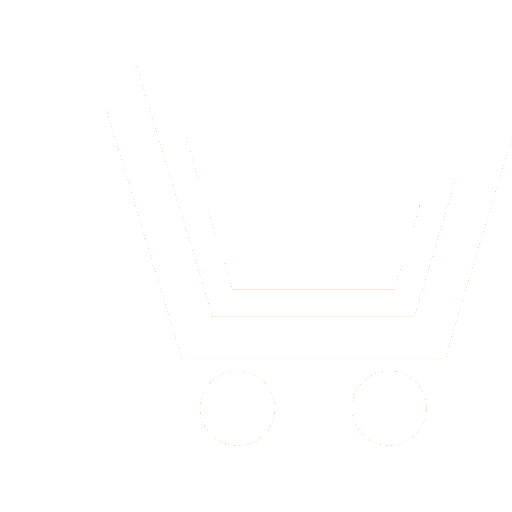
N.T. Abdullaev¹, U.N. Musevi², K.S. Pashaeva³
- Azerbaijan Technical University (Baku, Azerbaijan)
- Azerbaijan State University of Oil and Industry (Baku, Azerbaijan)
- Baku Higher Oil School (Baku, Azerbaijan)
Formulation of the problem. This work is devoted to the use of artificial neural networks for diagnosing the functional state of the gastrointestinal tract caused by the influence of parasites in the body. For the experiment, 24 symptoms were selected, the number of which can be increased, and 9 most common diseases. The coincidence of neural network diagnostics with classical medical diagnostics for a specific disease is shown.
The purpose of the work is to compare the neural networks in terms of their performance after describing the methods of preprocessing, isolating symptoms and classifying parasitic diseases of the gastrointestinal tract. Computer implementation of the experiment was carried out in the NeuroPro 0.25 software environment and optimization methods were chosen for training the network: "gradient descent" modified by Par Tan, "conjugate gradients", BFGS.
Results. The results of forecasting using a multilayer perceptron using the above optimization methods are presented. To compare optimization methods, we used the values of the minimum and maximum network errors.
Comparison of optimization methods using network errors makes it possible to draw the correct conclusion that for the task at hand, the best results were obtained when using the "conjugate gradients" optimization method.
Practical significance. The proposed approach facilitates the work of the experimenter-doctor in choosing the optimization method when working with neural networks for the problem of diagnosing parasitic diseases of the gastrointestinal tract from the point of view of assessing the network error.
Abdullaev N.T., Musevi U.N., Pashaeva K.S. Application of various optimization methods in calculating the error of a neural network for diagnosing parasitological diseases of the gastrointestinal tract. Biomedicine Radioengineering. 2021. V. 24. № 1. P. 29–34. DOI: 10.18127/j15604136
- Hajkin S. Nejronnye seti: Polnyj kurs. M.: Izdatel'skij dom «Vil'yams». 2006. 1104 s. (In Russian).
- Vyuchejskaya M.V., Krajnova I.N., Gribanov A.V. Nejrosetevye tekhnologii v diagnostike zabolevanij (obzor). Zhurnal medikobiologicheskih issledovanij. 2018. T. 6. № 3. S. 284–294 (In Russian).
- Maclin P.S., Dempsey J. Using an Artificial Neural Network to Diagnose Hepatic Masses. Journal Medical Systems. 1992. V. 16. № 5. P. 215–225 (In Russian).
- Kazmierczak S.C., Catrou P.G., Van Lente F. Diagnostic Accuracy of Pancreatic Enzymes Evaluated by Use of Multivariate Data Analysis. Clinical Chemistry. 1993. V. 39. № 9. P. 1960–1965 (In Russian).
- Mironov P.I., Lutfarahmanov I.I., Ishmuhametov I.H., Timerbulatov V.M. Iskusstvennye nejronnye seti v prognozirovanii razvitiya sepsisa u pacientov s tyazhelym ostrym pankreatitom. Annaly hirurgicheskoj gepatologii. 2008. T. 13. № 2. S. 85–89 (In Russian).
- https://www.looduspere.ee/ru/kishechnye-parasity/
- Diagnostika parazitarnyh infekcij https://empendium.com/ru/chapter/B33.V.28.4. (In Russian).
- https://ru.wikipedia.org/wiki/Spisok_parazitov_cheloveka (In Russian).
- Kulikov L.K., Bykova N.M., Privalov Yu.A., Navtanovich N.A., Shabanova O.G. Diagnostika giperal'dosteronizma t bol'nyh s incedentalomami nadpochechnikov pri pomoshchi nejronnyh setej. Fundamental'nye issledovaniya. 2010. № 3. S. 88–94 (In Russian).
- http://www.fundamental-research.ru/ru/article/view-id=6659/
- Ludermir T.B., Yamazaki A., Zanchettin C. An Optimization Methodolgy for Neural Network Weights and Architectures. IEEE Transaction on Neural Networks. 2006. V. 17. № 6. P. 1452–1459 (In Russian).