350 rub
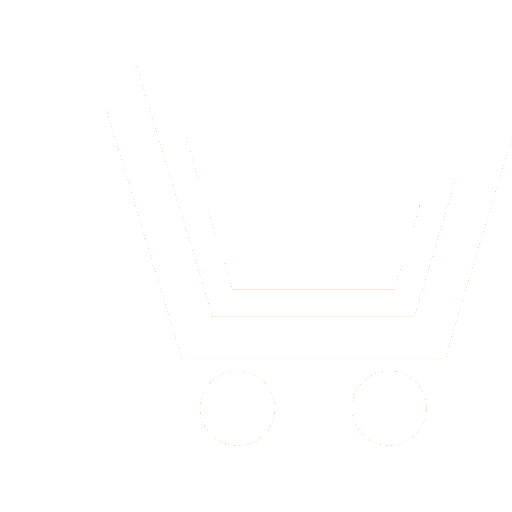
Journal Biomedical Radioelectronics №6 for 2013 г.
Article in number:
Estimation of classifiers generalization ability in solving problem of sleep stage recognition by using heart rate variability
Keywords:
REM sleep
non-REM sleep
wakefulness
heart rate variability
kNN
random forest
rotation forest
AdaBoost
Authors:
A.B. Tataraidze, L.N. Anishchenko, M.D. Alekhin
Abstract:
A comparative analysis of generalization ability of classifiers in the task of automated detection of REM sleep, non-REM sleep and wakefulness on the base of standard time and spectral characteristics of heart rate variability (HRV) was performed.
In the study experimental database of HRV signals collected during night polysomnography monitoring for 24 male subjects aged 44,7 ± 8,1 years with sleep apnea-hypopnea index of 35,08 ± 23,8 was used. The feature space for analyzed 30-second epochs of sleep was formed applying the following standard HRV characteristics: AVNN, SDNN, rMSSD, pNN50, TP,ULF, VLF, LF, HF, LF/HF, ULF%, VLF%, LF%, HF%. Every time the calculations of feature vectors components were carried out applying 5-minute moving window with a fixed step of 30 seconds. The analyzed epoch of sleep, for which feature extraction was performed, was located in the center of the moving window.
For automated detection of sleep structure on the base of time and spectral characteristics of HRV classification algorithms implemented in WEKA and Orange libraries were used. The best recognition accuracy of 83,8±0,4% and level of agreement at Cohen-s kappa value of 0,66±0,01 were achieved for the set of the following algorithms: kNN; Random Forest; Rotation Forest; AdaBoost.
It should be pointed out that while estimating generalization ability of classifiers in the task of automated detection of sleep structure on the base HRV characteristics by comparing recognition accuracy with corresponding values for «gold standard» diagnostics in sleep medicine (polysomnography, PSG) the results of interpretation of PSG records by different medical experts may have significant differences. This fact may affects the quality of calculated estimates.
Thus, the accuracy value of 83,8±0,4% for recognition of sleep stages should be considered as satisfactory for medical diagnostics tasks and Cohen-s kappa value of 0,66±0,01 reveals acceptable agreement of results obtained from PSG records by medical experts and automated detection of sleep structure on the base of time and spectral characteristics of HRV.
Pages: 44-51
References
- Van de Water A., Holmes A., Hurley D. Objective measurements of sleep for non-laboratory settings as alternatives to polysomnography - a systematic review // Journal of sleep research. 2011. V. 20. № 1. P. 183-200.
- Lee-Chiong T.L. Sleep: a comprehensive handbook. Wiley. 2006.
- Bromet E., et al. Cross-national epidemiology of DSM-IV major depressive episode // BMC medicine. 2011. V. 9. № 1. P. 90-106.
- Jennum P., Riha R.L. Epidemiology of sleep apnoea/hypo-pnoea syndrome and sleep-disordered breathing // European Respiratory Journal. 2009. V. 33. №. 4. P. 907-914.
- Redmond S.J., et al. Sleep staging using cardiorespiratory signals // Somnologie. 2007. V. 11. № 4. P. 245-256.
- Karlen W., Mattiussi C., Floreano D. Adaptive Sleep/Wake Classification Based on Cardiorespiratory Signals for Wearable Devices // IEEE Biomedical Circuits and Systems Conference. 2007. P. 203-206.
- Redmond S.J., Heneghan C. Cardiorespiratory-based sleep staging in subjects with obstructive sleep apnea // IEEE transactions on biomedical engineering. 2006. V. 53. № 3. P. 485-496.
- Adnane M., Jiang Z. Automatic sleep-wake stages classifier based on ECG // ICROS-SICE International Joint Conference. 2009. V. 2. P. 493-498.
- Ichimaru Y., Moody G.B. Development of the polysomnographic database on CD‐ROM // Psychiatry and Clinical Neurosciences. 2002. V. 53. №. 2. P. 175(177).
- Mendez M., et al. Sleep staging from Heart Rate Variability: time-varying spectral features and Hidden Markov Models // International Journal of Biomedical Engineering and Technology. 2010. V. 3. №3. P. 246-263.
- Yilmaz, B. et al. Sleep stage and obstructive apneaic epoch classification using single-lead ECG. // Biomedical engineering online. 2010. V. 9. P. 39-53.
- Goldberger A.L., et al. PhysioBank, PhysioToolkit, and PhysioNet: Components of a new research resource for complex physiologic signals // Circulation. 2000. V. 101. № 23. P. e215-e220.
- Hall M., et al. The WEKA data mining software: an update //ACM SIGKDD Explorations Newsletter. 2009. V. 11. №. 1. P. 10-18.
- Curk T., et al. Microarray data mining with visual programming // Bioinformatics. 2005. V. 21. №. 3. P. 396(398.
- Informaczionno-analiticheskij portal MachineLearning.ru [E'lektronny'j resurs]. URL: http://www.machinelearning.ru (data obrashheniya: 2.04.2013).
- Voronczov K.V. Lekczii po logicheskim algoritmam klassifikaczii [E'lektronny'j resurs]. M., 2007. URL: http://www.ccas.ru/voron/download/LogicAlgs.pdf (data obrashheniya: 2.04.2013).
- Rodriguez J.J., Kuncheva L.I., Alonso C.J. Rotation forest: A new classifier ensemble method // IEEE Transactions on Pattern Analysis and Machine Intelligence. 2006. V. 28. №. 10. P. 1619(1630.
- Breiman L. Random forests // Machine learning. 2001. V. 45. №. 1. P. 5(32.
- Petri A., Se'bin K. Naglyadnaya statistika v mediczine. M.: GE'OTAR-MED. 2003. S. 144.
- Shambroom J.R., Fábregas S.E., Johnstone J. Validation of an automated wireless system to monitor sleep in healthy adults // Journal of sleep research. 2012. V. 21. № 2. P. 221-230.