350 rub
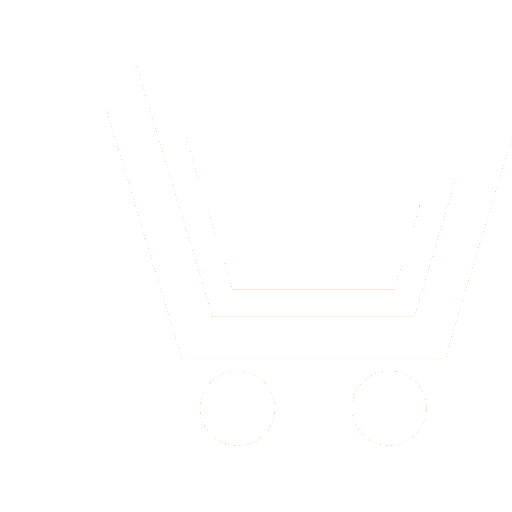
Journal Biomedical Radioelectronics №4 for 2013 г.
Article in number:
Estimation of information value of features used for echocardiography image processing
Keywords:
contouring left ventricle
automatic contouring
echocardiography
LV
classification
machine learning
decision tree
naive bayes
discriminant analysis
echocardiography video
Authors:
A.O. Bobkova, S.V. Porshnev, V.S. Kublanov
Abstract:
The paper presents results of estimating the parameters - informative properties of the heart left ventricle (LV) received by ultrasonography investigation images (USI-images). Moreover, application of machine-teaching methods to problem of the heart LV contouring is described.
The approach suggested is based on analysis of experience of expert doctors. The analysis shows that to construct the region of the heart LV, an expert leans on a set of low-level characteristics of each separate stop-shot and on specific details of variation of these parameters from a consequence of the shots. Thus, the problem of contouring the LV becomes equivalent to one of binary classification of pixels on shots of the echo-cardiograms.
In such a formulation, the heart LV position in each shot is given by the binary image, in which zero pixels correspond to the background, but the unit ones correspond to the heart LV region. It is necessary to note that, performing the construction of the heart LV contour, the expert actually implements the image procession, since, under presence of noises and distortions at the heart LV boundary, the expert ignores them and draws the boundary just as these noises would be absent. In the cases, when parts of the boundary are undistinguished, the expert reconstructs the contour on the basis of his own comprehension of an ideal contour. From this point of view, to solve the problem of automatic contouring the heart LV, it is necessary to analyze opportunity of application of various algorithms to image procession, which outline the important details of the image and delete inessential ones. As a result, individual opportunities of methods are estimated in distinguishing (separating) the background pixels from the heart LV ones. In the turn, it allows one to sift out the uninformative features and, further, to combine the residuary ones in the most optimal way for application them to the learning.
In the work, we use collections of video films in the learning and testing. Here, each collection can be divided into images of the following types: half-tone images that were received by the echo-cardiograms (series A); the binary images containing the heart LV region marked out by the expert (series B). In the binary images, pixels having the value 1 are joined with the heart LV region, but ones with the value 0 are referred to the background. Correspondingly, the problem of contouring the heart LV region is reduced to the problem of dividing the input half-tone images into two classes. The learning set has been formed on the basis of video films of ultrasonography investigation of four patients. The summary number of video shots for forming the learning set was 143. Thus, the summary quantity of pixels in the learning set was .
The testing set has been formed on the basis of video films of ultrasonography investigation of eleven patients. The summary number of video shots for forming the testing set was 299. Thus, the summary quantity of pixels in the testing set was .
Forty-three various characteristics of images were used as the pixel features. Analysis of parameters - informative properties allowed one to make a reasoned conclusion that all chosen parameters posses sufficient individual distinguishing ability.
It was found that it is necessary to choose different subsets of parameters on the learning set (the decision tree, discriminant function analysis, the naïve Byes classificatory, etc.) depending on the applied method. Under this, the part of classification errors achieves: on the basis of the decision tree it was 5.72%, on the basis of the discriminant function analysis it was 12.67%, and on the basis of the naïve Byes classificatory it was 4.8%.
Thus, it is possible to conclude that the decision tree method and the naïve Byes classificatory give similar results: in both methods the part of errors is lower of 6%. But taking into account that the power of the set of the classification parameters (features) in the decision tree method is nineteen but in the naïve Byes classificatory it is only six, application of the latter one to the problem under consideration is more preferable from the computational point of view.
Results of investigations described in the paper allow one to determine direction of the further researches. Namely, it is necessary to elaborate algorithms for additional procession aimed to deleting the distortions of the form of the distinguished binary regions corresponding to the heart LV.
Pages: 19-26
References
- Herlin I., Ayache N. Feature extraction and analysis methods for sequences of ultrasound images // In Proc. 2nd E.C.C.V. 1992. P. 43-55.
- Cohen L.D., Cohen I.Finite element methods for active contour models and balloons for 2-d and 3-d images // IEEE Trans. On Pattern Analysis and Machine Intelligence. 1993. V.15. P.1131-1147.
- Herlin I., Breziat D., Giraudin G., Nguyen C., Graffigne C. Segmentation of echocardiographic images with Markov random field // In Proc. 3nd E.C.C.V. 1994. P.201-206.
- Mailloux G. et al.Computer analysis of heart motion from two-dimensional echocardiograms // IEEE Trans. on Biomedical Engineering. 1987. V.34, №.5, P.356,
- Levashkina A.O., Porshnev S.V. Metody poiska izobrazhenijj na osnove vizualnogo skhodstva, LAPLAMBERTAcademicPublishing, 2011. 293 s.
- Armstrong W. F., Ryan Th., Feigenbaum H. Feigenbaum\'s Echocardiography. Lippincott Williams & Wilkins. 2010. 785 p.
- Smith M. Statistical Analysis Handbook // A free web-based statistics resource [http://www.statsref.com/]
- Porshnev S.V., Solomakha I.V. Issledovanie metodov analiza i prognozirovanija tekhnologicheskojj informacii, sobiraemojj informacionnymi sistemami teplovykh ehlektricheskikh stancijj. Ekaterinburg: UrFU. 2011. 208 s.
- Zagorujjko N.G.Prikladnye metody analiza dannykh i znanijj. Novosibirsk: IM SO RAN. 1999. 270 c.
- Levitin A.V.Algoritmy: vvedenie v razrabotku i analiz. M: Viljams. 2006. S.409-417.
- Borovikov V.P.Iskusstvo analiza dannykh, 2-e izd. SPb: PITER. 2005. 608 s.
- Subbotin S.V., Bolshakov D.JU. Primenenie bajjesovskogo klassifikatora dlja raspoznavanija klassov celejj // Radioehlektronika, 2006. № 4. Rezhim dostupa, svobodnyjj: http://jre.cplire.ru/jre/oct06/2/text.html (data dostupa 3.03.2013).