350 rub
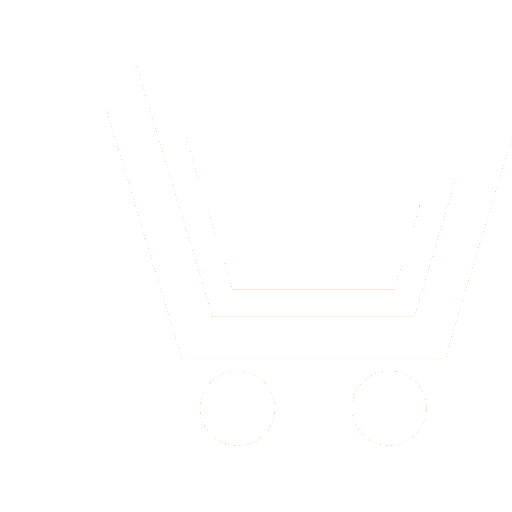
Journal Biomedical Radioelectronics №2 for 2013 г.
Article in number:
Algorithm for elimination of spatially uncorrelated EEG noise
Authors:
P.E. Volynsky, E.L. Masherov, G.A. Shekut-ev, Yu.V. Obukhov
Abstract:
EEG is widely used to studying brain activity. Digitizing of EEG recordings using modern equipment allows one to utilize a wide range of mathematical algorithms of digital signal processing. However, most of current digital analysis algorithms are sensitive to distortions of the original signal by non-physiological artifacts and noise.
A variety of different methods have been already proposed to solve this problem. However these either require direct involvement of the researcher or based on additional measurements, or cannot remove artifact activity to a sufficient extent. Here we propose a novel analysis method which is based on independence of the artifact signals in different EEG channels and on the representation of electrical activity in one channel through activity in other channel (cross-regression, CR). This method does not use any a priory information and thus is fully automated. Testing of the proposed method using model signals and real EEG recordings has demonstrated its effectiveness for elimination of the non-correlated EEG components such as electromyogram or movement artifacts.
Application of the CR analysis may lead to noise amplification in EEG channels that contain high amplitude long lasting artifact signals, especially when analysing short EEG recordings. Pre-processing of the EEG data using frequency filtration or amplitude thresholding normally allows eliminating such noise amplification. However this approach requires choosing a priory settings of threshold parameters for frequency or amplitude values. In order to remove such pre-processing step we tested a CR based Least Squares algorithm and a Least Amplitude Module algorithm. We demonstrate that the Least Amplitude Module algorithm allows artefact elimination without significant distortion of the source EEG signal. Thus we conclude that the Least Amplitude Module algorithm can be directly applied for artefact elimination without the pre-processing step.
Pages: 63-72
References
- Ille N., Berg P., Scherg M.Artifact correction of the ongoing EEG using spatial filters based on artifact and brain signal topographies// J. Clin. Neurophysiol. 2002. V. 19. № 2. P. 113-124.
- Schlögl A., Keinrath C., Zimmermann D., Scherer R., Leeb R., Pfurtscheller G.A fully automated correction method of EOG artifacts in EEG recordings // Clin Neurophysiologic.2007. V. 118. № 1. P. 98-104.
- Erfanian A., Mahmoudi B. Real-time ocular artifact suppression using recurrent neural network for electro-encephalogram based brain-computer interface // Med. Biol. Eng. Comput. 2005 V. 43. № 2. P. 296-305.
- Jiang J.A., Chao C.F., Chiu M.J., Lee R.G., Tseng C.L., Lin R.An automatic analysis method for detecting and eliminating ECG artifacts in EEG // Comput. Biol. Med. 2007. V. 37. № 11. P. 1660-1671.
- Пономарев В.А., Кропотов Ю.Д., Терещенко Е.П., Мюллер А.Сравнение эффективности различных методов удаления артефактов морганий при анализе количественной электроэнцефалограммы и вызванных потенциалов // Физиология человека. 2009. № 2.С. 124-131.
- James C.J., Hesse C.W. Independent component analysis for biomedical signals // Physiol. Meas. 2005. V. 26. № 1. P. R15-39.
- Vigário R., Särelä J., Jousmaki V., Hämäläinen M., Oja E. Independent component approach to the analysis of EEG and MEG recordings// IEEE Trans. Biomed. Eng. 2000. V. 47. № 5. P. 589.-93.
- Castellanos N.P., Makarov V.A.Recovering EEG brain signals: artifact suppression with wavelet enhanced independent component analysis // J. Neurosci Methods. 2006. V. 158. № 2. P. 300-312.
- James C.J., Gibson O.J.Temporally constrained ICA: an application to artifact rejection in electromagnetic brain signal analysis // IEEE Trans. Biomed. Eng. 2003. V. 50. № 9. P. 1108-1116.
- Ghandeharion H., Erfanian A.A fully automatic method for ocular artifact suppression from EEG data using wavelet transform and independent component analysis // Conf. Proc. IEEE Eng. Med. Biol. Soc. 2006. № 1. P. 5265-5268.
- LeVan P., Urrestarazu E., Gotman J.A system for automatic artifact removal in ictal scalp EEG based on independent component analysis and Bayesian classification // Clin. Neurophysiol. 2006. V. 117. № 4. P. 912-927.
- Buchthal F., Madsen A.Synchronous activity in normal and atrophic muscle // EEG and Clin. Neurophysiol. 1950. V. 2 № 4. P. 425-444.
- Дж. Себер.Линейный регрессионный анализ. М.: Мир. 1980. 456 с.
- Nelder J.A., Mead R. A simplex method for function minimization // The Computer Journal 1965. V. 7. № 4. P. 308-313.
- Press W.H., Teukolsky S.A., Vetterling W.T., Flannery B.P. 10.5 Downhill Simplex Method in Multidimensions. Numerical Recipes, 3rd Edition.Cambridge University Press. 2007. P. 502-507.
- Bell A.J., Sejnowski T.J.An information maximization approach to blind separation and blind deconvolution // Neural Computation. 1995. № 7. P. 1129-1159.
- Makeig S., Bell A.J., Jung T.-P., Sejnowski T.J.Independent component analysis of electroencephalographic data // Advances in Neural Information Processing Systems / Eds. D. Touretzky, M. Mozer, M. Hasselmo. Cambridge, MA: MIT Press. 1996. V. 8. P. 145-151.
- Makeig S., Westerfield W., Enghoff S.EEGlab: ICA Toolbox for Psychophysiological Research. WWW Site, Swartz Center
for Computational Neuroscience, Institute of Neural Computation, University of
San Diego California
, 2000. [World Wide Web Publication].