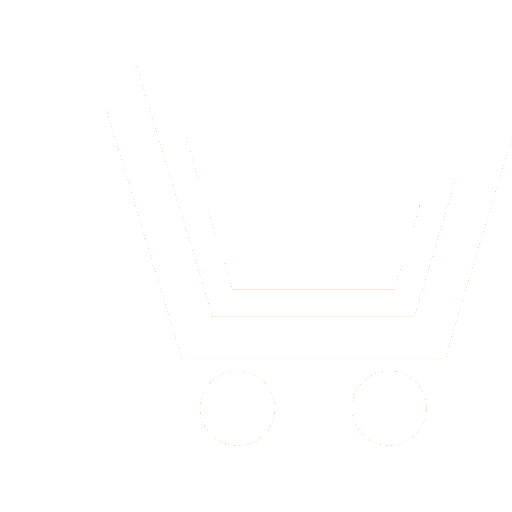
O.V. Melnik – Dr. Sc. (Eng.), Professor, Department “Information-Measuring and Biomedical Engineering”, Ryazan State Radio Engineering University n.a. acad. V.F. Utkin
E-mail: omela111@yandex.ru
V.A. Sablina – Ph.D. (Eng.), Associate Professor, Department “Electronic Computing Machines”, Ryazan State Radio Engineering University n.a. acad. V.F. Utkin
E-mail: flyingvictory@mail.ru
A.V. Savin – Post-graduate Student, Department “Electronic Computing Machines”,
Ryazan State Radio Engineering University n.a. acad. V.F. Utkin
E-mail: savin.alex@mail.ru
A.B. Borschev – Undergraduate, Department “Electronic Computing Machines”,
Ryazan State Radio Engineering University n.a. acad. V.F. Utkin
Statement of the problem. Automatic determination of the psychoemotional state of a person based on the analysis of video sequences is an urgent task in various fields related to ensuring the safety of production, air and transport communications, crime prevention and terrorist threats, etc. One of the key steps in the automatic assessment of emotions by facial expression is the correct detection of facial anthropometric points, which is a difficult task in the condition of individual variability in the shape and appearance of faces of different people, varying distances to the camera, posture and position of the human head, variable lighting and possible overlapping faces with foreign objects.
Aim of the work. Development and software implementation of a model of a multitask cascade convolutional neural network for detecting anthropometric points of a face in an image that provides stable recognition under conditions of large differences in scale, posture and occlusion (strong or partial obstruction of the face by another object).
Results. A model of a deep neural network based on a three-stage transformation of information was developed, which allows, in contrast to known modifications, to jointly solve the problems of detection and face alignment. The neural network was trained on a set of training examples WIDER FACE dataset, pre-grouped into three levels of complexity depending on the frequency of detection of facial frames. It is shown that on a large and medium scales a high level of detection is achieved – more than 90% even for objects of the “middle” and “heavy” classes. The impact of occlusion and posture deformation on recognition efficiency has been evaluated. Assessment by the WIDER face standard showed a significant increase in the performance of the proposed model compared to face detection methods without in-depth study.
Practical significance. The proposed model can be used as an integral part of the system for recognizing emotions based on the analysis of video sequences.
- Novikova E.I., Aleshina L.I., Marinina M.G., Fedoseeva S.Yu. Osnovy nejropsihologii i psihofiziologii: Ucheb.-metod. posobie. Volgograd: Volgogradskij gosudarstvennyj social'no-pedagogicheskij universitet, «Peremena», 2019. 87 c. [elek-tronnyj resurs]. URL: http://www.iprbookshop.ru/84396.html (data obrashcheniya: 23.04.2020) (In Russian).
- Ekman P. Psihologiya emocij. SPb.: Piter. 2018. 334 s. (In Russian).
- Nikolaeva E.I. Psihofiziologiya. Psihologicheskaya fiziologiya s osnovami fiziologicheskoj psihologii: Uchebnik. Izd. 4-e. M.–Saratov: PER SE, Aj Pi Er Media. 2019. 623 c. [elektronnyj resurs]. URL: http://www.iprbookshop.ru/88212.html (data obrashcheniya: 23.04.2020) (In Russian).
- Shishkoedov P.N. Obshchaya psihologiya. M.: Eksmo. 2009. 284 s.
- Ekman P., Rosenberg E.L., What the face reveals: Basic and Applied Studies of Spontaneous Expression Using the Facial Action Cod-ing System (FACS), Second Edition. Oxford University Press. USA. 2005. 672 p.
- Yan W.-J., Wang S.-J., Liu Y.-J., Wu Q., Fu X. For micro-expression recognition: Database and suggestions. Neurocomputing. 2014. V. 136. P. 82–87.
- Sablina V.A., Sergeeva A.D. Klassifikaciya metodov obnaruzheniya antropometricheskih tochek lica. Biotekhnicheskie, medi-cinskie i ekologicheskie sistemy, izmeritel'nye ustrojstva i robototekhnicheskie kompleksy. Biomedsistemy 2019: Sb. tr. XXXII Vseros. nauch.-tekhn. konf. stud., mol. uchenyh i spec., 4 6 dekabrya 2019 g.. Pod obshch. red. V.I. ZHuleva. Ryazan': IP Ko-nyahin A.V. (Book Jet), 2019. C. 203–206 (In Russian).
- Wu Y., Ji Q. Facial Landmark Detection: A Literature Survey. International Journal of Computer Vision. February 2019. V. 127. Is. 2. P. 115–142.
- Zhang K., Zhang Z., Li Z., Qiao Y. Joint Face Detection and Alignment Using Multitask Cascaded Convolutional Networks. IEEE Sig-nal Processing Letters. Oct. 2016. V. 23. № 10. P. 1499–1503.