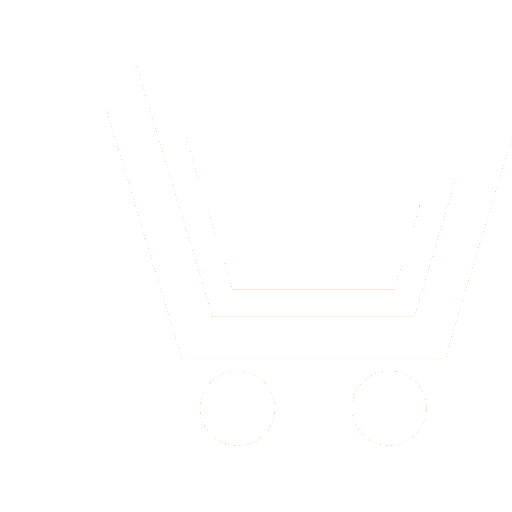
V.N. Gridin¹, I.A. Kuznetsov², A.I. Gazov³, E.S. Sirota4
1,2,3,4 Design Information Technologies Center Russian Academy of Sciences (DITC) (Moscow Region, Odintsovo, Russia)
4 I.M. Sechenov First Moscow State Medical University (Moscow, Russia)
The paper considers an integrated approach for constructing models for predicting the perioperative parameters of laparoscopic kidney resections, which include the duration of the operation, the time of thermal ischemia, and the glomerular filtration rate 24 hours after the operation. The approach is based on the principle of expanding the feature space, extracted from the analysis of the surgeon's "learning curve" data when mastering laparoscopic kidney resections. The aim of this work is to predict the main perioperative parameters that have the most significant impact on the surgical tactics of treatment at the stage of planning surgery. New methods have been developed for identifying significant parameters that take into account the complexity of the operation and the qualifications of the surgeon based on his “learning curve”. The parameters to be distinguished include: “complexity of the operation” based on nephrometric indices (RENAL, PADUA and C-index); the average value of the predicted perioperative parameters of surgical interventions depending on the complexity; slope and standard error based on the regression line of predicted perioperative parameters. Models were developed for predicting the perioperative parameters of laparoscopic organ-preserving kidney interventions using modern approaches based on machine learning, which are based on the algorithms “decision trees”, “multilayer perceptron”, “Naïve Bayes”, “logistic regression”. A comparative analysis of the quality of the developed models was carried out, as a result of which the best result was obtained using the “logistic regression” algorithm. The F-measure was used as a metric. A comparative analysis of the developed models was carried out to assess the impact on the final quality of the new selected features. For the predicted parameter “time of thermal ischemia” the increase was from 9.68% to 16.68%; for the predicted parameter “duration of surgery” the increase was from 2.76% to 4.08%. At the same time, for the predicted parameter “GFR in 24 hours” there was no significant increase, and for the “multilayer perceptron” algorithm it turned out to be negative. The obtained forecasting models can be used in applied software solutions that act as decision support systems in determining the surgical tactics of treating patients with localized formations of the renal parenchyma. Such software solutions can be implemented as a web service or as a separate program.
Gridin V.N., Kuznetsov I.A., Gazov A.I., Sirota E.S. Prediction of perioperative parameters of laparoscopic organ-sparing interventions on the kidney taking into account the surgeon's "learning curve". Biomedicine Radioengineering. 2021. V. 24. № 2. Р. 13−20. DOI: https://doi.org/10.18127/j15604136-202102-02 (in Russian).
- Campbell S. et al., Renal Mass and Localized Renal Cancer: AUA Guideline. J. Urol. 2017. V. 198. № 3. P. 520–529. Doi: 10.1016/j.juro.2017.04.100
- Ljungberg B. et al., EAU guidelines on renal cell carcinoma: 2014 update. Eur. Urol. 2015. V. 67. № 5. P. 913–924. Doi: 10.1016/j.eururo.2015.01.005
- Pushkar D.Y., Alyaev Y.G., Glybochko P.V. Rossijskie klinicheskie rekomendacii po urologii. GEOTAR-Media. 2016. S. 496 (in Russian).
- Subramonian K., Muir G. The ‘learning curve’ in surgery: what is it, how do we measure it and can we influence it? BJU Int. 2004. V. 93. № 9. P. 1173–1174. Doi: 10.1111/j.1464-410X.2004. 04891.x
- Abboudi H. et al., Learning curves for urological procedures: a systematic review. BJU Int. 2014. V. 114. № 4. P. 617–629. Doi: 10.1111/bju.12315
- Kutikov A., Uzzo R.G. The R.E.N.A.L. Nephrometry Score: A Comprehensive Standardized System for Quantitating Renal Tumor Size, Location and Depth. J. Urol. 2009. V. 182. № 3. P. 844–853. Doi: 10.1016/j.juro.2009.05.035
- Ficarra V. et al., Preoperative Aspects and Dimensions Used for an Anatomical (PADUA) Classification of Renal Tumours in Patients who are Candidates for Nephron-Sparing Surgery. Eur. Urol. 2009. V. 56. № 5. P. 786–793. Doi: 10.1016/j.eururo.2009.07.040
- Simmons M.N., Ching C.B., Samplaski M.K., Park C.H., Gill I.S. Kidney Tumor Location Measurement Using the C Index Method. J. Urol. 2010. V. 183. № 5. P. 1708–1713. Doi: 10.1016/j.juro.2010.01.005
- Chowdhary C.L., Acharjya D.P. Segmentation and Feature Extraction in Medical Imaging: A Systematic Review. Procedia Computer Science. 2020. V. 167. P. 26-36. Doi: 10.1016/j.procs.2020.03.179
- Pacheco F., Exposito E., Aguilar J., Gineste M., Baudoin C. A novel statistical based feature extraction approach for the inner-class feature estimation using linear regression. International Joint Conference on Neural Networks (IJCNN). Rio de Janeiro. 2018. P. 1–8. Doi: 10.1109/IJCNN.2018.8488992
- Ferdous M., Debnath J., Chakraborty N.R. Machine Learning Algorithms in Healthcare: A Literature Survey. 11th International Conference on Computing, Communication and Networking Technologies (ICCCNT). Kharagpur. India. 2020. P. 1–6. Doi: 10.1109/ICCCNT49239.2020.9225642
- Guseva A.I., Kuznetsov I.A. The use of entropy measure for higher quality machine learning algorithms in text data processing. Proceedings 2017 5th International Conference on Future Internet of Things and Cloud Workshops (FiCloudW). 2017. P. 47–52. Doi:10.1109/FiCloudW.2017.84
- Ruiz-Chavez Z., Salvador-Meneses J., Garcia-Rodriguez J. Machine Learning Methods Based Preprocessing to Improve Categorical Data Classification. In: Yin H., Camacho D., Novais P., Tallón-Ballesteros A. (eds) Intelligent Data Engineering and Automated Learning – IDEAL 2018. IDEAL 2018. Lecture Notes in Computer Science. 2018. V. 11314. Springer, Cham. Doi: 10.1007/978-3-030-03493-1_32