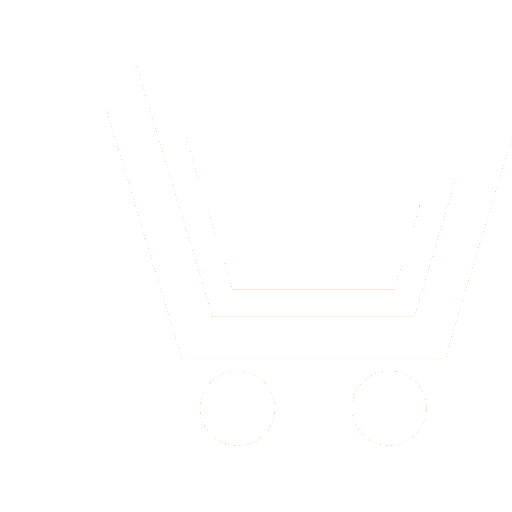
Yu.A. Chelebaeva1, S.V. Chelebaev2
1,2 Ryazan State Radio Engineering University named after V.F. Utkina (Ryazan, Russia)
It is necessary to perform operations of converting analog signals into their digital equivalent (code) when developing measuring systems for medical purposes, which include sensors of analog signals, in which such parameters as frequency, duration, phase, voltage or current are carriers of primary information. The task of designing frequency-time parameter converters of biosignals into a digital code therefore arises. One of the promising approaches to solving the problem is the construction of converters using neural network technologies. To build a converter characterized by low hardware costs for its implementation, a two-layer neural network with one layer of trained weight coefficients was chosen.
Purpose – development of a model for converting frequency-time parameters of biosignals into code based on a neural network with one layer of trained weight coefficients, development of a technique for adjustment a network of the converter.
The use of a 2-layer neural network for converting frequency-time parameters of biosignals into a code with one layer of trained weight coefficients is proposed.
A modification of the delta-rule algorithm, taking into account the training of one layer of weight coefficients in a two-layer direct distribution network, is considered.
The method of adjusting the neural network converter of frequency-time parameters of biosignals into a code consisting of 2 stages of network training has been developed. To reduce the reproduction error of nonlinear functional dependencies, multi-stream learning is used.
The results of the converter network training were analyzed. He says that such a network achieves 0.1% accuracy in reproducing wide functional dependencies, which is sufficient for most biosignal processing tasks.
The obtained results make it possible to conclude that the proposed structure of the biosignal time-frequency parameter converter to a digital code has a low error of reproduction of a nonlinear dependence, is characterized by simple training of a neural network, and can be implemented on programmable logic with insignificant hardware costs.
Chelebaeva Yu.A., Chelebaev S.V. Converters of frequency-time parameters of biosignals into code based on neural network with one layer of trained weight coefficients. Biomedicine Radioengineering. 2023. V. 26. № 3. Р. 90-98. DOI: https://doi.org/10.18127/ j15604136-202303-11 (In Russian).
- Galushkin A.I. Neyronnyye seti: osnovy teorii. M.: Goryachaya liniya – Telekom. 2010. 496 s. (in Russian).
- Golovko V.A. Neyronnyye seti: obucheniye. organizatsiya i primeneniye. M.: IPRZhR. 2001. 256 s. (in Russian).
- Loktyukhin V.N., Chelebayev S.V. Neyrosetevyye preobrazovateli impulsno-analogovoy informatsii: organizatsiya. sintez. realizatsiya. Pod obshch. red. A.I. Galushkina. M.: Goryachaya liniya – Telekom. 2008. 144 s. (in Russian).
- Chelebayev S.V., Chelebayeva Yu.A. Preobrazovateli chastotno-vremennykh parametrov biosignalov v kod dvukh peremennykh na osnove primeneniya mnogosloynykh perseptronov. Biomeditsinskaya radioelektronika. 2019. T. 22. № 4. S. 60–66. (in Russian).
- Melnik O.V., Chelebayev S.V., Chelebayeva Yu.A. Analiz kardioritmogrammy v neyrosetevom bazise operatsiy. Biomeditsinskaya radioelektronika. 2018. № 8. S. 39–44. (in Russian).