X., Wu W. Fusion of global statistical and segmental spectral features for speech emotion recognition. INTERSPEECH. 2007. P. 2269–2272.
- Shami M. T., Kamel M. S. Segment-based approach to the recognition of emotions in speech. 2005 IEEE international conference on multimedia and expo. IEEE, 2005. P. 4.
- Picard R. W., Vyzas E., Healey J. Toward machine emotional intelligence: Analysis of affective physiological state. IEEE transactions on pattern analysis and machine intelligence. 2001. Т. 23. №. 10. P. 1175–1191.
- El Ayadi M., Kamel M. S., Karray F. Survey on speech emotion recognition: Features, classification schemes, and databases. Pattern recognition. 2011. Т. 44. №. 3. P. 572–587.
- Le D., Provost E. M. Emotion recognition from spontaneous speech using hidden markov models with deep belief networks. 2013 IEEE Workshop on Automatic Speech Recognition and Understanding. IEEE, 2013. P. 216-221.
- Vlasenko B., Wendemuth A. Tuning hidden Markov model for speech emotion recognition. Fortschritte der akustik. 2007. Т. 33. №. 1. P. 317.
- Muthusamy H., Polat K., Yaacob S. Improved emotion recognition using gaussian mixture model and extreme learning machine in speech and glottal signals. Mathematical Problems in Engineering. 2015. Т. 2015.
- Patel P., Chaudhari A.A., Pund M.A., Deshmukh D.H. Speech emotion recognition system using gaussian mixture model and improvement proposed via boosted GMM. International Conference on Science & Engineering for Sustainable Development, September 18–20. 2017. New York, USA. P. 56–64.
- Chavhan Y., Dhore M. L., Yesaware P. Speech emotion recognition using support vector machine. International Journal of Computer Applications. 2010. Т. 1. №. 20. P. 6–9.
- Chaudhary R., Saraswat S., Chaturvedi S., Naregalkar P. Speech Emotion Recognition Using Neural Network. International Journal of Scientific Research in Engineering and Management. 2020. №. 4(8) P. 5.
- Lu C. et al. Speech Emotion Recognition via an Attentive Time-Frequency Neural Network. IEEE Transactions on Computational Social Systems. 2022. №. 9. P. 1–10.
- Singh A., Srivastava K.K., Murugan H. Speech Emotion Recognition Using CNN. International Journal of Psychosocial Rehabilitation. 2020. №. 24(8). P. 2408–2416.
- Schuller B., Rigoll G., Lang M. Speech emotion recognition combining acoustic features and linguistic information in a hybrid support vector machine-belief network architecture. 2004 IEEE international conference on acoustics, speech, and signal processing. IEEE. 2004. Т. 1. P. 577–580.
- Razak A. A. et al. Comparison between fuzzy and nn method for speech emotion recognition. Third International Conference on Information Technology and Applications (ICITA'05). IEEE. 2005. Т. 1. P. 297–302.
- Pierre-Yves O. The production and recognition of emotions in speech: features and algorithms. International Journal of Human-Computer Studies. 2003. Т. 59. №. 1-2. P. 157–183.
- Alimuradov A. K. et al. Development of natural emotional speech database for training automatic recognition systems of stressful emotions in human-robot interaction. 2020 4th Scientific School on Dynamics of Complex Networks and their Application in Intellectual Robotics (DCNAIR). IEEE. 2020. P. 11–16.
- Alimuradov A.K. et al. Development of natural emotional speech database for training automatic recognition systems of stressful emotions in human–computer interaction. 2020 XVI International Conference on New Information Technologies and Systems. November 18–19. 2020. Penza. Russia. P. 115–121.
- Audacity Free, Open Source, Cross-Platform Audio Software. Available: https://www.audacityteam.org (Date of application February 5, 2023).