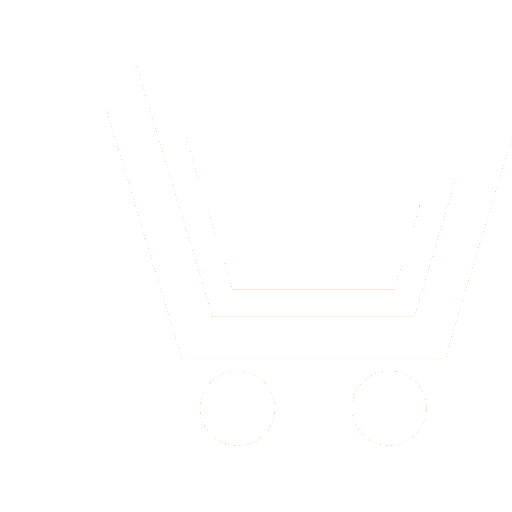
A.M. Nosovskii – Dr.Sc. (Biol.), Leading Research Scientist, Russian Federation State Research Center Institute of Biomedical Problems RAS (Moscow)
K.V. Gordienko – Junior Research Scientist, Russian Federation State Research Center Institute of Biomedical Problems RAS (Moscow) E-mail: k.vl.gordienko@gmail.com
V.E. Novikov – Ph.D. (Med.), Leading Research Scientist, Russian Federation State Research Center Institute of Biomedical Problems RAS (Moscow)
T.A. Smirnova – Ph.D. (Biol.), Leading Research Scienist, Russian Federation State Research Center Institute of Biomedical Problems RAS (Moscow)
G.Yu. Vasilyeva – Ph.D. (Med.), Leading Research Scientist, Russian Federation State Research Center Institute of Biomedical Problems RAS (Moscow)
Formulation of the problem. Being in conditions of reduced functional load is the main reason for decreasing bone mineral density during space flight, which is associated in clinical practice with an increased risk of a non-traumatic type of fracture. However, the participants of the main expeditions of Mir orbital station (OS) and the International Space Station (ISS) have an individual variability of this indicator.
Aim of the work – to assess the possibility of using fuzzy clustering methods to distinguish groups of subjects with a different reaction to a decrease in the functional load associated with a human being in space flight.
Results. An algorithm for dividing into groups by the c-means method with a preliminary determination of the number of clusters by the method of mountain clustering is described.
Using the analysis of variance, the null hypothesis was verified that there was no reaction of the skeletal system to space flight conditions (preflight values were compared with postflight ones) both before and after division into clusters. Differences in results are shown.
Practical significance. The division into clusters allows a deeper study of the effect on biological systems. Moreover, the fuzzy clustering method allows the researcher to independently choose the degree of belonging of the values of the studied parameter to one of the formed clusters.
The allocation of groups according to the reaction to a decrease in functional load will allow adjusting the complex of preventive measures in flight, as well as rehabilitation tactics after the flight.
- Gordienko K., Nosovsky A., Novikov V. Constitutional characteristics and bone mineral content in astronauts before and after flights // 69th International Astronautical Congress. Bremen, 1-5 Oct., 2018. [electronic sources] https://iafastro.directory/iac/paper/id/47768/abstract-pdf/IAC18,A1,IP,12,x47768.brief.pdf-2018-08-01.19:36:53 (18.06.2019).
- Novikov V.E., Rykova M.P., Antropova E.N., Berendeeva T.A., Kalinin S.A., Vasil'eva G.YU., Ponomarev S.A. Mineral'naya plotnost' kostnoj tkani i molekulyarno-geneticheskie markery ee remodelirovaniya v krovi u kosmonavtov posle dlitel'nyh poletov na mezhdunarodnoj kosmicheskoj stancii // Fiziologiya cheloveka. 2018. № 6. S. 88–94.
- Oganov V.S. Kostnaya sistema, nevesomost' i osteoporoz. Izd. 2-e, pererab. i dop. Voronezh: Nauchnaya kniga. 2014. 291 s.
- Boldina M.YU. Primenenie metoda nechetkih mnozhestv v razrabotke instrumentariya sociologicheskogo issledovaniya // Innovacionnaya nauka. 2015. № 12. S. 231–233.
- Manco G., Ortale R., Sacca D. Similarity-based clustering of Web transactions. Proceedings of the 2003 ACM symposium on Applied computing. P. 1212–1216.
- Migaly S., Abonyi J., Szeifert F. Fuzzy Self-Organizing Map Based on Regularized Fuzzy C-Means Clustering. Advances in Soft Computing, Engineering Design and Manufacturing. In J.M. Benitez, O. Cordon, F. Hoffmann, et al. (Eds.), Springer Engineering Series. Revised papers of the 7th On-line World Conference on Soft Computing in Industrial Applications. 2002. P. 99–108.
- SHtovba S.D. Vvedenie v teoriyu nechetkih mnozhestv i nechetkuyu logiku / http://matlab.exponenta.ru/fuzzylogic/book1/12.php (17.06.2019).
- Höppner F., Kruse R., Klawonn F., Runkler T. Fuzzy Cluster Analysis. Methods for Classification, Data Analysis and Image Recognition. Wiley. 1999.
- Bezdek, J.C. Pattern Recognition with Fuzzy Objective Function Algorithms. New York: Plenum Press. 1981. 266 r.
- Yager R.R., Filev D.P. Learning of fuzzy rules by mountain clustering. Proc. SPIE Conf. Applicat. Fuzzy Logic Technol. 1993. P. 246–254.