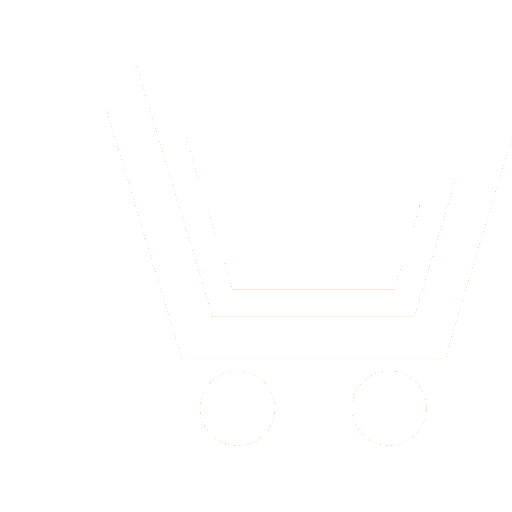
N.V. Gridina – Post-graduate Student, Department of Forensic Medicine, Sechenov First Moscow State Medical University; Design information technologies Center of RAS (Odintcovo, Moscow Region)
E-mail: info@ditc.ras.ru, nata_g_7@mail.ru
G.V. Zolotenkova – Ph. D. (Med.), Associate Professor, Sechenov First Moscow State Medical University; Design information technologies Center of RAS (Odintcovo, Moscow Region)
E-mail: info@ditc.ras.ru, zolotenkova.galina@bk.ru
A.I. Rogachev – Design information technologies Center of RAS (Odintcovo, Moscow Region)
E-mail: info@ditc.ras.ru
Introduction. Identification of human individuals is a fundamental, socially significant problem. On the first step in the forensic examination of an unknown person multiple regression equations are used in order to establish the biological age. This approach is not always efficient according to the standpoint of modern intelligent technologies. The authors propose using machine learning methods to improve the methodological approach to problem-solving process. The article presents the results of a comparative analysis of the application of various classification algorithms and evaluates the appropriateness of using them to solve specific problems of forensic medical examination.
Purpose. The analysis the possibilities of using machine learning methods in forensic medicine obtained comparing the most popular algorithms with unknown (unidentified) persons using various morphometric datasets.
Results. Based on a comparative analysis of the machine learning methods that are mostly used in medicine, the optimal ones for solving forensic problems were selected: Random Forest algorithm, as well as multilayer neural networks. Comparative experiments show that Random Forest has a sufficient degree of accuracy, are easily interpreted, correct for decision trees' habit of overfitting to their training sets. Neural networks, however, allow to achieve the highest accuracy score.
Practical significance. The result can be used as a justification for the choice of decision trees and neural networks as a basic classifier within the problem-solving process of biological age diagnosing of an unknown individual using modern information technologies.
- Identifikaciya lichnosti pri chrezvychajnyh proisshestviyah s massovymi chelovecheskimi zhertvami / Pod red. YU.I. Pigolkina. M. 2008. 235 s.
- Pigolkin YU.I., Nikolenko V.N, Zolotenkova G.V., Dallakyan V.F. Vozmozhnosti ispol'zovaniya morfologicheskih metodov ustanovleniya obshchih i specificheskih priznakov v sudebno-medicinskoj identifikacii lichnosti // Saratovskij nauchno-medicinskij zhurnal. 2012. T. 8. Vyp. 4. S. 900–905.
- Glybochko P.V., Pigolkin YU.I., Nikolenko V.N., Zolotenkova G.V., Efimova A.A., Alekseev YU.D., Fedulova M.V., Savenkova E.N., Kurzin M., Goncharova N.N., YUrchenko M.A., Miroshnichenko N.V. Sudebno-medicinskaya diagnostika vozrasta. M.: Pervyj MGMU imeni I.M. Sechenova. 2016. 318 s.
- Pigolkin YU.I., Tkachenko S.B., Zolotenkova G.V., Velenko P.S., Zolotenkov D.D., Safroneeva YU.L. Kompleksnaya ocenka vozrastnyh izmenenij kozhi // Sudebno-medicinskaya ekspertiza. 2018. T. 61. Vyp. 3. S. 15–18.
- Gridin V.N., Solodovnikov V.I., Evdokimov I.A., Filippkov S.V. Postroenie derev'ev reshenij i izvlechenie pravil iz obuchennyh nejronnyh setej // Iskusstvennyj intellekt i prinyatie reshenij. 2013. № 4. S. 6–33.
- Gridin V.N., Solodovnikov V.I. Osobennosti vnutrennego predstavleniya i vizualizacii izvlekaemoj iz dannyh informacii s ispol'zovaniem modul'noj nejronnoj seti bp-som // Novye informacionnye tekhnologii v avtomatizirovannyh sistemah. 2017. № 20. S. 170–175.
- Waaijer M.E.C., Gunn D.A., Catt S.D. Morphometric skin characteristics dependent on chronological and biological age: The Leiden Longevity Study // AGE. 2013. V. 34. Is. 6. P. 1543–1552.
- Habtamu M.A., Joon-Kyung S., Jeong-Hyeon S. Biological Brain Age Prediction Using Cortical Thickness Data: A Large Scale Cohort Study // Front. AgingNeurosci. 2018.
- Heath R.P., Kuzniecky R. NAPR: a Cloud-Based Framework for Neuroanatomical Age Prediction // Neuroinformatics. 2018. V. 16. Is. 1. P. 43–49.
- Mamoshina P., Kochetov K., Cortese F., Aliper A. et al. Population Specific Biomarkers of Human Aging: A Big Data Study Using South Korean, Canadian, and Eastern European Patient Populations // The Journals of Gerontology Series A Biological Sciences and Medical Sciences. 2018. V. 73(11).
- Arkad'ev A.G., Braverman E.M. Obuchenie mashiny raspoznavaniyu obrazov. M.: Nauka. 1964. 110 s.
- Rish I. An empirical study of the naive Bayes classifier // IJCAI 2001 Workshop on Empirical Methods in Artificial Intelligence.
- Statnikov A., Aliferis C., Hardin D. A Gentle Introduction to Support Vector Machines in Biomedicine: Theory and methods. World Scientific. 2011.
- Hajkin S. Nejronnye seti: polnyj kurs. M.: Vil'yams. 2006. 1104 s.
- Quinlan J.R. Induction of Decision Trees. MachineLearning. 1986. № 1. 81–106.
- Hastie T., Tibshirani R., Friedman J. Chapter 15. Random Forests // The Elements of Statistical Learning: Data Mining, Inference, and Prediction. 2nd ed. Springer–Verlag. 2009.
- Riley R.D., Ahmed I., Debray T.P., Willis B.H., Noordzij P., Higgins J.P., Deeks J.J., Summarising and validating test accuracy results across multiple studies for use in clinical practice // Statistics in Medicine. 2015. V. 34 (13).