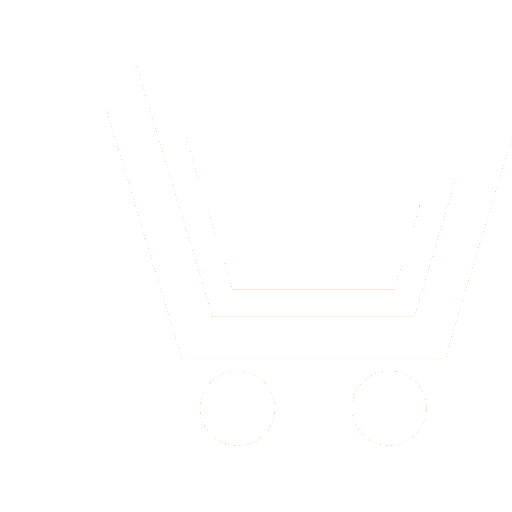
L.N. Anishchenko – Ph.D. (Eng.), Associate Professor, Senior Research Scientist, Biomedical Engineering Department, Research Section of scientific and educational complex “Fundamental Sciences”,
Bauman Moscow State Technical University
E-mail: anishchenko@rslab.ru
Problem statement. The need to solve the problem of detecting falls for the elderly is caused by the well-known phenomenon of global population aging, which is the result of the life expectancy increasing and the birth rate decreasing. Every year, the population over 60 years old increases by 3%. According to the UN estimates in 2030, the number of people over 60 can reach 1.4 billions. Older people are subject to negative physiological changes that can increase the risk of injuries, including those caused by falls. About 13% of all deaths of older people are the result of a lack of help after a fall episode.
The aim of the work – the development of effective methods for detecting falls is an up-to-date medical and engineering problem. Results. We currently have wearable fall sensors on the market, and the scientific community is actively working on the development and improvement of contactless systems that use optical and non-optical sensors for automatic fall detection. However, there are still unresolved issues related primarily to the high level of false alarms, privacy violation, the high cost of the device. The bioradiolocation method is free from a number of the listed drawbacks, however, due to the relatively high cost and complexity of the hardware, the use of this method in practice has been doubting so far. The paper describes an experimental bioradar model for contactless detection of human falls and a classification algorithm based on the application of wavelet analysis and deep learning, which together make it possible to create an inexpensive portable bio-radar for remote fall detection. The architecture of the deep neural network GoogLeNet, previously trained for image recognition, was used to detect falls in the work. The time-frequency representation of the bio-radar signal, representing the absolute values of the wavelet transform coefficients, was used as input data for this network. The Morlet wavelet was used as the base wavelet. The network architecture of GoogLeNet has been adapted to solve the problem of detecting falls. Data processing was performed using the MATLAB 2019a package. The classification accuracy using the proposed algorithm for the test dataset was estimated to be 98.96%. The limitation of this work is that the experimental data used to train the classifier were obtained only for 5 subjects and for relatively small (1–2 m) distances between the radar and the object.
Practical significance. In the course of further research, it is planned to expand the experimental dataset taking into account various surroundings and distances from the radar to a biological object.
- Desa U.N. World Population Prospects, United Nations Department of Economic and Social Affairs // Population Division. 2017.
- Institute of Medicine (US). Division of Health Promotion and Disease Prevention. The second fifty years: Promoting health and preventing disability. National Academy Press. 1992.
- Fernando E. et al. Risk factors associated with falls in older adults with dementia: a systematic review // Physiotherapy Canada. 2017. V. 69. № 2. P. 161–170.
- Prevention O.F. et al. Guideline for the prevention of falls in older persons // J. Am. Geriatr. Soc. 2001. V. 49. № 5. P. 664–672.
- Kau L.J., Chen C.S. A smart phone-based pocket fall accident detection, positioning, and rescue system // IEEE journal of biomedical and health informatics. 2014. V. 19. № 1. P. 44–56.
- Rakhman A.Z. et al. Fall detection system using accelerometer and gyroscope based on smartphone. 2014 The 1st International Conference on Information Technology, Computer, and Electrical Engineering. IEEE. 2014. P. 99–104.
- Rathi N. et al. Wearable low power pre-fall detection system with IoT and bluetooth capabilities. 2017 IEEE National Aerospace and Electronics Conference (NAECON). IEEE. 2017. P. 241–244.
- Agrawal S.C., Tripathi R.K., Jalal A.S. Human-fall detection from an indoor video surveillance. 2017 8th International Conference on Computing, Communication and Networking Technologies (ICCCNT). IEEE. 2017. P. 1–5.
- Sase P.S., Bhandari S.H. Human Fall Detection using Depth Videos. 2018 5th International Conference on Signal Processing and Integrated Networks (SPIN). IEEE. 2018. P. 546–549.
- Anishchenko L. et al. Application of step-frequency radars in medicine. Radar Sensor Technology XVIII. International Society for Optics and Photonics. 2014. V. 9077. P. 90771N.
- Wu Q. et al. Radar-based fall detection based on Doppler time–frequency signatures for assisted living. IET Radar, Sonar & Navigation. 2015. V. 9. № 2. P. 164–172.
- Dremina M.K., Anishchenko L.N. Contactless fall detection by means of CW bioradar. 2016 Progress in Electromagnetic Research Symposium (PIERS). IEEE. 2016. С. 2912–2915.
- Anishchenko L., Alborova I., Dremina M. Discriminant analysis in bioradar-based fall events classification. 2017 IEEE International Conference on Microwaves, Antennas, Communications and Electronic Systems (COMCAS). IEEE. 2017. P. 1–4.
- K-LC5 High Sensitivity Dual Channel Transceiver, URL: https://www.rfbeam.ch/product-id=9.
- Amin M., Ahmad F., Jokanovic B. Personalized fall detection and classification through walls and in heavy indoor clutter. Radar Sensor Technology XIX; and Active and Passive Signatures VI. International Society for Optics and Photonics. 2015. V. 9461. P. 94610H.
- Torrey L., Shavlik J. Transfer learning. Handbook of research on machine learning applications and trends: algorithms, methods, and techniques. IGI Global. 2010. P. 242–264.
- Szegedy C. et al. Going deeper with convolutions. Proceedings of the IEEE conference on computer vision and pattern recognition. 2015. P. 1–9.
- Anishchenko L.N., et al. Low-Cost Portable Bioradar System for Fall Detection. 2019 Progress in Electromagnetic Research Symposium (PIERS). IEEE, 2019. In press.