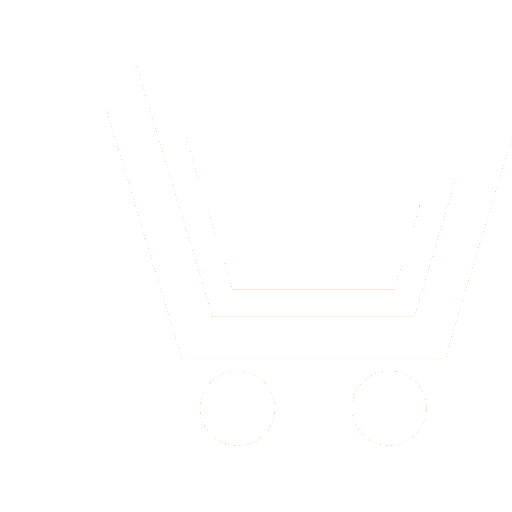
L.N. Anishchenko – Ph.D. (Eng.), Associate Professor, Senior Research Scientist, Biomedical Engineering Department, Research Section of scientific and educational complex “Fundamental Sciences”, Bauman Moscow State Technical University
E-mail: anishchenko@rslab.ru
A.V. Zhuravlev – Ph.D. (Phys.-Math.), Leading Research Scientist, Research Section of scientific and educational complex “Fundamental Sciences”, Bauman Moscow State Technical University
E-mail: azhuravlev@rslab.ru
V.V. Razevig – Ph.D. (Eng.), Senior Research Scientist, Research Section of scientific and educational complex “Fundamental Sciences”, Bauman Moscow State Technical University
E-mail: vrazevig@rslab.ru
M.A. Chizh – Ph.D. (Phys.-Math.), Junior Research Scientist, Research Section of Scientific and Educational Complex “Fundamental Sciences”, Bauman Moscow State Technical University
E-mail: mchizh@rslab.ru
The paper presents a multi-channel complex for non-contact movement detection, which consists of an RGB-D camera and bioradar sensors. Although the feasibility studies of recognition of different types of people’s movements by the help of RGB-D cameras as well as bioradars have been carried out for the past decades, the usage of such methods to detect aggressive behaviors has been mainly neglected. However, such methods may be extremely demanded in many surveillance scenarios (e.g. stadiums, railway stations, etc.). Moreover, the combined usage of both RGB-D sensors and bioradar has not been considered yet. As bioradars in the proposed complex, we used two monochromatic radars operating at 24.0 GHz and 24.4 GHz. As an RGB-D sensor IntelR RealSenseTM Depth Camera D435 was used. The software designed for collecting and processing data from all channels was written in Python 3.5. The proposed system was validated on an experimental dataset collected with participation of 10 volunteers (4 males and 6 females) in the age group between 19 and 22 years. All subjects gave their informed consent prior to the start of the experiments. During the experiments, the data from the RGB-D camera were recorded simultaneously with the signals from two bioradars. Each subject was asked to perform different types of common everyday motion patterns (going in and out of the observation zone, sitting/standing, whole body turning, raising hands, touching one's toes) and aggressive movements. Each motion pattern was repeated three times. The experimental data processing results have shown that when recognizing aggressive human behavior using the bioradar method, it is advisable to use a system of several bioradars, which will increase the accuracy, sensitivity and specificity of the classification to 86, 86, 87%, respectively. The proposed classification algorithm based on machine learning methods showed 98 % accuracy and Cohen's kappa of 78 % for the non-aggressive/aggressive behavior classification. The results should be accepted with caution because only data for young practically healthy examinees were used for the classifier training. Moreover, all movements were performed in the same surrounding conditions, and at the similar subject distance from the camera and bioradars. In future, we are planning to enrich the dataset considering different surroundings, lighting scenarios, viewing angles, subject distance to the bioradars. Furthermore, it is planned to use the modulated probing signal instead of monochromatic one, which allows estimating of the subject distance to be used to compensate the dependence of the bioradar signal amplitude on it.
- Anishchenko L., et. al. Application of step-frequency radars in medicine // Proceedings of SPIE 9077 Radar Sensor Technology XVIII. 2014. V. 90771N.
- Anishchenko L., Bechtel T., Ivashov S., Alekhin M., Tataraidze A., Vasiliev I. Bioradiolocation as a Technique for Remote Monitoring of Vital Signs // In: «Advanced Ultrawideband Radar: Signals, Targets and Applications». Taylor J.D. ed. CRC Press. 2016. P. 297–322.
- Kooij J.F.P., Liem M.C., Krijnders J.D., Andringa T.C., Gavrila D.M. Multi-modal human aggression detection // Computer Vision and Image Understanding. 2016. V. 144. P. 106–120.
- Bermejo Nievas E., Deniz Suarez O., Bueno Garca G., Sukthankar R. Violence Detection in Video Using Computer Vision Techniques // Berciano, A., et.al.(Eds.): Computer Analysis of Images and Patterns. CAIP 2011 // Lecture Notes in Computer Science. 2011.V. 6855. P. 332–339.
- Zizi T.K.T., et al. Aggressive movement detection using optical ow features base on digital & thermal camera // Proceedings of the 6th International Conference on Computing and Informatics, Kuala Lumpur, Malaysia. April 2017. P. 256–261.
- Rice D. Evaluating camera performance in challenging lighting situations // SDM Magazine, Sept., 2014.
- Wu Q., Zhang Y.D., Tao W., Amin M.G. Radar-based fall detection based on Doppler time-frequency signatures for assisted living // IET Radar, Sonar & Navigation, special issue on Application of Radar to Remote Patient Monitoring and Eldercare. 2015. V. 9. № 2. P. 164–172.
- Liang L., Popescu M., Skubic M., Rantz M., Yardibi T., Cuddihy P. Automatic fall detection based on doppler radar motion signature // Proceedings of the 5th International Conference on Pervasive Computing Technologies for Healthcare (PervasiveHealth). Dublin, Ireland. 2011. P. 222–225.
- Dremina M.K., Anishchenko L.N. Contactless fall detection by means of CW bio- radar // Proceedings of Progress in Electromagnetic Research Symposium (PIERS). Shanghai. China. August 2016. P. 2912–2915.
- Dremina M.K., Anishchenko L.N. Ispol'zovanie metoda bioradiolokacii dlya beskontaktnoj detekcii padenij // Biomedicinskaya radioelektronika. 2016. № 7. S. 50–55.
- Dremina M., Alborova I., Anishchenko L.N. Importance of the bioradar signal preprocessing in fall detection // 2017 Proceedings of Progress In Electromagnetics Research Symposium – Spring (PIERS), St.-Petersburg, Russia. 2017. P. 699–703.
- Anishchenko L., Alborova I., Dremina M. Discriminant Analysis in Bioradar-based Fall Event Classification // Proc. of Int. Conf. IEEE COMCAS. Tel Aviv, Israel, November 13–15. 2017. 4 p.
- Yang L., Li G., Ritchie M., Fioranelli F., Griths H. Gait classi_cation based on micro-Doppler features // Proceedings of 2016 CIE International Conference on Radar (RADAR). Guangzhou. China. October 2016. 1–4.
- Sun Z., Wang J., Sun J., Lei P. Parameter estimation method of walking human based on radar micro-Doppler // Proceedings of IEEE Radar Conference (RadarConf). Seattle WA. May 2017. P. 0567–0570.
- K-LC5 High Sensitivity Dual Channel Transceiver, URL: https://www.rfbeam.ch/product?id=9.
- SanPin 2.2.4/2.1.8.055-96, «Radiofrequency electromagnetic radiation under occupational and living conditions».
- https://click.intel.com/intelr-realsensetm-depth-camera-d435.html
- Jia Y., Shelhamer E., Donahue J., Karayev S., Long J., Girshick R., Guadarrama S., Darrell T. Caffe: Convolutional Architecture for Fast Feature Embedding. arXiv preprint arXiv:1408.5093, 2014.