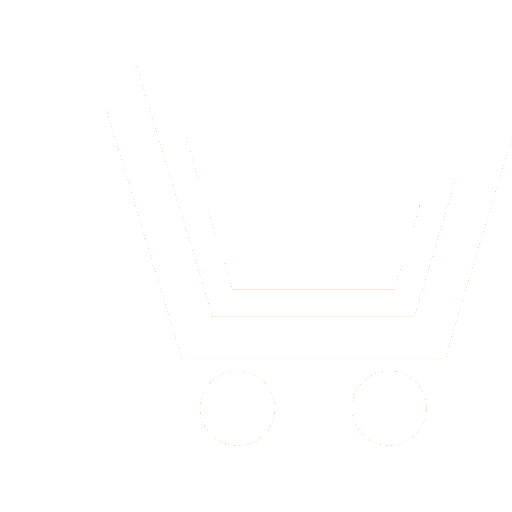
L.N. Anishchenko – Ph.D. (Eng.), Associate Professor, Senior Research Scientist, Research Section of scientific
and Educational Complex “Basic Sciences”, Bauman Moscow State Technical University
E-mail: anishchenko@rslab.ru
A.V. Turetskaya – Student, Department “Biomedical Engineering”, Bauman Moscow State Technical University
E-mail: asi300@yandex.ru
A.S. Bugaev – Academician of RAS, Dr. Sc. (Phys.-Math.), Laboratory of Remote Sensing of the Scientific
and Educational Complex “Fundamental Sciences”, Bauman Moscow State Technical University
E-mail: rslab@rslab.ru
Stress is a normal physiological reaction to changes of environmental conditions, psychological or social factors. Chronic stress, which is one of the fundamental problems of modern society, can lead to different irreversible negative physiological and psychological changes. The methods and devices currently used to detect stress in medical practice cannot be used for everyday longterm automated monitoring of a person’s stress state, as they are laborious and uncomfortable for a subject due to the need of contact sensors usage and involvement of qualified specialists to analyze data. Thus, the development of new effective methods for non-contact daily assessment of stress levels is an up-to-date problem.
In this work, two BioRASCAN-24 monochromatic bio-radars with probing frequencies in the range of 24.0 – 24.2 GHz were used for conducting experiments. The simultaneous use of spaced transceivers allowed observing the subject from different angles. This ensures that the examinees vital parameters are recorded by at least one bioradar even if the view of the thorax movements is partially blocked by examinees body for another bioradar.
34 practically healthy volunteers characterized by varying level of fitness and stress resistance took part in the experiments: 4 volunteers were overweight (body-mass index(BMI) above 25 kg/m2) and 5 subjects regularly took exercises. The test subject was sitting in front of bioradars at a distance of 1.5 m. Each volunteer was asked to perform successive experiments simulating a calm state and a state of mental stress lasting 5 and 3 minutes, respectively.
Data processing consisted of three following stages: preliminary processing of the bioradar signals, features selection and extraction, and utilizing machine learning for training a classifier to identify the stress state of the subject. Bioradar signal processing and classification algorithms were implemented in Python 3.7.
To form a feature vector, we used the parameters of bioradar signals estimated in the time and frequency domains, as well as using recurrence analysis. For successful training of the classifier, collinear features containing redundant information were removed using the threshold value of the correlation coefficient of 0.7. The remaining 6 features were used to train the steady state/mental stress classifier.
In the present work the 14 most commonly used in practice machine learning algorithms were utilized to identify the state of mental stress against the background of a relaxed one according to bioradar data. The best result was shown by the CatBoost classifier. In the classifying between a calm state and a mental stress, this method allows to identify the latter with an accuracy of 89% and F1-score of 88%. Moreover, it does not require the usage of any additional information about the fitness state of the user or his/her BMI.
In the course of further studies, it is supposed to expand the experimental dataset with data from people of other age groups. In addition, it is planned to conduct studies of longer duration in order to be able to identify changes in the psychophysiological state due to the fatigue of the subject.
- Selye H. The Evolution of the stress concept. The originator of the concept traces its development from the discovery in 1936 of the alarm reaction to modern therapeutic applications of syntoxic and catatoxic hormones. American Scientist. 1973. V. 61(6). P. 692–699.
- Selye H. Stress and Distress. In Comprehensive Therapy. 1975. V. 1(8). P. 9–13.
- Steptoe A., Kivimäki M. Stress and cardiovascular disease. Nat Rev Cardiol. 2012. V. 9(6). P. 360–70.
- Glozier N. et.al. Psychosocial risk factors for coronary heart disease. Med. J. Aust. 2013. V. 199(3). P. 179–180.
- Kelly S.J., Ismail M. Stress and type 2 diabetes: a review of how stress contributes to the development of type 2 diabetes. Annu Rev Public Health. 2015. V. 18(36). P. 441–462.
- Van Winkel R., Stefanis N.C., Myin-Germeys I.I. Psychosocial Stress and Psychosis. A Review of the Neurobiological Mechanisms and the Evidence for Gene-Stress Interaction. Schizophrenia Bulletin. 2008. V. 34(6). P. 1095–1105.
- McKlveen J.M., Morano R.L., Fitzgerald M. et al. Chronic Stress Increases Prefrontal Inhibition: A Mechanism for Stress-Induced Prefrontal Dysfunction. Biological psychiatry. 2016, V. 80(10). P. 754–764.
- Slavich G.M., Irwin M.R. From stress to inflammation and major depressive disorder: a social signal transduction theory of depression. Psychol. Bull. 2014. V. 140(3). P. 774–815.
- Dickerson S.S., Kemeny M.E. Acute Stressors and Cortisol Responses: a Theoretical Integration and Synthesis of Laboratory Research. Psychological Bulletin. 2004. V. 130(3). P. 355–391.
- Setz C. et al. Discriminating Stress from Cognitive Load using a Wearable EDA Device. IEEE Transactions on Information Technology in Biomedicine. 2010. V. 14(2). P. 410–417.
- Vrijkotte T.G., van Doornen L.J., de Geus E.J. Effects of Work Stress on Ambulatory Blood Pressure, Heart Rate, and Heart Rate Variability. Hypertension. 2000. V. 35(4). P. 880–886.
- Fairclough S.H. Fundamentals of physiological computing. Interacting with Computers. 2009. V. 21(1–2). P. 133–145.
- Mokhayeri F., Akbarzadeh-T M.R., Toosizadeh S. Mental Stress Detection using Physiological Signals based on Soft Computing Techniques. In Proceedings of the 18th IEEE Iranian Conference of Biomedical Engineering (ICBME). 2011. Tehran. Iran. P. 232–237.
- Deschênes A. et al. Facial Expression Recognition Impairment following Acute Social Stress. Journal of vision. 2015. V. 15(12). P. 1383.
- Anishchenko L., Bechtel T., Ivashov S., Alekhin M., Tataraidze A., Vasiliev I. Bioradiolocation as a technique for remote monitoring of vital signs. In: Advanced Ultrawideband Radar: Signals, Targets and Applications. Taylor J.D. (Ed.). CRC Press. 2016. P. 297–322.
- Fernandez J.R.M., Anishchenko L. Mental stress detection using bioradar respiratory signals. Biomedical Signal Processing and Control. May 2018. V. 43. P. 244–249.
- Anishchenko L. Challenges and Potential Solutions of Psychophysiological State Monitoring with Bioradar Technology. Diagnostics 2018. V. 8. P. 73.
- Anishchenko L., Zhuravlev A., Razevig V. Chizh M., Evteeva K., Korostovtseva L., Bochkarev M., Sviryaev Yu. Non-contact Sleep Disorders Detection Framework for Smart Home. Proc. of 2019 PhotonIcs & Electromagnetics Research Symposium (PIERS). Rome, Italy. 2019. June 17–20. P. 3553–3557.
- Li C., Lin J. Microwave noncontact motion sensing and analysis. 1st ed. Wiley: New Jersey. USA. 2014.
- Sun F.-T. et al. Activity-aware Mental Stress Detection Using Physiological Sensors, in International Conference on Mobile Computing, Applications, and Services. 2010: Heidelberg, Berlin. P. 282–301.
- Schmidt P. et al. Introducing wesad, a multimodal dataset for wearable stress and affect detection // Proceedings of the 20th ACM International Conference on Multimodal Interaction. 2018. S. 400–408.
- Karthikeyan P., Murugappan M., Yaacob S. Multiple Physiological Signal-Based Human Stress Identification Using Non-Linear Classifiers. Elektronika ir Elektrotechnika. 2013. V. 19(7). P. 80–85.
- Subhani A.R. et al. Machine Learning Framework for the Detection of Mental Stress at Multiple Levels. IEEE Access. 2017. V. 5. P. 13545–13556.
- Anishchenko L.N., Tureckaya A.V. Programmno-apparatnyj kompleks dlya beskontaktnoj ocenki psihofiziologicheskogo sostoyaniya cheloveka // Biomedicinskaya radioelektronika. 2018. № 10. S. 61–66. (In Russian).