350 rub
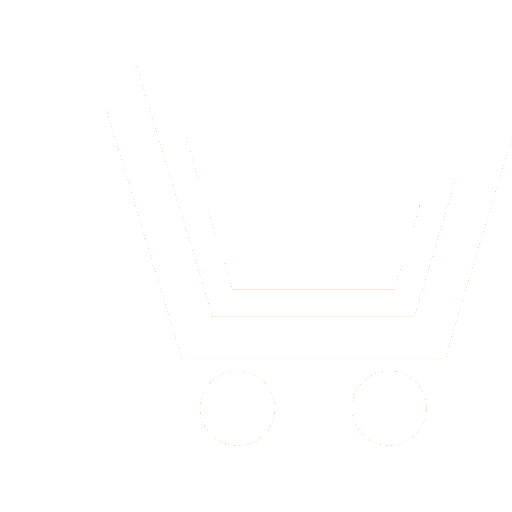
Journal Biomedical Radioelectronics №7 for 2016 г.
Article in number:
Bioradiolocation in contactless fall detection
Authors:
M.K. Dremina - Master Degree Student, Biomedical Engineering Department, Bauman Moscow State Technical University
E-mail: mary.dremina@student.bmstu.ru
L.N. Anishchenko - Ph.D. (Eng.), Associate Professor, Senior Research Scientist, Research Section of Scientific and Educational Complex «Basic Sciences», Bauman Moscow State Technical University
E-mail: anishchenko@rslab.ru
Abstract:
The elderly population in the world has been continuously increasing. Every day about 200 thousand people celebrate their sixtieth birthday, all around the world, and that number will grow [1]. The aging process is accompanied by degradation of extremity function in the elderly population, which can restrict their daily quality of life and gradually increase the fall risk. Falls significantly affect morbidity and quality of life as well as health care costs among the aging population [4]. One determining factor influencing the severity of fall consequences in older people is the amount of time spent lying on the floor or ground. Falls are particularly critical when the elderly person is injured and cannot call for help. Immediate help in such cases is a key thing for the successful recovery and returning to the natural life rhythm. Therefore, in recent years, more and more scientists have been paying attention to question of developing effective fall detection system [5-13].
There is a variety of wearable devices for motion activity tracking and fall detection based on movement sensors (e.g., accelerometers and gyroscopes) in the market. However, wearable devices are not always comfortable to use, and suffer from a significant false-positive rate. Current approaches for remote fall detection are based on video cameras technologies for which walls and fabrics became optically opaque obstacle, and they are sensitive to lighting conditions [6, 7].
This paper presents the results of studies carried out to confirm the possibility of using bioradar for the fall detection. An exploration of multi-frequency system advantages of physical activity monitoring over a single frequency system were considered. Furthermore, an effectiveness developing classifier based on time domain parameters was studied. Two machine learning algorithms were compared: the support vector machines and the method k - nearest neighbors. Four classifiers were developed and tested on a set of various basic movements that were recorded by the (CW) radar: BioRASCAN-4 designed at Bauman Moscow State Technical University. It operates at probing frequencies of 3.6 to 4.0 GHz. The dataset was obtained by experiments for 7 different motion patterns, including forward falling, backward falling, sitting and standing, turning 90 and 180 degrees, arm motion, going in and out of the location zone.
Pages: 49-55
References
- United Nations, Department of Economic and Social Affairs, Population Division (2013). World Population Prospects: The 2012 Revision, CD-ROM Edition.
- Federalnaja sluzhba gosudarstvennojj statistiki (Rosstat) «CHislennost naselenija po subektam Rossijjskojj federacii». Doklad ot 26.02.2016 g
- Djomin A.V., Moroz T.P. Osobennosti posturalnojj nestabilnosti i faktorov riska padenijj u lic pozhilogo i starcheskogo vozrasta (literaturnyjj obzor) // Molodojj uchenyjj. 2014. № 3. S. 164-175.
- Todd C., Skelton D. What are the main risk factors for falls among older people and what are the most effective interventions to prevent these falls - Copenhagen, WHO Regional Office for Europe (Health Evidence Network report. 2004; http://www.euro.who.int/document/E82552.pdf, accessed 5 April 2004).
- Gjoreski M., Gjoreski H., Lustrek M. How Accurately Can Your Wrist Device Recognize Daily Activities and Detect Falls - // Sensors. 2016. № 16. R. 800.
- Wang X., Gao C., Guo Y. Elderly fall detection using SIFT hybrid features // Published in Proceedings. V. 9675: AOPC 2015: Image Processing and Analysis. October 2015.
- Mundher Z.A. A Real-Time Fall Detection System in Elderly Care Using Mobile Robot and Kinect Sensor // International Journal of Materials, Mechanics and Manufacturing. May 2014. V. 2. № 2.
- Chuah Y., Lee J. Fall Detection of Elderly People in Bathroom: A Complement Method of Wearable Device // International Journal of Applied Engineering Research. 2016. V. 11. № 6. R. 4184-4186.
- Anishchenko L., Alekhin M., Tataraidze A., Ivashov S., Bugaev A., Soldovieri F. Application of step-frequency radars in medicine // Proc. SPIE 9077. Radar Sensor Technology May 29, 2014. № XVIII. R. 90771N.
- Wu Q., Zhang Y.D., Tao W., Amin M.G. Radar-based fall detection based on Doppler time-frequency signatures for assisted living // IET Radar, Sonar & Navigation. Special issue on Application of Radar to Remote Patient Monitoring and Eldercare. Feb. 2015. V. 9. № 2. R. 164-172.
- Liang Sjc L., Popescu M., Skubic M., Rantz M., Yardibi T., Cuddihy P. Automatic fall detection based on doppler radar motion signature // in Proceedings of the 5th International Conference on Pervasive Computing Technologies for Healthcare (PervasiveHealth). 2011. R. 222-225.
- Karsmakers D.P., Croonenborghs T., Mercuri M., Schreurs D., Leroux P. Automatic in-door fall detection based on microwave radar measurements // in Proceedings of the 9th European Radar Conference. 2012. R. 202-205.
- Tivive C., Bouzerdoum A., Amin M.G. A human gait classification method based on radar Doppler spectrograms // EURASIP J. Adv. Sig. Proc. 2010. V. 2010. R. 1-12.
- Kohavi R. A Study of Cross-Validation and Bootstrap for Accuracy Estimation and Model Selection // International Joint Conference on Artificial Intelligence. 1995. № 14. R. 1137-1145.
- Aha D., Kibler D., Instance-based learning algorithms // Mach. Learn. 1991. № 6. R. 37-66.
- Cristianini N., Shawe-Taylor J. An Introduction to Support Vector Machines and Other Kernel-Based Learning Methods // Cambridge University Press: Cambridge, UK. 2000.
- Hastie, T., Tibshirani, R., Friedman J. The Elements of Statistical Learning, 2nd edition. Springer. 2009. 533 p.