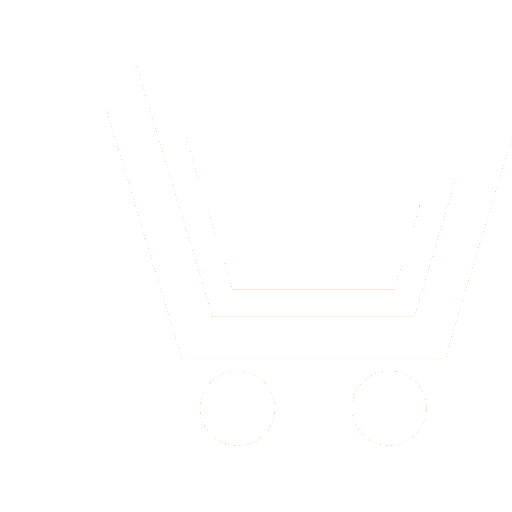
A.A. Moshkova1, A.V. Samorodov2, N.A. Voinova3, A.K. Volkov4, M.V. Ershova5, E.O. Ivanova6, E.Y. Fedotova7
1,2 Bauman Moscow State Technical University (Moscow, Russia)
3,4 Scientific and Educational Medical-Technological Center of Bauman Moscow State Technical University (Moscow, Russia)
5–7 Research Center of Neurology, 5th neurological department (Moscow, Russia)
Currently, researchers have noted an increase in the life expectancy of the population around the world, which will undoubtedly cause an increase in the incidence of Parkinson's disease (PD) in the next decade. The currently used methods of visual assessment of the severity of the disease based on rating scales, as many studies show, are not objective and unsuitable for screening. It is known that PD patients do not consult a neurologist in a timely manner, which significantly reduces the effectiveness of therapy. The relevance of creating an automated PD recognition system, first of all, during screening, is confirmed by the need to objectify the assessment, as well as the possibility of its widespread use, including home use. Thus, this work is devoted to the development and study of a method for automated recognition of Parkinson's disease based on binary classification. To solve the problem of binary classification, features of hypokinesia are used, which is the most important symptom for the diagnosis of Parkinson's disease. The calculation of the features of hypokinesia is carried out on the basis of 3 motor tasks including the task of motor activity of the hands assessment and two tasks for the assessment of hypomimia: mimic activity and facial expressiveness assessment. The motor activity of the hands is assessed by 11 kinematic parameters when performing the following motor exercises: fingers tapping, opening / closing the palm, pronation / supination of the hand. Mimic activity is assessed by 11 kinematic parameters when performing the following motor exercises: eyes closing, eyebrows raising, smiling with effort, frowning. Facial expressiveness is characterized by the degree of expressiveness, calculated as the degree of difference between the emotional expression, formed both when imitating the emotional expression depicted on the photograph shown to the subject, and when freely expressing the named emotion, from the neutral facial expression of the subject. In previous studies 35 features, including the features of all motor exercises for assessing the motor activity of the hands, features of mimic activity when performing the exercises "frown" and "smile with effort", features of facial expressiveness when imitating the emotional expressions depicted in the photographs, were recognized as informative. In this work, as a result of correlation analysis, the feature space was reduced to 19 features. The calculation of the accuracy of the binary classification was carried out on the subsets of features of motor activity of the hands, hypomimia and the whole set of 19 features. When using all 19 features, including features of all 3 motor tasks, the highest classification result was obtained – 93.8 %. After additional feature selection using an ensemble of classifiers based on decision trees, the classification accuracy reached 96.9 % using naive Bayesian classifierand 8 features of all 3 motor tasks. The achieved values of the classification accuracy for the separately selected subsets of features of motor activity of the hands and hypomimia are also high: 90.6 % and 93.8 %, respectively. The best feature set consisted of 8 features, still including features of all 3 motor tasks, which indicates the importance of joint assessment of hypokinesia in both considered localizations. A set of 8 features includes speed of opening / closing the palm, speed of pronating / supination of the hand, number of movements of opening / closing the palm, speed of the fingers tapping, the duration of the ‘frown’ exercise, the duration of the ‘smile with effort’ exercise, variations in the amplitude of movements during the ‘frown’ exercise, the degree of expression of imitating the emotion of surprise. The results obtained, in general, are consistent with the results published in other works, devoted to the recognition of PD using various sets of hypokinesia features. Taking into account the availability of the selected localizations (face and hands) for registration, the results obtained in this study show the suitability of the developed method of automated PD recognition for screening this disease.
- Samii A., Nutt J.G., Ransom B.R. Parkinson’s disease. The Lancet. 2004. V. 363. P. 1783–1793.
- Bayramukova A.M., Azhakhmetova A.K., Karpov S.M. Epidemiologiya bolezni Parkinsona v razlichnykh stranakh mira. Uspekhi sovremennogo estestvoznaniya. 2013. № 9. S. 20–21 (in Russian).
- Katunina A.E., Titova N.V. Diagnostika i lecheniye rannikh stadiy bolezni Parkinsona: metodicheskoye posobiye. M. 2011. S. 3 (in Russian).
- Willis A.W., Schootman M., Evanoff B.A., Perlmutter J.S., Racette B.A. Neurologist care in parkinson disease: a utilization, outcomes, and survival study. Neurology. 2011. V. 77. № 9. P. 851–857.
- Levin O.S., Datiyeva V.K. Diagnostika i lecheniye ranney stadii bolezni Parkinsona. Consilium medicum. 2012. T. 9. S. 47–54. (In Russian).
- Razdorskaya V.V., Yudina G.K. Klinicheskaya diagnostika bolezni Parkinsona. BMIK. 2014. №3. URL: https://cyberleninka.ru/article/n/klinicheskaya-diagnostika-bolezni-parkinsona (data obrashcheniya: 22.02.2021) (in Russian).
- Goetz C.G., Tilley B.C., Shaftman S.R. et al. Movement Disorder Society-sponsored revision of the Unified Parkinson’s Disease Rating Scale (MDS-UPDRS): scale presentation and clinimetric testing results. Mov. Disord. 2008. V. 23. P. 2129–2170.
- Richards M., Marder K., Cote L., Mayeux R. Interrater reliability of the Unified Parkinson's Disease Rating Scale motor examination. Mov. Disord. 1994. V. 9. № 1. P. 89–91.
- Evers L.J.W., Krijthe J.H., Meinders M.J., Bloem B.R., Heskes T.M. Measuring Parkinson's disease over time: the real-world within-subject reliability of the MDS-UPDRS. Mov. Disord. 2019. V. 34. P. 1480–1487.
- Moshkova A., Samorodov A., Voinova N., Volkov A., Ivanova E., Fedotova E. Facial Emotional Expression Assessment in Parkinson's Disease by Automated Algorithm Based on Action Units. 2020 27th Conference of Open Innovations Association (FRUCT). 2020. P. 172–178.
- Moshkova A., Samorodov A., Ivanova E., Fedotova E., Voinova N., Volkov A. Studying Facial Activity in Parkinson's Disease Patients Using an Automated Method and Video Recording. 2021 28th Conference of Open Innovations Association (FRUCT). 2021. P. 301–308.
- Moshkova A., Samorodov A., Ivanova E., Fedotova E., Voinova N. Hand Movement Kinematic Parameters Assessment for Parkinson’s Disease Patients. 2021 IEEE Conference of Russian Young Researchers in Electrical and Electronic Engineering (EIConRus). 2021.
- Ekonometrika: Uchebnik / pod red. I.I. Eliseyevoy. M.: Finansy i statistika. 2002. S. 344 (in Russian).
- Sahyoun A., Chehab K., Al-Madani O., Aloul F., Sagahyroon A. ParkNosis: Diagnosing parkinsons disease using mobile phones. in 2016 IEEE 18th International Conference on e-Health Networking, Applications and Services (Healthcom). 2016. P. 1–6.
- Langevin R., Ali M.R., Sen T., Snyder C., Myers T., Dorsey E.R., Hoque M.E. The PARK framework for automated analysis of Parkinson’s disease characteristics. Proceedings of the ACM on Interactive, Mobile, Wearable and Ubiquitous Technologies. 2019. V. 3. № 2. P. 1–22.
- Giovanni A. et al. An integrated multi-sensor approach for the remote monitoring of Parkinson’s disease. Sensors. 2019. V. 19. № 21. Article number: 4764. [Elektronnyy resurs]. – Rezhim dostupa: URL: https://doi.org/10.3390/s19214764
- Butt A.H. et al. Objective and automatic classification of parkinson disease with leap motion controller. Biomed. Eng. Online. 2018.
V. 17. Article number: 168. [Elektronnyy resurs]. – Rezhim dostupa: URL: https://doi.org/10.1186/s12938-018-0600-7 - Maachi I.El, Bilodeau G.A., Bouachir W. Deep 1D-convnet for accurate Parkinson disease detection and severity prediction from gait. Expert Syst. Appl. 2020. V. 143. Article number: 113075. [Elektronnyy resurs]. – Rezhim dostupa: URL: https://doi.org/10.1016/ j.eswa.2019.113075
- Vinokurov N., Weinshall D., Arkadir D., Bergman H., Linetsky E. Quantifying hypomimia in parkinson patients using a depth camera. Communications in computer and information science. 2016. V. 604. P. 63–71.
- Kovalenko E. et al. Distinguishing Between Parkinson’s Disease and Essential Tremor Through Video Analytics Using Machine Learning: a Pilot Study. IEEE Sensors Journal. Preprint [Elektronnyy resurs]. – Rezhim dostupa: URL: https://doi.org/10.1109/ JSEN.2020.3035240