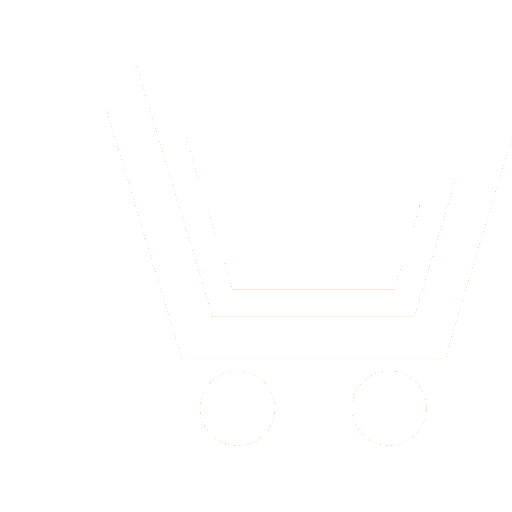
N.A. Al-Khulaidi, A.A. Sallam, A.R.A. Abdulraqeb, L.T. Sushkova
Blood pressure signals are rich with pathological information that can be used in the diagnostic of the cardiovascular system of the body. Tin this work, was compared the blood pressure signals classification by using Radial Basis Function Neural Network (RBFNN) and Multilayer Perceptron Neural Network (MLPNN). The best results of classified blood pressure signals for both neural networks were compared based on different parameters. The number of neurons in the hidden layer for MLPNN selected as significant parameter. This parameter was investigated along with fixed number of output and input nods. While, the value of spread parameter selected as significant parameter for RBFNN. The way, that the optimum number of this parameter was found, is by investigating different RBFNN with various values of spread parameter. Sensitivity, specificity and accuracy were used as criteria for evaluating the results of the classification of blood pressure signals. The results of a comparative analysis of the classification of blood pressure signals showed that the application of RBS exceeds the MP by 4.3% for sensitivity, 1.8% for specificity and 2.2% for accuracy.
- Qiao Zhang. Cuff-free blood pressure estimation using signal processing techniques. College of Graduate Studies and Research in Partial Fulfillment of the Requirements for the degree of Master of Science in the Division of Biomedical Engineering, University of Saskatchewan Saskatoon. 2010. 25 p.
- Bojanov G. Handbook of Cardiac Anatomy, Physiology, and Devices/ Iaizzo P.A., Ed. Totowa, NJ: Humana. 2005. 320 p.
- Radian Belu. Artificial Intelligence Techniques for Solar Energy and Photovoltaic Applications. Drexel University, USA & Desert Research Institute. 2013. 658 p.
- Jeff Heaton. Introduction to Neural Networks for C#, 2nd Edition Paperback. Heaton Research, Inc. St. Louis. 2008. 450 p.
- Tonekabonipour H., Emam A., Teshnelab M., Shoorehdeli M. A. Ischemia prediction via ECG using MLP and RBF predictors with ANFIS classifiers epileptic seizure prediction using ANN // for. Conference: ISSD'12, Third International Symposium on Sustainable Development, At Sarajevo, Bosnia and Herzegovina. P. 491–498.
- Kevric J., Subasi A. Classification of EEG signals for epileptic seizure prediction using ANN // Conference: ISSD'12, Third International Symposium on Sustainable Development, At Sarajevo, Bosnia and Herzegovina. 2012. P. 491–498.
- Mai V., Khalil I., Meli C. ECG biometric using multilayer perceptron and radial basis function neural network // Conf Proc IEEE Eng Med Biol Soc. 2011. P. 2745–2748.
- Javad K., Boostani R., Dehghani P., Nowroozipour F., Saki N. Prediction of acute myocardial infarction with artificial neural networks in patients with nondiagnostic electrocardiogram // Journal of Cardiovascular Disease Research. 2015. P. 51–59.
- Rajesh G., Ghato A. An Effective Feature Set for ECG Pattern Classification. Medical Biometrics // First International Conference ICMB. 2008. P. 25–32.
- Mohamed M. Neuronal Classification of Atrial Fibrillation // Leonardo Journal of Science. 2008. Is. 12. P. 196–213.
- Zhang Q.J., Gupta K.C. Neural Networks for RF and microwave Design. Norwood, MA: Artech House, USA. 2000. 695 p.
- Subana S., Sandhy S. Artificial Neural Network Modelling. Springer. 1st ed. 2016. 1200 p.
- web page http://www.physionet.org/physiobank date of access [01.07.2018]
- Inspection and Palpation of Venous and Arterial Pulses. Examination of the Heart Part 2. American Heart Associations, Fighting Heart Disease and Stroke.
- Walker H.K., Hall W.D., Hurst J.W. Clinical Methods: The History, Physical, and Laboratory Examinations // 3rd edition. Emory University School of Medicine, Atlanta, Georgia. Boston: Butterworths. 1990. P. 1320–1345.
- Kumar P., Clark’s M. Clinical Medicine. 7th ed. Elsevier Saunders. 2009. 812 p.
- Pijush S., Sanjiban S., Valentina E. Sanjiban Sekhar and Valentina E. Balas. Handbook of Neural Computation. 1st Edition.Elsevire. 2017. 658 p.
- Ke-Lin Du, Swamy M.N.S. Neural Networks and Statistical Learning // Springer. 2014. P. 950–967.