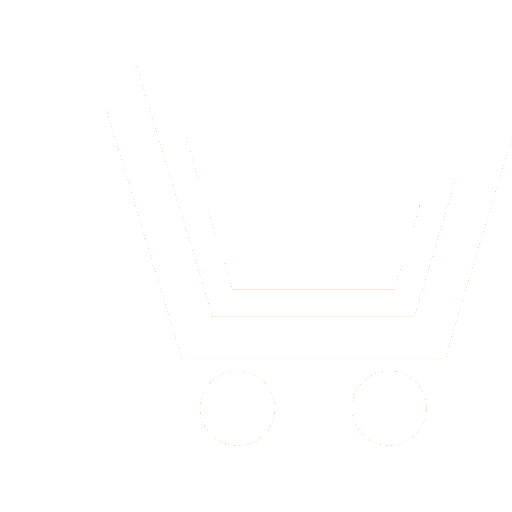
D.S. Makhov1, G.S. Razmakhaev2, E.N. Slavnova3, A.V. Samorodov4
1,4 Bauman Moscow State Technical University (Moscow, Russia)
2,3 Oncocytology department, P.A. Hertzen Moscow Oncology Research Institute (Moscow, Russia)
Breast cancer (BC) is one of the most common diseases in women. Accurate treatment tactics could be provided by determining BC subtype according to molecular and genetics classification. About all of the BC cases are HER2-positive. These subtypes are more aggressive and reoccurrence probability is the highest among all BC subtypes. Recently target treatment with trastuzumab (Herceptin) was discovered which highly increases survival probability. HER2 determination is held using immunochemical study according to ASCO/CAP recommendations and guidelines. In equivocal cases the HER2 gene amplification determination is carried out via fluorescent in situ hybridization (FISH). In both immunochemical and FISH studies pathologist manually investigates the sample which is a laborious and time-consuming process. Thus, the article presents the designing and testing results of the biotechnical system for automated HER2 gene amplification determination in breast cancer by fluorescent in situ hybridization. In cooperation with P. Hertsen Moscow Oncology Research Institute a verified image database of 158 images was collected for 44 patients (15 with amplification, 29 without amplification). The images were captured using Zeiss Axio Imager M1 microscope with CHROMA SP100V2 (DAPI), CHROMA MF101 (FITC), CHROMA SP103V1 (TexasRed) fluorescent filter cubes and Metasystems CoolCube1 camera. The capturing process was controlled by the Metasys Metafer software. Due to the software limitations the registered images had size of 990 by 878 pixels. Nuclei and fluorescent signals were manually segmented on images for 7 patients (4 with amplification and 3 without amplification) for further investigation. The U-net based neural network was trained on a subset of labeled fluorescent images to segment the nuclei and determine signal regions. For automated medical decision support in determining HER2 status the ratio average red-to-green signals ratio was calculated by the additional software. In our previous work we showed that applying background correction improves foreground contrast and increases the quality of segmentation after retraining the neural network. In this study we verified diagnostic sensitivity and specificity on a test dataset of 37 patients. Diagnostic sensitivity and specificity estimates were 0.70, 0.63 and 0.60, 0.72 without and with background correction, respectively. The causes of such low results are high variation in biological tissue and not fully standardized sample preparation protocol. Moreover, in current software version there is no special cluster processing, so the final statistics based on average signal counts could failure. After visual inspection of the segmented images by the neural network we can conclude that criteria for background correction application should be constructed prior to segmentation. This adaptation should increase the quality of nuclei segmentation and signal detection, thus improving HER2 gene amplification automated determination.
Makhov D.S., Razmakhaev G.S., Slavnova E.N., Samorodov A.V. Biotechnical system for automated HER2-status determination in breast cancer via fluorescent in situ hybridization (FISH). Biomedicine Radioengineering. 2022. V. 25. № 5. Р. 58-69. DOI: https://doi.org/10.18127/j15604136-202205-07 (In Russian)
- Bray М.F. et al. Global cancer statistics 2018: GLOBOCAN estimates of incidence and mortality worldwide for 36 cancers in 185 countries. CA: a cancer journal for clinicians. 2018. V. 68. № 6. P. 394–424.
- Malhotra G.K. et al. Histological, molecular and functional subtypes of breast cancers. Cancer biology & therapy. 2010. V. 10. № 10.
P. 955–960. - Sovremennaya tsitologicheskaya diagnostika zabolevaniy molochnoy zhelezy: monografiya. N.N. Volchenko, E.N. Slavnova, Z.D. Gladunova i dr.. Pod red. E.N. Slavnovoy. M.: MGTU im. N.E. Baumana. 2014. 198 s. (in Russian).
- Wolff A.C. et al. Human Epidermal Growth Factor Receptor 2 Testing in Breast Cancer: American Society of Clinical Oncology/College of American Pathologists Clinical Practice Guideline Focused Update. Journal of Clinical Oncology. 2018. V. 36. № 20. P. 2105–2122.
- Jiang H. et al. Evaluation of HER2 gene amplification in breast cancer using nuclei microarray in situ hybridization //International Journal of Molecular Sciences. 2012. V. 13. № 5. P. 5519–5527.
- Smoley S.A. et al. Standardization of fluorescence in situ hybridization studies on chronic lymphocytic leukemia (CLL) blood and marrow cells by the CLL Research Consortium. Cancer genetics and cytogenetics. 2010. V. 203. № 2. P. 141–148.
- Bogdanovska-Todorovska M. et al. Standardization and optimization of fluorescence in situ hybridization (FISH) for HER-2 assessment in breast cancer: A single center experience. Bosnian journal of basic medical sciences. 2018. V. 18. № 2. P. 132.
- Petersen B.L. et al. Fluorescence in situ hybridization on formalin-fixed and paraffin-embedded tissue: optimizing the method. Applied Immunohistochemistry & Molecular Morphology. 2004. V. 12. № 3. P. 259–265.
- Souza M.M., Urdampilleta J.D., Forni Martins E.R. Improvements in cytological preparations for fluorescent in situ hybridization in Passiflora. Genetics and Molecular Research. 2010. V. 9. № 4. P. 2148–2155.
- Castleman K.R. Color compensation for digitized FISH images. Bioimaging. 1993. V. 1. № 3. P. 159–165.
- Choi H., Castleman K.R., Bovik A.C. Joint segmentation and classification of M-FISH chromosome images. The 26th Annual International Conference of the IEEE Engineering in Medicine and Biology Society. IEEE. 2004. V. 1. P. 1636–1639.
- Likar B. et al. Retrospective shading correction based on entropy minimization. Journal of Microscopy. 2000. V. 197. № Pt 3. P. 285–295.
- Choi H., Castleman K.R., Bovik A.C. Color compensation of multicolor FISH images. IEEE transactions on medical imaging. 2008. V. 28. № 1. P. 129–136.
- Tomaževič D., Likar B., Pernuš F. Comparative evaluation of retrospective shading correction methods //Journal of microscopy. 2002. V. 208. № 3. P. 212–223.
- Bagwell C.B., Adams E.G. Fluorescence spectral overlap compensation for any number of flow cytometry parameters. Annals of the New York Academy of Sciences. 1993. V. 677. № 1. P. 167–184.
- Ronneberger O., Fischer P., Brox T. U-net: Convolutional networks for biomedical image segmentation. International Conference on Medical image computing and computer-assisted intervention. Springer, Cham. 2015. P. 234–241.
- Makhov D.S., Samorodov A.V., Razmakhayev G.S., Slavnova E.N. Korrektsiya fona dlya avtomatizatsii analiza FISH-izobrazheniy. Biotekhnosfera. 2021. № 1 (66). P. 23–29. (in Russian).