350 rub
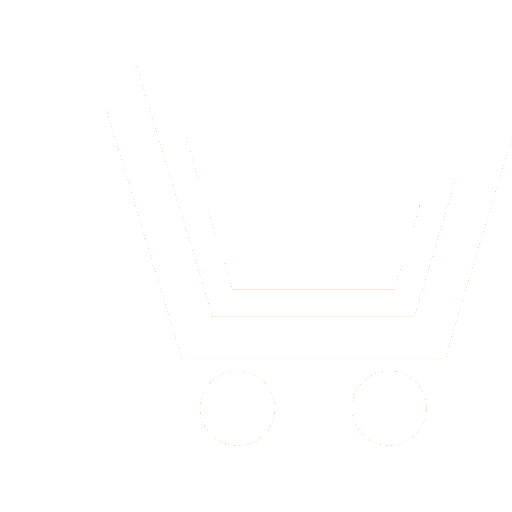
Journal Biomedical Radioelectronics №3 for 2017 г.
Article in number:
Advanced methods for thermal images processing for medical applications
Authors:
I.S. Kozhevnikova - Ph.D. (Biol.), Senior Research Scientist, Institute for Biomedical Research of Northern (Arctic) Federal University named after M.V. Lomonosov, Arkhangelsk
E-mail: kogevnikovais@yandex.ru, i.s.kozhevnikova@narfu.ru
N.A. Ermoshina - Bachelor of Information Systems and Technology of the Baltic State Technical University "VOENMEKH" named after D.F. Ustinov, St.-Petersburg
E-mail: ermoshina.n@gmail.com
M.N. Pankov - Ph.D. (Med.), Associate Professor, Deputy Director, Institute for Biomedical Research of Northern (Arctic) Federal University named after M.V. Lomonosov, Arkhangelsk
E-mail: m.pankov@narfu.ru
Abstract:
The systematic review on major achievements in computer aided interpretation of thermogram aims to provide a comprehensive survey of the latest CADx models developments and applications.
Thermography can be used to identify and analyze thermal anomalies; it provides useful diagnostic and therapeutic information however the interpretation of thermograms remains a laborious task prone to errors due to visual ambiguity. The temperature pattern of disease especially on early stages can be subtle, nonlinear and invisible. Human eye can capture only a fraction of information because of the limitations of the visual system. Therefore the demand for a proper computer aided thermograms processing and interpretation method is still an open discussion. The CADx development brings additional value helping to overcome subjectivity by providing second opinion and improve reliability of thermography as diagnostic and screening method.
The paper briefly outlines the main concepts including some fundamental techniques used in CADx systems and provides the detailed investigation and thorough discussion of reported performance of surveyed systems. Computer algorithms involved in theCADx scheme include four steps: image pre-processing for noise reduction and removal of artifacts, defining regions of interest/segmentation and classification. These algorithms come from the domains of image processing, pattern recognition and machine learning. Research in image processing incorporated with an intelligent system not only provides a solution to identify, classify, and quantify disease patterns from images, but it is likely going to change the way we draw inference from image data.
We discuss benefits and drawbacks of methods offering several possible future research directions. In recent years, there have been significant results achieved in CADx models development in terms of accuracy, specifity and senility. These results were possible mainly due to recent sensor improvements, falling costs and advances in images processing techniques and data analysis. Brest cancer diagnosis is the most developed field of research. As the classification accuracy approaches 100%, the proposed methods are able to automatically segment ROIs with the acceptable precision the problems of relatively high rates of false-positive and false-results is still far from being solved. The most promising research areas address such problems as thermal images sequence (dynamic thermography) analysis and interpretation and offers methods to separate cancerous tissue in thermal images, classify benign and malignant cancer. Various attempts in other field of medicine have been made to develop a CADx. However the majority of described systems is fragmented and focuses on one or several aspects within the CADx development cycle. Describing, for example, the feature extractions and selection procedures they leave the segmentation to be performed manually. There is a lack of full cycle models developed and described.
Pages: 22-31
References
- Basha N.S., SHulga L.A. Sistema vydelenija podkozhnogo risunka krovenosnogo risunka po termograficheskim izobrazhenijam // Vestnik MGTU im. N.EH. Baumana. Ser. Estestvennye nauki. 2012. № 2. S. 98-106.
- Chao Jin, Yang Yang, Zu Jun Xae, Ke-Min Liu, Jing Liu Automated Analysis Method for Screening Osteoarthritis using Medical Infrared thermography // Journal of Medical and Biological Engineering. 2012. № 33(5). P. 471-477.
- Dinsha D., Manikandaprabu N. Breast tumor segmentation and classification using SVM and Bayesian from thermogram images // Unique journal of engineering and advanced sciences. 2014. № 2. P. 147-51.
- Etehad Tavakol M., Sadri S., Ng E. Y. K. Application of K and fuzzy c-means for color segmentation of thermal infrared breast images // Journal of Medical Systems. 2010. V. 34. № 1. P. 35-42.
- Frize M. Ogungbemile A. Estimating Rheumatoid Arthritis Activity with Infrared Image Analysis // Studies in Health Technology and Informatics IOS Press. 2012. V. 180. R. 594-598.
- Golestani N., M Etehad Tavakol., Ng E. Y. K. Level set method for segmentation of infrared breast thermograms // EXCLI Journal. 2014. № 13. P. 241-251.
- Gopinath M. P., Prabu S. Classification of thyroid abnormalities on thermal image: a study and approach // Institute of Integrative Omics and Applied Biotechnology. 2016. V. 7. P. 41-57.
- Harlick R. M., Shanmugam K. Textural Features for Image Classification // IEEE transactions on Systems, Man and Cybernetics. 1973. V. 3. P. 610-621.
- Kazerouni A. Zadeh H.G., Haddadnia J. Novel Model for Smart Breast Cancer Detection in Thermogram Images // Asian Pacific Journal of Cancer Prevention. 2014. № 15 (24). P. 73-76.
- Krawczyk B., Woźniak M., Schaefer G. Cost-sensitive decision tree ensembles for effective imbalanced classification // Applied Soft Computing. 2012. V. 14. C. P. 554-562.
- Lashkari A., Pak F., Firouzmand M. Full Intelligent Cancer Classification of Thermal Breast Images to Assist Physician in Clinical Diagnostic Applications // Journal of Medical Signals and Sensors. 2016. № 6. P. 12-24.
- Lin Chih-Lung An Approach to Improve the Quality of Infrared Images of Vein-Patterns // Sensors. 2011. № 11. P. 11447-11463.
- Marzec M., Koprowski R., Wróbel Z. Automatic method for detection of characteristic areas in thermal face images // Multimed Tools Appl. 2015. № 74. P. 4351-4368.
- Milosevic M., Jankovic D., Peulic A. Thermography basedbreast cancer detection usingtexture features and minimum variance quantization // EXCLI Journal. 2014. № 13. P. 1204-1215.
- Mona A. S. Ali, Gehad I. S., Tarek G., Aboul E. H., Vaclav S., Lincoln F. Silv Detection of Breast Abnormalities of Thermograms based on a New Segmentation Method // Proceedings of the Federated Conference on Computer Science and Information Systems. 2015. P. 255-261.
- Mrinal K. B., Shawli B., Kakali D., Debotosh B., Satyabrata N. Pain Related Inflammation Analysis using Infrared Images // Thermosense: Thermal Infrared Applications XXXVIII, 986116. Proc. SPIE 9861. 2016. P. 1417-1422.
- Muthu R., Mookiah U., Acharya R., Ng E.Y.K. Data mining technique for breast cancer detection in thermograms using hybrid feature extraction strategy // Quant itative InfraRed Thermography Journal. 2012. № 9(2). P.151-165.
- Negin M, Ziskin M.C., Piner C., Lapayowker M.S. A computerized breast thermographic interpreter. // IEEE Transactions on Biomedical Engineering. 1977. № 24. P. 347-352.
- Nizami M. Segmentation of Thermal Images for Evaluation of Rheumatoid Arthritis Disease // International Journal of Emerging Engineering Research and Technology. 2014. V. 2. P. 35-44.
- Qi H., Kuruganti P.T., Snyder W.E. Detecting breast cancer from thermal infrared images by asymmetry analysis // Biomedical Engineering Handbook. CRC Press. 2006. Chapters.
- Savari A., Julian S. Brest cancer detection on thermogram at preliminary stage by using fuzzy inferences systems // Journal of Theoretical and Applied Information Technology 2014. V. 68. № 3. P. 705-715.
- Silva L., Saade D.S. M., Sequeiros G.O. O., Silva A., Paiva A., Bravo R.S., Conci A. A new database for breast research with infrared image // Journal of Medical Imaging and Health Informatics. 2014. V. 4. № 1. P. 92-100.
- Sudhir R., Bhalerao S.V. Designing of Neuro-Fuzzy Based Thermal Imaging System on MATLAB for Detection of Rheumatoid // Arthritis International Journal of Engineering Research and General Science. 2015. V. 3. P. 592-596.
- Suganthi S., Ramakrishnan S. Semi automatic segmentation of breast thermograms using variational level set method // The 15th International Conference on Biomedical Engineering. Springer. 2014. P. 231-234.
- Swaminathan R., Suganthi S.S. Segmentation of breast tissues in infrared images using modified phase based level sets // Biomedical Informatics and Technology. Springer. 2014. P. 161-174.
- Tang X., Lu H., Ma S. Face recognition using kernel scatter-difference-based discriminant analysis. // IEEE Transactions Neural Network. 2006. № 17(4). P. 1081-1085.
- Zadeh H.J, Haddadnia J., Montazeria A. Model for Diagnosing Breast Cancerous Tissue from Thermal Images Using Active Contour and Lyapunov Exponent // Iran J Public Healt. 2016.V. 45. № 5. P. 657-669.
- Zadeh H. G., Haddadnia J. Distinguishing breast cancer based on thermal features in infrared images //Canadian Journal on Image Processing and Computer Vision. 2011. № 2(6). P. 54-8.