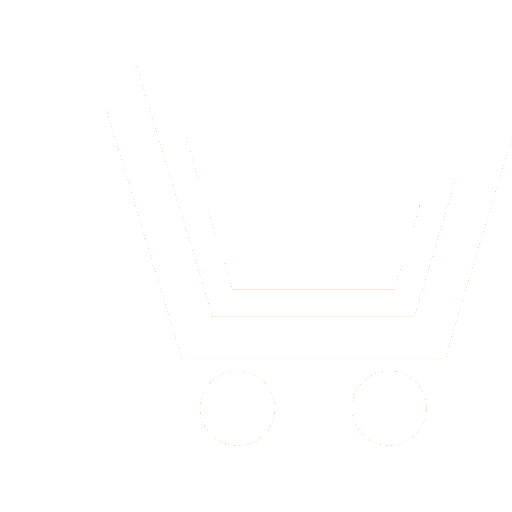
A.V. Samorodov1
1 Bauman Moscow State Technical University (Moscow, Russia)
The development of automated methods for preparing and analyzing of cytological preparations for solving the problems of oncocytology requires the formation of quantitative criteria for the quality of the analyzed preparations, the main of which is the number of cells being analyzed. At present, modeling and experimental study of the correlation between the informativity of the cytological conclusion and the sample size of the cells has been carried out only for a limited range of tasks; their results are not directly applicable to the problems of breast cancer diagnosing.
The purpose of the work is to develop a model of the correlation between the indicators of the informativity of the cytological conclusion in the tasks of breast cancer diagnosing and the sample size of the cells being analyzed.
For the condition of simple random sampling and making a diagnostic decision based on the cells formula, a model was built that provides an estimate of the sample size, taking into account the given values of diagnostic sensitivity (DSe) and diagnostic specificity (DSp), errors of individual cells classification and biological variation.
The application of the model was demonstrated by the examples of immunocytochemical estimation of estrogen receptors and breast cancer degree of differentiation estimation based on cells classification on previously estimated 6 nuclear morphofunctional types.
Dependence of sample size of the cells on equal error rate (EER) of their binary classification (stained with DAB stain / not stained) was calculated for the task of differentiation between relative concentrations of DAB-stained cells of zero and 0,01, which corresponds to Allred scores PS = 0 and PS = 1 differentiation. For DSe and DSp 95 % each and zero EER, sample size equals 268. If EER of binary cells classification is 0,05, sample size grows up to 6872 cells. For DSe and DSp 97,5 % each, the same conditions leads to 381 and 9757 cells respectively.
The presence of biological variation leads to a significant increase in the requirements for the number of analyzed cells. For example, coefficient of variation 50 % leads to the increase in the sample size of cells to more than 85 thousand for EER 0,05 and DSe and DSp 95 % each, and to more than 120 thousand for DSe and DSp 97,5 % each.
The same calculations were made for the task of breast cancer degree of differentiation estimation on the base of pairwise classifications: well- and poorly differentiated, well- and moderately differentiated, poorly and moderately differentiated breast cancer. EER of 6-class cells classification 0,05 and multivariate coefficient of biological variation (Voinov and Nikulin’s coefficient) 20 % leads to sample size of approximately a hundred cells to obtain the probability of correct pairwise classification between 90 and 95 %.
In general, the model derived generalizes a number of previously published results and opens up the possibility of formalizing the requirements for the quality of cytological preparations. It provides the calculation of the informativity indicators of the analysis results taking into account the diagnostic problem being solved and the parameters of image processing algorithms, and, in general, the scientifically based formation of requirements for the automation of cytological analysis in oncology. Of course, further research should be carried out regarding the applicability of the assumptions made, manly, the assumption of simple random sampling.
Samorodov A.V. Evaluation of requirements for the sample size in the tasks of cytological diagnosis of breast cancer. Biomedicine Radio-engineering. 2023. V. 26. № 1. Р. 27-34. DOI: https://doi.org/10.18127/j15604136-202301-03 (In Russian).
- Volchenko N.N., Slavnova E.N., Gladunova Z.D. i dr. Sovremennaya tsitologicheskaya diagnostika zabolevaniy molochnoy zhelezy. pod red. N.N. Volchenko. E.N. Slavnovoy. M.: Izd-vo MGTU im. N.E. Baumana. 2014. 199 s. (in Russian).
- Field A.S. et al. The International Academy of Cytology Yokohama system for reporting breast fine needle aspiration biopsy cytopathology. Acta Cytologica. 2019. V. 63. P. 257–273. DOI: 10.1159/000499509
- Wilbur D.C., Bibbo M. Automation in Cervical Cytology. in Comprehensive Cytopathology (Third Edition). Elsevier Inc. 2008. P. 1021–1042. DOI: 10.1016/B978-141604208-2.10034-X
- Samorodov A.V. Otsenka kachestva tsitologicheskikh preparatov po ikh izobrazheniyam. Biomeditsinskaya radioelektronika. 2008. № 10. S. 39–45. (in Russian).
- Gomez-Macias G.S., Garza-Guajardo R., Segura-Luna J., Barboza-Quintana O. Inadequte fine needle aspiration biopsy samples: Pathologists versus other specialists. CytoJournal. 2009. V. 6. Iss. 1. P. 1–9. DOI: 10.4103/1742-6413.52831
- Kitchener H.C. et al. A study of cellular counting to determine minimum thresholds for adequacy for liquid-based cervical cytology using a survey and counting protocol. Health Technology Assessment. 2015. V. 19. Iss. 22. P. 1–63. DOI: 10.3310/hta19220
- Castleman K.R., White B.S. The tradeoff of cell classifier error rates. Cytometry. 1980. V. 1. № 2. P. 156–160. DOI: 10.1002/cyto.990010211
- White B.S., Castleman K.R. Estimating cell populations. Pattern Recognition. 1981. V. 13. № 5. P. 365–370. DOI: 10.1016/0031-3203(81)90092-3
- Timmers T., Gelsema E.S. A model for the classification of specimens containing random proportions of abnormal cells. Cytometry. 1985. V. 6. P. 22–25. DOI: 10.1002/CYTO.990060105
- Castleman K.R., Price K.H., White B.S. Effect of random abnormal cell proportion on specimen classifier performance. Cytometry. 1993. V. 14. P. 1–8. DOI: 10.1002/cyto.990140103
- Samorodov A.V. Avtomatizirovannyy morfologicheskiy analiz tsitologicheskikh preparatov. Biomeditsinskaya radioelektronika. 2009. № 10. S. 35–40. (in Russian).
- Volchenko N.N. i dr. Kompyuternyy analiz tekstury izobrazheniy yader v opredelenii stepeni differentsirovki invazivnogo protokovogo raka molochnoy zhelezy. Rossiyskiy onkologicheskiy zhurnal. 2008. № 1. S. 13–18. (in Russian).
- Dobrolyubova D.A. et al. Automatic image analysis algorithm for quantitative assessment of breast cancer estrogen receptor status in immunocyto-chemistry. Pattern Recognition and Image Analysis. 2016. V. 26. № 3. P. 552–557. DOI: 10.1134/S1054661816030032
- Samorodov A.V. Biotechnical systems for automated microscopy of cytology specimens. Biomedical Engineering. 2019. V. 52. № 6. P. 387–390. DOI: 10.1007/s10527-019-09853-9
- Brouckaert O. et al. A critical review why assessment of steroid hormone receptors in breast cancer should be quantitative. Annals of Oncology. 2013. V. 24. Iss. 1. P. 47–53. DOI: 10.1093/annonc/mds238
- Aziz N. et al. Biological variation of immunological blood biomarkers in healthy individuals and quality goals for biomarker tests. BMC Immunology. 2019. V. 20. Article № 33. DOI: 10.1186/s12865-019-0313-0
- Duvall E. ABC3 and LBC – adequate or not?. Cytopathology. 2013. V. 24. P. 211–215. DOI: 10.1111/cyt.12081