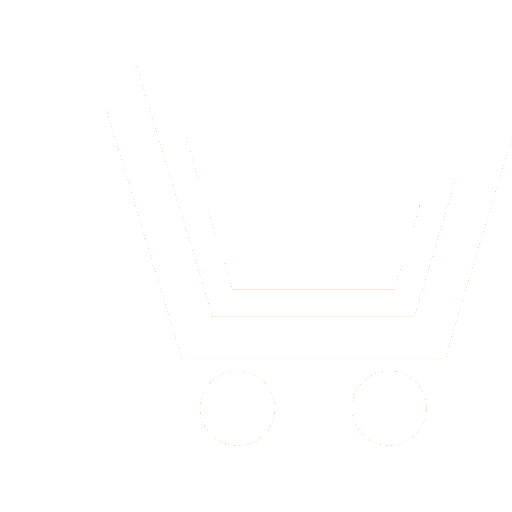
V.N. Gridin1, N.N. Koberskaya2, B.R. Salem3, D.S. Smirnov4, V.I. Solodovnikov5, N.N. Yakhno6
1–6 Design Information Technologies Center Russian Academy of Sciences (Odintsovo, Moscow Region, Russia)
The article is devoted to the problem of detecting the initial stages of cognitive decline, which at the current level can be carried out only by analyzing a large number of neuropsychological tests, which complicates the study of these processes, due to the high laboriousness of this tests implementation. The earliest forms of cognitive decline attract the greatest attention of researchers, when the most effective methods of therapy and prevention of an increase in cognitive decline up to the development of dementia and other cognitive disorders. The proposed methodology allows to reduce the number of screening tests, while retaining information that is important for assessing the degree of cognitive decline, as well as to be used for information systems for analyzing data from the examination of patients with subjective and mild cognitive impairments.
Purpose of the work – to investigate methods for selecting the best combinations of neuropsychological tests obtained using statistical analysis and machine learning methods, as well as algorithms for calculating the integral indicator and a classifier based on it for the distribution of patients into groups of pre-moderate cognitive decline
We analyzed the data obtained as a result of neuropsychological examination of patients presenting with cognitive complaints, the purpose of which was to identify the most significant key combinations of neuropsychological tests, as well as to develop algorithms for calculating the integral indicator of the degree of cognitive decline and a classifier based on it. Several neuropsychological tests combinations were selected. Notably, that with different methods of selection, we’ve got agreed results. A method is proposed for calculating the integral indicator of the degree of cognitive decline based on the selected most informative features, using unconditional optimization by the Nelder-Mead deformable polyhedron method. It is shown that the use of machine learning, in particular, gradient boosting, made it possible to improve the result obtained by methods of statistical analysis. An additional advantage of the proposed approach is the ability to conveniently interpret and visualize the dynamics of the patient's cognitive status in the form of a graph over time.
The practical significance of the work lies in the field of development new mathematical approaches that can form the basis of tools for statistical processing of medical data and an integral assessment of the degree of cognitive decline, as well as predictive models of the dynamics of this decline.
Gridin V.N., Koberskaya N.N., Salem B.R., Smirnov D.S., Solodovnikov V.I., Yakhno N.N. Mathematical approaches to the development of an integral indicator of the degree of cognitive decline. Biomedicine Radioengineering. 2021. V. 24. № 6. Р. 46−57. DOI: https://doi.org/10.18127/j15604136-202106-05 (In Russian)
- Yakhno N.N., Zakharov V.V. Sindrom umerennykh kognitivnykh rasstroystv v pozhilom vozraste: diagnostika i lecheniye.. Russkiy meditsinskiy zhurnal. 2004. T. 12(10). S. 573–576. (in Russian).
- Reisberg B., Gauthier S. Current evidence for subjective cognitive impairment (SCI) as the pre-mild cognitive impairment (MCI) stage of subsequently manifest Alzheimer’s disease. Int. Psychogeriatr. 2008. V. 20. P. 1–16.
- Lokshina A.B., Zakharov V.V. Legkiye i umerennyye kognitivnyye rasstroystva pri distsirkulyatornoy entsefalopatii. Nevrologicheskiy zhurnal. 2006. T. 11. Prilozheniye № 1. S. 57–64. (in Russian).
- Koberskaya N.N., Ostroumova T.M., Perepelov V.A., Smirnov D.S. Vliyaniye geneticheskikh. komorbidnykh emotsionalno-affektivnykh faktorov na doumerennoye kognitivnoye snizheniye u patsiyentov srednego vozrasta. Neyropsikhologiya i neyrosomatika. 2021. T. 13. № 4. S. 66–74. (in Russian).
- Yakhno N.N., Gridin V.N., Smirnov D.S., Panishchev V.S., Parfenov V.A., Ostroumova T.M., Koberskaya N.N. Statisticheskaya obrabotka i metodika sokrashcheniya razmernosti prostranstva dannykh patsiyentov pri analize kognitivnykh narusheniy// Informatsionnyye tekhnologii. 2020. T. 26. № 9. S. 515–522. (in Russian).
- Yakhno N.N., Gridin V.N., Koberskaya N.N., Smirnov D.S., Solodovnikov V.I. Algoritm filtratsii i poiska zavisimostey pri diagnostike patsiyentov s doumerennym kognitivnym snizheniyem. Informatsionnyye tekhnologii i vychislitelnyye sistemy. 2021. № 2. S. 34–43. DOI: 10.14357/20718632210204 (in Russian).
- Nelder J.A., Mead R. A simplex algorithm for function minimization. Computer Journal. 1965. V. 7. P. 308–313. DOI: 10.1093/comjnl/7.4.308
- Buckland M., Gey F. The relationship between Recall and Precision. Journal of the American Society for Information Science. 1994. V. 45(1). P. 12–19.
- Powers D. Evaluation: From Precision, Recall and F Factor to ROC, Informedness, Markedness and Correlation. Technical Report SIE07-001, Flinders University. 2007.
- Friedman J.H. Greedy function approximation: a gradient boosting machine. Annals of Statistics. 2001. P. 1189–1232.
- Cameron A.C., Windmeijer F.A.G. An R-squared measure of goodness of fit for some common nonlinear regression models. Journal of Econometrics. 1997. V. 77. № 2. P. 329–342. https://doi.org/10.1016/s0304-4076(96)01818-0
- Botchkarev A. A New Typology Design of Performance Met-rics to Measure Errors in Machine Learning Regression Algorithms. Interdisciplinary Journal of Information, Knowledge, and Management. 2019. V. 14. P. 045–076. http://dx.doi.org/10.28945/4184
- Bergstra J., Bengio Y. Random search for hyper-parameter optimization. J. Mach. Learn. Res. 2012. V. 13. null (3/1/2012). P. 281–305. 14. Refaeilzadeh P., Tang L., Liu H. Cross-Validation. Encyclopedia of Database Systems. Boston, MA: Springer US, 2009. P. 532–538. https://doi.org/10.1007/978-0-387-39940-9_565