350 rub
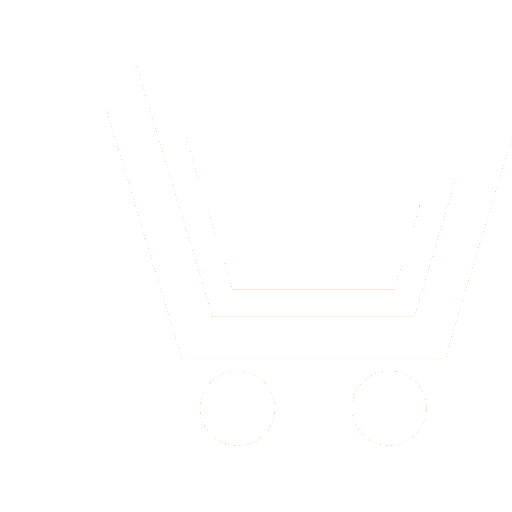
Journal Biomedical Radioelectronics №2 for 2016 г.
Article in number:
Models of Gaussian mixture distributions of the EEG signal wavelet spectrum
Authors:
K. O. Ivanov - Post-graduate Student, Volga State University of Technology, Yoshkar-Ola, Russia
V.V. Sevastyanov - Dr.Sc. (Med.), Professor, Volga State University of Technology, Head of the Center for Speech Pathology and Neuro-Rehabilitation, Yoshkar-Ola, Russia
S.N. Svintsov - Neurologist of Highest Medical Category, The Republican Clinical Hospital, Yoshkar-Ola, Russia
Abstract:
The paper reveals the relevance of the development of systems for automatic classification of EEG phenomena. The advantages of using a discrete wavelet transform to create a feature space for classifying EEG signal phenomena are noted. A brief overview of the existing methods of EEG patterns recognition by using the wavelet spectrum of the signal is presented; shortcomings of existing approaches are identified, in particular, the reduction of time resolution due to the use of averaged characteristics of the signal.
The study aims to develop a new method of EEG signal recognition which will be based on the wavelet spectrum of the signal and will allow avoiding degradation of time resolution. The task was accomplished through the use of Gaussian mixture models (GMM) of wavelet spectrum of the signal. Multiresolution fast discrete transform by Mallat algorithm was implemented for signal transform in this study. The analyzed signals were produced by a digital electroencephalograph with the sampling rate of 500 Hz. The study showed that eleven levels of wavelet decomposition are necessary to analyze the signal provided that the frequency range of the VOI process is from 0.35 to 40 Hz, and its sampling rate equals 500 Hz. The wavelet spectrum of the signal was then decomposed into several Sub-bands, each responsible for exhibiting a specific EEG phenomenon, such as alpha rhythm, beta rhythm, and epileptic spike-and-slow wave complex. Each area of the EEG signal wavelet spectrum was represented as a GMM model. The parameters of the GMM models were identified by using the EM algorithm. Each GMM model included five mixtures of 11D Gaussian distributions. If each random digit of the EEG signal is represented by an 11D vector from its wavelet spectrum, and if the probabilistic models of the EEG phenomena are known, the random digit of the EEG signal can be categorized on the basis of the maximum likelihood method, i.e. the random digit will be referred to the GMM model for which the log-likelihood function value of wavelet coefficients of the EEG sample and the model parameters is higher than for the other models. In order to test the efficiency of the proposed approach, an experiment was carried out, in which a recording of EEGs in a patient with epilepsy was done during seven minutes. After the signal was then decomposed through the use of the Daubechies D4 wavelet transform, and respective GMM models of its wavelet spectrum were obtained, all epileptic complexes and alpha rhythm areas were recognised correctly. Thus, the use of GMM models of EEG phenomena wavelet spectra allows classifying the EEG signal into components of norm and pathology, with good localization of different patterns in time.
Pages: 46-52
References
- Zenkov L.R. Klinicheskaja ehlektroehncefalografija (s ehlementami ehpileptologii): Rukovodstvo dlja vrachejj. Izd. 5-e. M.: MEDpress-inform. 2012. 356 s.
- Pavlov A.N., KHramov A.E., Koronovskijj A.A., Sitnikova E.JU., Makarov V.A., Ovchinnikov A.A. Vejjvlet-analiz v nejjrodinamike // Uspekhi fizicheskikh nauk. 2012. T. 182. № 9. S. 905-939.
- Jensen A., la Cour-Harbo A. Ripples in mathematics: the discrete wavelet transform. Springer Science & Business Media. 2001.
- Benedetto J.J., Pfander G.E. Wavelet detection of periodic behavior in EEG and ECoG data // 15th IMACS World Congress. Berlin. 1997. T. 1.
- Zhang Z., Kawabata H., Liu Z.Q. Electroencephalogram analysis using fast wavelet transform // Computers in biology and medicine. 2001. V. 31. №. 6. P. 429-440.
- Akareddy S.M., Kulkarni P.K. EEG signal classification for epilepsy seizure detection using improved approximate entropy // International Journal of Public Health Science (IJPHS). 2013. V. 2. №. 1. P. 23-32.
- Shoeb A.H., Guttag J.V. Application of machine learning to epileptic seizure detection //Proceedings of the 27th International Conference on Machine Learning (ICML-10). 2010. P. 975-982.
- Omerhodzic I., et al. Energy distribution of EEG signals: EEG signal wavelet-neural network classifier // arXiv preprint arXiv:1307.7897. 2013.
- Bilmes J.A., et al. A gentle tutorial of the EM algorithm and its application to parameter estimation for Gaussian mixture and hidden Markov models // International Computer Science Institute. 1998. V. 4. № 510. R. 126.
- Mallat S. Multiresolution approximation and wavelet orthonormal basis of L^2(R) // Trans. AMS. 1989. V. 315. P. 69-87.
- Adeli H., Ghosh-Dastidar S. Automated EEG-based diagnosis of neurological disorders: Inventing the future of neurology. CRC Press. 2010.
- Dobeshi I. Desjat lekcijj po vejjvletam. M.: NIC «Reguljarnaja i khaoticheskaja dinamika». 2001.
- Smolencev N.K. Osnovy teorii vejjvletov. Vejjvlety v MATLAB. M.: DMK Press. 2005. T. 304. S. 11.
- Huang X., et al. Spoken language processing: A guide to theory, algorithm, and system development. Prentice Hall PTR. 2001.