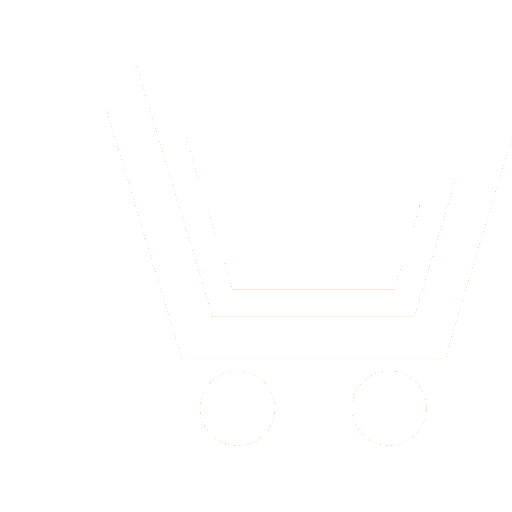
A.P. Kazantsev – Ph.D. (Eng.), Associate Professor, Pushchino State Institute of Natural Sciences; Senior Research Scientist, Institute of Biological Instrumentation of the Russian Academy of Sciences, Acting Head of the laboratory of Biomedical Research
E-mail: telemed.ak@gmail.com
N.S. Minaev – Bachelor of Economics, Engineer, Institute of Biological Instrumentation of the Russian Academy of Sciences, Post-graduate Student, Pushchino State Institute of Natural Sciences
E-mail: st.denko@rambler.ru
I.S. Minaev – Master’s Degree in Biomedical Engineering; Engineer, Institute of Biological Instrumentation of the Russian Academy of Sciences – Separate Union of Federal Research Center Pushchino Scientific Center for Biological Research of the Russian Academy of Sciences
E-mail: st.denko@rambler.ru
L.A. Cherkashina – Bachelor of Biotechnology; Engineer, Institute of Biological Instrumentation of the Russian Academy of Sciences – Separate Union of Federal Research Center Pushchino Scientific Center for Biological Research of the Russian Academy of Sciences, Graduate Student of the Pushchino State Institute of Natural Sciences
E-mail: anarore-kriptih@yandex.ru
The paradigm of personalized and preventive medicine rises new demands to medical technologies i.e. to the remote monitoring of a fetal state. The paper is aimed at the feasibility evaluation of automated fetal intracardiac bloodflow sonography making use of an off-the-shelf handheld monitor.
Doppler ultrasonography plays a principle role in the assessment of a fetal condition. A fetal Doppler acquires sonography signals that carries out the information of bloodflow rate and the velocity of formal elements. The chirplet transform of a sonographic signal produces the series of elementary amplitude- and frequency-modulated functions named chirplets. Reverse composition of the elementary functions assembles back the original signal. Very useful feature of a chirplet is being a container of a Doppler shift and intensity of echo for an imaginary drop of blood moving within bloodflow. The chirplet basis allows to consider the entire bloodflow as a large set of blood drops and brings the simulation model of volumetric bloodflow expressed as bipolar profile. The sonographic signal representing a single cardiac cycle breaks into the series of phases marked with key events such as opening/closing of heart valves and atrial contractions. The events recognition helps to accurately locate the certain drops representing unique bloodflow pulses through heart valves. In the case of atrioventricular valves this assembly model suggests an effective algorithm of diastolic ratio computation. To provide the accurate location of the events the neural network of two convolutional layers followed by one fully connected classifier was developed and trained on the data sets of key events copied with random variation of their intrincic parameters.
- A mathematic simulation model of fetal intracardiac bloodflow is suggested.
- A convolutional neural network for cardiac cycle key events location is developed.
- Ventricular diastolic indices calculated on a test data set fell in the low hypoxia state limits in agreement with the independent diagnosis of chronical placental insufficiency.
The feasibility of automated fetal intracardiac bloodflow sonography backed by computational intelligence is demonstrated. The approach should meet with high acceptance in fetal monitoring technology.
- Kazancev A.P. Malobyudzhetnoe monitorirovanie ploda v sisteme s vychislitel'nym intellektom dlya massovogo telemedicinskogo obsluzhivaniya beremennyh // LAP Lambert Academic Publishing, 2018. 56 s.
- Mann S., Haykin S. The chirplet transform: Physical considerations // In IEEE Trans. Signal Processing. 1995. V. 43. P. 2745–2461.
- Rybakova M.K., Mit'kov V.V. Ekhokardiografiya v tablicah i skhemah. Nastol'nyj spravochnik M.: VIDAR. 2011. 288 s.
- Nikolenko S., Kadurin A., Arhangel'skaya E. Glubokoe obuchenie. SPb.: Piter. 2018. 480 s. (Seriya «Biblioteka programmista»).
- Haykin S. Neural Networks: A Comprehensive Foundation. 2nd Edition. Prentice-Hall. 1999. 842 p.
- Ageeva M.I. Doplerograficheskoe issledovanie vnutriserdechnoj gemodinamiki ploda pri fiziologicheskom ego razvitii vo II–III trimestrah beremennosti // Ul'trazvukovaya i funkcional'naya diagnostika. 2005. № 3. S. 11–20.