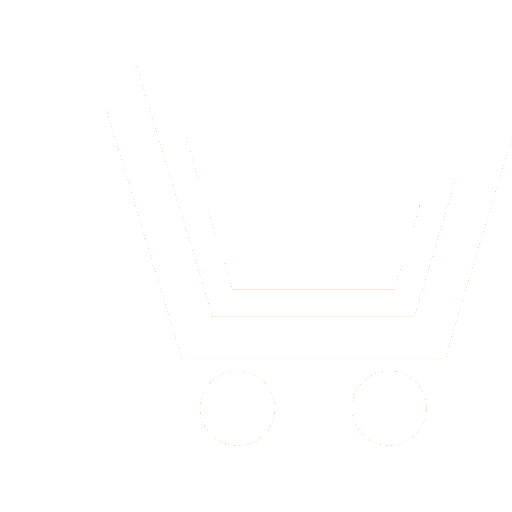
V.Yu. Soboleva – Statistical Programmer I, Parexel International (Moscow); Master of Science,
Department “Medical and Technical Information Technologies” (BMT-2),
Bauman Moscow State Technical University
E-mail: vitalina.soboleva@yandex.ru
A.I. Malakhov – Ph.D. (Eng.), Associate Professor, Department “Medical and Technical Information Technologies” (BMT-2), Bauman Moscow State Technical University
E-mail: aimalakhov@bmstu.ru
V.Yu. Kaplunova – Dr. Sc. (Med.), Chief Research Scientist, NIO “Metabolic Syndrome” Research Center,
Department of Hospital Therapy No. 1, Faculty of Medicine, I.M. Sechenov First Moscow State Medical University
E-mail: kaplunovavy@mail.ru
A.M. Levando – Software Engineer, Bitrobotics; Master of Science, Department “Medical and Technical Information Technologies” (BMT-2), Bauman Moscow State Technical University
E-mail: anton-leva@yandex.ru
M.S. Shmatkova – Analyst, Venta Grande; Master of Science, Department “Medical and Technical Information Technology” (BMT-2) Bauman Moscow State Technical University
E-mail: shmatkova.maria@gmail.com
According to the world Healthcare Organization predictions in 2030 around 23.6 million people will die from cardiovascular diseases (CVD). Currently CVDs is the leading cause of death worldwide. Diagnosis and timely initiation of CVD treatment are the most important tasks in clinical practice. The use of prediction and classification models in medical practice helps to improve the efficiency and quality of diagnosis and treatment. The goal of creating an accurate model is to assess the risks of complications in patients as early and accurately as possible and to develop an individual treatment plan for the quality of medical care improvement. Prediction models cannot substitute the clinical expertise, but they can provide evidence-based data about a person's risk of developing diseases and avoid some common mistakes seen in clinical decision-making.
The aim of the study was to develop a heart disease classification model with the possibility of hypertrophic cardiomyopathy (HCM) detecting based on the analysis of the heart departments phase activity parameters obtained using the precordial electroimpedance study.
The classification model was based on the database of heart examination results obtained with the help of precordial reography method. The data were collected from 2016 to 2019 years in patients of the cardiological department of Medical University hospital №1 of I.M. Sechenov First Moscow State Medical University. The total number of subjects was 47. The average age of patients was 60 ± 10 years. The studied disease was hypertrophic cardiomyopathy. During the study the following parameters were evaluated: atrial pre-ejection period (APEP), atrial ejection time (AET), pre-ejection period (ventricular) (PEP) and right ventricular ejection time (RVET). In the data sample patients had the following clinically confirmed diseases: 38% of patients had HCM, 12% had IHD, 6% had atrial fibrillation, 4% had heart failure, 6% had aortic valve damage, 12% had angina, 11% had Tachycardia, and 11% had mitral valve damage.
As a result of the study was conducted that the AET parameter should be used in prediction models with wider range of predictors, including various types of research and analysis of the cardiovascular system. The logistic regression model can be applied to a larger sample size and more predictors to achieve higher sensitivity.
- Hypertrophic Cardiomyopathy. Rick A. Nishimura, MD, Steve R. Ommen, MD, and A.J. Tajik, MD, 2004 [Elektronnyj resurs]. Rezhim dostupa: https://www.researchgate.net/publication/8581547_Hypertrophic_cardiomyopathy (25.11.2019).
- Malakhov A.I., Tikhomirov A.N., Shchukin S.I., Kaplunova V.Y., Markova N.Y., Kozlova N.V. Algorithm for detecting HCM signs based on the precordial rheocardial signals analysis // AIP Conference Proceedings 2140, 020042 (2019); https://doi.org/10.1063/1.5121967.
- Gipertroficheskaya kardiomiopatiya. Klinicheskie rekomendacii. [Elektronnyj resurs]. Rezhim dostupa: https://scardio.ru/content/activities/2019/ guide/KR_GKMP_2019.pdf (10.04.2019).
- Malahov A.I. Biotekhnicheskaya sistema mnogokanal'nyh elektroimpedansnyh issledovanij fazovoj struktury deyatel'nosti serdca: Dis. … kand. tekhn. nauk. M. 2018. 127 s.
- Maren E., Shipe1, Stephen A. Deppen1, Farhood Farjah, Eric L. Grogan. Developing prediction models for clinical use using logistic regression: an overview. [Elektronnyj resurs]. Rezhim dostupa: https://www.ncbi.nlm.nih.gov/pubmed/31032076 (01.03.2020).
- Jenni A. M., Chris J. Machine learning in medicine: a practical introduction. [Elektronnyj resurs]. Rezhim dostupa: https://bmcmedresmethodol. biomedcentral.com/articles/10.1186/s12874-019-0681-4 (20.04.2019).
- Generalized Linear Models in R Part 2: Understanding Model Fit in Logistic Regression Output. [Elektronnyj resurs]. Rezhim dostupa: https://www.theanalysisfactor.com/r-glm-model-fit/ (05.04.2020).
- David W. Hosmer, Jr., Stanley Lemeshow, Rodney X. Sturdivant, Applied Logistic Regression. Second Edition. John Wiley & Sons. 2013. 528 p.
- Shahadat Uddin, Arif Khan, Md Ekramul Hossain, Mohammad Ali Moni. Comparing different supervised machine learning algorithms for disease prediction. [Elektronnyj resurs]. Rezhim dostupa: https://bmcmedinformdecismak.biomedcentral.com/articles/10.1186/s12911-019-1004-8 (12.04.2019).