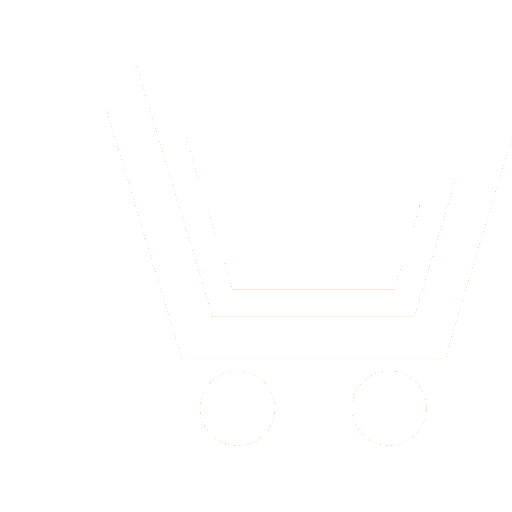
N.Haddad1, M.V. Derkach2, A.N. Dmitriev3, I.K. Sergeev4, S.I. Shchukin5
1–5 Bauman Moscow State Technical University (Moscow, Russia)
Brain-computer interface is a promising technology that gives humans with a motor disease in some particular cases the ability to directly control computers or any external devices using only brain signals such as EEG. Among a variety of EEG patterns that were used to design EEG-based BCI, P300 is considered as one of the most common options for fine motor rehabilitation. Efficient P300 detection is essential due to its crucial role in evaluating the accuracy and reliability of brain-computer interfaces, in the last few years, Machine learning methods have been widely used as classifiers for P300. Where the quality of the classification using these methods significantly depends on the input features.
This work aims to study the parameters of visual stimulation, as well as the characteristics of the P300, which would lead to an increase in the accuracy of the target stimulus detection for the P300-BCI system.
Correlation analysis between target – non-target stimuli and wavelet Morlet has been done after splitting the data according to the stimulation frequency for comparisons, taking into account the stimulation parameters that were used in each experiment to find out which stimulation parameters are more suitable for brain-computer interface based on P300. A comparative analysis of various features of the P300 using artificial neural networks has been considered to achieve more analytical and consistent conclusions. The use of ANN in our work has been decided after considering many other methods like support vector machine, linear discriminant analysis, and Random forest. A significant improvement in the classification accuracy was achieved using the correlation function as a P300 feature. It was found that the wavelet parameters should be selected individually for each participant. However, no direct relationship was found between the wavelet parameters and the stimulation frequency.
Taking into account the individual parameters of the wavelet Morlet for each patient, and using the correlation function as a P300 feature, allows achieving a significant increase in the classification accuracy of the target stimulus. In addition to the importance of choosing the most appropriate paradigm during the experiment, which would increase the quality of data and thus improve BCI performance.
Haddad N., Derkach M.V., Dmitriev A.N., Sergeev I.K., Shchukin S.I. Selection of P300 signal features based on machine learning and the Morlet wavelet use for BCI applications. Biomedicine Radioengineering. 2021. V. 24. № 5. P. 17–29. DOI: 10.18127/j15604136-202105-03 (in Russian)
- Briko A.N., Kobelev A.V., Shchukin S.I. Electrodes interchangeability during electromyogram and bioimpedance joint recording. IEEE. 2018. P. 17–20.
- Nicolas-Alonso L.F., Gomez-Gil J. Brain computer interfaces, a review. Sensors. 2012. № 2 (12). P. 1211–1279.
- Brouwer A.-M., Erp J.B.F. Van A tactile P300 brain-computer interface. Frontiers in neuroscience. 2010. (4). P. 19.
- Ganin I.P., Kim S.A., Liburkina S.P., Galkina N.V., Luzhin A.O., Majorova L.A., Malyukova N.G., SHklovskij V.M., Kaplan A.Ya. Nabor teksta pacientami s postinsul'tnoj afaziej v komplekse «Nejrochat» na osnove tekhnologii interfejsov mozg-komp'yuter na volne P300 // Zhurnal vysshej nervnoj deyatel'nosti im. I.P. Pavlova. 2020. № 4 (70). S. 435–445 (In Russian).
- Dmitriev A.N, Al-harosh M., Sergeev I.K., Nicolaev A. The optimal stimulation mode and the number of averaging epochs selection for P300 detection. 2018 Ural Symposium on Biomedical Engineering, Radioelectronics and Information Technology (USBEREIT). 2018. P. 91–94.
- Bulanov V.A., Zakharov A.V, Khivintseva E.V. Wavelet transform for the identification of P300. IOP Publishing, 2020. P. 52049.
- Manyakov N.V., Chumerin N., Combaz A., Van Hulle M.M. Comparison of classification methods for P300 brain-computer interface on disabled subjects. Computational intelligence and neuroscience. 2011. (2011). P. 519868.
- Gnezdickij V.V. Vyzvannye potencialy mozga v klinicheskoj praktike. Taganrog: TRTU, 1997. 252 s. (In Russian).
- Hanley J.A., McNeil B.J. The meaning and use of the area under a receiver operating characteristic (ROC) curve. Radiology. 1982. № 1 (143). P. 29–36.
- Dmitriev A.N., Kotin V.V. Time series prediction of morbidity using artificial neural networks. Biomedical Engineering. 2013. № 1 (47). P. 43–45.
- Dmitriev A.N., Kurganova A.V., Sergeev I.K., Shchukin S.I. Primenenie algoritmov udaleniya artefakta morganiya pri detekcii P300 dlya zadach reabilitacii pacientov s parezami: pilotnoe issledovanie na zdorovyh ispytuemyh. Biomedicinskaya radioelektronika. 2018. № 10. S. 15–24 (In Russian).
- Rice L., Wong E., Kolter Z. Overfitting in adversarially robust deep learning. International Conference on Machine Learning. PMLR. 2020. P. 8093–8104.