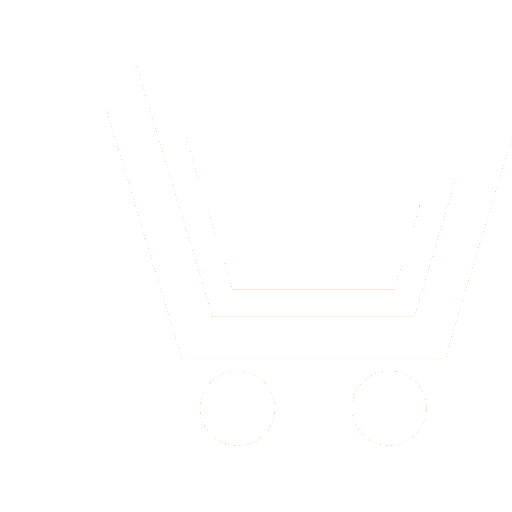
N.S. Konnova – Ph.D. (Eng.), Associate Professor, Bauman Moscow State Technical University
E-mail: nkonnova@bmstu.ru
V.Yu. Khaperskaya – Post-graduate Student, Bauman Moscow State Technical University
E-mail: lynx.lg@gmail.com
Cardiovascular disease is one of the major causes of death worldwide. Therefore, new diagnostic tools should be created to provide early detection to reduce mortality and increase duration and quality of life for patients with heart disease.
Renewed interest in investigating the utility of SCG accelerated recently and benefited from new advances in low-cost lightweight sensors, and machine learning methods. Recent studies demonstrated the clinical utility of SCG signals for the detection and monitoring of cardiovascular conditions.
This article compares various machine learning algorithms (the method of nearest neighbors, the method of support vectors, decision trees, the ensemble of models) and neural networks: based on the architecture of long short-term memory and convolutional. Metrics for evaluating the obtained results were introduced, an original numerical experiment was carried out using the developed mathematical software, in the framework of which the mentioned methods and algorithms were implemented. The effectiveness of the considered models for solving the problem of diagnosing diseases of the cardiovascular system is evaluated. To compare the effectiveness of classifiers, Accuracy, Error, Sensitivity, Specificity, Precision, FP Rate, and F1 measures are given. Moreover, the classifiers are tested on data sets in order to determine the capabilities of the methods of “recognizing” the patient depending on the position of the body, psychophysiological state, etc. (its SСG signal will differ from the originally recorded signal, so it is important to check whether the recognizer is able to correctly classify the signals recorded under various conditions), and, for example, before and after surgery, to check the effectiveness of the same algorithms in the diagnostic problem. For each algorithm and data set, confusion matrices and ROC curves were constructed. During the study, much attention was also paid to the preparation and preliminary processing of data. Results of this research show that convolutional neural networks are very effective at detection of cardiovascular conditions.
- Konnova N.S., Basarab M.A. Application of neural networks in cardiovascular decision support systems. Proc. of 7th International Conference on Advances in Computing, Electronics and Communication (ACEC 2018). 2018. P. 73–78. DOI: 10.15224/978-1-63248-157-3-27.
- Konnova N.S., Basarab M.A., Basarab D.A. Image processing using artificial intelligence methods in cardiovascular decision support systems. Proc. of SPIE V. 10836. 2018. International Conference on Image and Video Processing, and Artificial Intelligence. 2018. 108361U-1. DOI: 10.1117/12.2515343.
- Gamage P.T., Khurshidul. Azad M., Taebi A., Sandler R.H., Mansy H.A. Clustering Seismocardiographic Events using Unsupervised Machine Learning. IEEE Signal Processing in Medicine and Biology Symposium (SPMB). 2018. P. 1–5. DOI: 10.1109/SPMB.2018.8615615.
- Solar B.E., Taebi A., Mansy H.A. Classification of seismocardiographic cycles into lung volume phases. IEEE Signal Processing in Medicine and Biology Symposium (SPMB). 2017. P. 1–2. DOI: 10.1109/SPMB.2017.8257033.
- Yao J., Tridandapani S., Auffermann W.F., Wick C.A., Bhatti P.T. An Adaptive Seismocardiography (SCG)-ECG Multimodal Framework for Cardiac Gating Using Artificial Neural Networks. IEEE Journal of Translational Engineering in Health and Medicine. 2018. V. 6. 1900611. DOI: 10.1109/JTEHM.2018.2869141.
- Qurraie S.S., Afkhami R.G. ECG arrhythmia classification using time frequency distribution technique. Biomedical Engineering Letters. 2017. V. 7. P. 325–332. DOI 10.1007/s13534-017-0043-2.
- Sodmann P., Vollmer M., Nath N., Kaderali L. A convolutional neural network for ECG annotation as the basis for classification of cardiac rhythms. Physiological Measurement. 2018. DOI: 10.1088/1361-6579/aae304.
- Weems A., Harding M., Choi A. Classification of the ECG Signal Using Artificial Neural Network. Institute of Physics and Engineering in Medicine Physiological Measurement. 2018. V. 39. № 10. P. 545–555. DOI 10.1007/978-3-319-17314-6_70.
- Babušiak B., Mohylová J. The EEG signal prediction by using neural network. Advances in Electrical and Electronic Engineering. 2008. V.7. P. 342–345.
- Rajpurkar P., Hannun A.Y., Haghpanahi M, Bourn C., Ng A.N. Cardiologist-Level Arrhythmia Detection with Convolutional Neural Networks. Nature Medicine. 2019. № 25. P. 65–69. DOI:10.1038/s41591-018-0268-3.
- Shafiq G., Tatinati S., Ang W.T., Veluvolu K.C. Automatic Identification of Systolic Time Intervals in Seismocardiogram. Scientific Reports. 2016. № 6. 37524. DOI: 10.1038/srep37524.
- Ghongade R., Ratnaparkhi A., Joshi D. Artificial neural network based electrocardiogram pattern recognition. Third International Conference on Computational Intelligence and Information Technology (CIIT 2013). 2013. P. 305–312. DOI: 10.1049/cp.2013.2606.
- Gamage P.T., Azad M.K., Taebi A., Sandler R.H., Mansy H.A. Clustering SCG Events using Unsupervised Machine Learning. IEEE Signal Processing in Medicine and Biology Symposium (SPMB). 2018. P. 1–5. DOI: 10.1109/SPMB.2018.8615615.
- Solar B.E., Taebi A., Mansy H.A. Classification of Seismocardiographic Cycles into Lung Volume Phases. IEEE Signal Processing in Medicine and Biology Symposium (SPMB). 2017. P. 1–2. DOI: 10.1109/SPMB.2017.8257033.
- Zakeri V., Tavakolian K. Identification of Respiratory Phases Using Seismocardiogram: A Machine Learning Approach. Computing in Cardiology Conference (CinC). 2015. P. 305–308. DOI: 10.1109/CIC.2015.7408647.
- García-González M.A., Argelagós-Palau A., Fernández-Chimeno M., Ramos-Castro J.A. Comparison of heartbeat detectors for the seismocardiogram. Computing in Cardiology. 2013. № 40. P. 461–464.
- García-González M.A., Argelagós-Palau A., Fernández-Chimeno M., Ramos-Castro J. Differences in QRS Locations due to ECG Lead: Relationship with Breathing. XIII Mediterranean Conference on Medical and Biological Engineering and Computing. IFMBE Proceedings. 2014. V. 41. P. 962–964. DOI: 10.1007/978-3-319-00846-2_238.
- Goldberger A.L., Amaral L.A.N., Glass L., Hausdorff J.M., Ivanov P.Ch., Mark R.G., Mietus J.E., Moody G.B., Peng C.-K., Stanley H.E. PhysioBank, PhysioToolkit, and PhysioNet: Components of a New Research Resource for Complex Physiologic Signals. Circulation. 2003. № 101(23). P. e215–e220.
- Achildiev V.M., Soldatenkov V.A., Bedro N.A., Gruzevich Yu.K., Evseeva Yu.N., Levkovich A.D., Basarab M.A., Konnova N.S. Cardioseismometer unit based on micromechanical sensors. Proc. of 25th Anniversary Saint Petersburg International Conference on Integrated Navigation Systems. 2018. P. 272–281.
- Konnova N., Basarab M., Khachatryan M., Domracheva A., Ivanov I. Convolutional neural networks application in cardiovascular decision support systems. Proc. SPIE 11321. International Conference on Image and Video Processing, and Artificial Intelligence. 2019. DOI: 10.1117/12.2548193.
- Gaikwad K.M., Chavan M.S. Removal of high frequency noise from ECG signal using digital IIR Butterworth filter. IEEE Global Conference on Wireless Computing & Networking (GCWCN). 2014. P. 121–124. DOI: 10.1109/GCWCN.2014.7030861.
- Konnova N.S., Basarab M.A., Basarab D.A., Minin D.V., Achildiev V.M., Soldatenkov V.A., Bedro N.A., Gruzevich Yu.K., Evseeva Yu.N., Levkovich A.D., Komarova M.N. Preparation and application of electrosemocardiography data for diagnostics of the human cardiovascular system state. Journal Neurocomputers. 2019. № 1. P. 52–67.
- Mateo C., Talavera J. Short-Time Fourier Transform with the Window Size Fixed in the Frequency Domain. Digital Signal Processing. 2018. P. 13–21. DOI:10.1016/j.dsp.2017.11.003.
- Najmi A.-H., Sadowsky J., Morlet O. The Continuous Wavelet Transform and Variable Resolution Time-Frequency Analysis. Johns Hopkins apl technical digest. 1997. V. 18. № 1. P. 134–140.
- O’Toole J., Mesbah M., Boashash B. A Discrete Time and Frequency Wigner-Ville Distribution: properties and Implementation. 8th International Symposium on DSP and Communication Systems, Noosa, Queensland, Australia. 2005.
- Mann S., Haykin S. The chirplet transform: physical considerations. IEEE Transactions on Signal Processing. 1995. V. 43. № 11. P. 2745–2761. DOI:10.1109/78.482123.
- Vonesch C., Blu T., Unser M. Generalized Daubechies wavelet families. IEEE Transactions on Signal Processing. 1997. V. 55. № 9. P. 4415–4429.
- Pan J., Tompkins W.J. A real-time QRS detection algorithm. IEEE Transactions on Biomedical Engineering. 1985. V. BME-32. P. 230–236.
- Cunningham P., Delany S. k-Nearest neighbour classifiers. Multiple Classifier Systems. 2007. P. 1–17.
- Evgeniou T., Pontil M. Support Vector Machines: Theory and Applications. Machine Learning and Its Applications. 2001. LNCS. V. 2049. P. 249–257. DOI:10.1007/3-540-44673-7_12.
- Rokach L., Maimon O. Decision Trees. In: Maimon O., Rokach L. Data Mining and Knowledge Discovery Handbook. Springer, Boston, MA. 2005. P. 165–192. DOI:10.1007/0-387-25465-X_9.
- Chawla N., Eschrich S., Hall L. Creating Ensembles of Classifiers. IEEE International Conference on Data Mining. 2002. P. 580–581.
- Sherstinsky A. Fundamentals of Recurrent Neural Network and Long Short-Term Memory Network. 2018.
- Albawi S., Mohammed T.A., Al-Zawi S. Understanding of a convolutional neural network. International Conference on Engineering and Technology. 2017. P. 1–6. DOI:10.1109/ICEngTechnol.2017.8308186.
- Chinchor N. MUC-4 Evaluation Metrics. Proc. of the Fourth Message Understanding Conference. 1992. P. 22–29.
- Sasaki Y. The truth of the F-measure. Teach Tutor Mater. 2007. V. 1(5). P. 1–5.
- Powers D. Evaluation: From Precision, Recall and F-Factor to ROC. Informedness, Markedness & Correlation. Journal of Machine Learning Technologies. 2011. V. 2. Is. 1. P. 37–63. DOI:10.9735/2229-3981.
- Basarab M.A., Konnova N.S., Basarab D.A. Compression and Analysis of Ultrasonic Doppler Blood Flow Meter Data by the Discrete Chebyshev Transform. Proceedings of PIERS 2019 in Roma. 2019. In press.