350 rub
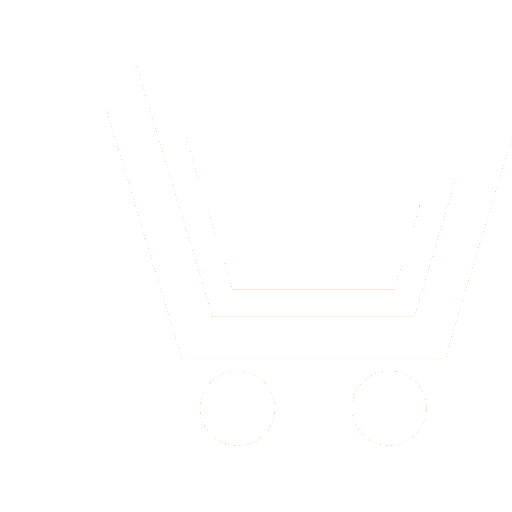
Journal Biomedical Radioelectronics №10 for 2013 г.
Article in number:
Neuro-fuzzy incidence forecasting models
Keywords:
prognosis
epidemy
data models
time series
incidence
scarlet fever
neuro-fuzzy networks
fuzzy logic
Authors:
Alexander Nikolaevich Dmitriev - Diploma student, Chair «Medical and technical information technologies», Faculty «Biomedical Engineering», Bauman Moscow State Technical University. E-mail: dmitalexnic@gmail.com
Vladimir Vladimirovich Kotin - Ph.D. (Phys.-Math.), Assistant Professor, Chair «Medical and technical information technologies», Faculty «Biomedical Engineering», Bauman Moscow State Technical University. Е-mail: v.kotin@gmail.com
Vladimir Vladimirovich Kotin - Ph.D. (Phys.-Math.), Assistant Professor, Chair «Medical and technical information technologies», Faculty «Biomedical Engineering», Bauman Moscow State Technical University. Е-mail: v.kotin@gmail.com
Abstract:
One of the essential problems of nowadays is developing new practically demanded models and methods of social science and mathematical epidemiology and their integration into the sphere of solving medical problems.
The aim of such research is raising the efficiency of planning the events to response to epidemics and improving the population dynamics.
Methods of computational and artificial intelligence can be applied to solve the problems of disease prediction.
The use of fuzzy sets for the prediction of disease dynamics allows to improve the validity and controllability of the management decision-making with the threat of epidemics.
Hybridization, or in other words, development and application of ensembles of models that interact with the aim of mutual compensation of disadvantages and limitations of each individual model, is an approach to solving a wide range of forecast problems of the incidence.
Fuzzy Systems with the paradigm of hybridization are used to analyze the data together with other methods of intelligent computing, such as multi-agent simulation, artificial neural networks, genetic algorithms, expert systems, etc., forming a useful alternative to dynamic models.
The main types of fuzzy models are Mamdani and TSK (Takagi-Sugeno-Kang), they have a modular structure, ideal for system representation in the form of a uniform multilayer structure resembling the structure of the classical neural networks. The package MATLAB Fuzzy Logic Toolbox neuro-fuzzy network are implemented in the form of adaptive neuro-fuzzy inference ANFIS (Adaptive Neuro-Fuzzy Inference System).
The model ANFIS is taken as the basic structure of the neuro-fuzzy system. To analyze the possibilities of fuzzy neural network prediction of disease, the data of the incidence of scarlet fever in Moscow in 1996-2008 was taken. Tests showed that the average relative error of prediction is 37%.
Pages: 55-59
References
- Leonenkov A.V. Nechetkoe modelirovanie v srede MATLAB i fuzzyTECH. SPb.: BXV-Peterburg. 2005. 736 s.
- Churyumova I.G. Sistema mediczinskoj diagnostiki na osnove nechetkoj logiki // Vostochno-Evropejskij zhurnal peredovy'x texnologij. 2006.
- Popov'yan P. Informaczionny'e texnologii v mediczinskom diagnostirovanii // Otkry'ty'e sistemy'. 2005. № 5 - 6.
- Py't'ev. Ju.P. Neopredelenny'e nechetkie modeli i ix primeneniya // Intellektual'ny'e sistemy'. 2004. T. 8. Vy'p. 1-4. S. 147-310.
- Chumachenko D.I., Sokolov A.A. Primenenie mul'tiagentny'x texnologij v zdravooxranenii // Sb. materialov Studencheskij nauchno-praktich. konf. s mezhdunar. uchastiem «Avtomatizacziya i komp'yuterno-integrirovanny'e texnologii. «Avtomatizacziya-2006». Xar'kov: XNADU. S. 26.
- Jarushkina N.G., Perfil'eva I.G., Afanas'eva T.V. Integracziya nechetkix modelej dlya analiza vremenny'x ryadov // Izvestiya Samarskogo nauchnogo czentra RAN. 2010. T. 12. №4(2). S. 506 - 509.
- Ry'zhko P.P. Soczial'naya informatika matematicheskix modelej e'pidemicheskix proczessov // Verecen'. 2006. № 3. S. 103 - 106.
- D'yachenko D.E. Intellektualizacziya e'kspertno-diagnosticheskogo proczessa na osnove nejrosetevogo modelirovaniya i nechetkoj logiki: Avtoref. diss. ... kand. texn. nauk. Voronezh: VGTU. 2004 125 s.
- Uskov A.A. Princzipy' postroeniya sistem upravleniya s nechetkoj logikoj // Pribory' i sistemy'. Upravlenie. Kontrol'. Diagnostika. 2004. № 6. S. 7-13.
- Prognozirovanie czen s pomoshh'yu nejronny'x setej. URL.2007.http://articles.mql4.com/ru/395 (Data obrashheniya 9.04.13)
- Laty'pov A.I., Radchenko S.V. Primenenie nechyotkoj logiki v differenczial'noj diagnostike stomatologicheskix zabolevanij // E'lektronny'j mediczinskij zhurnal. 2009.
- Brejkin T.V., Kamalova L.Z., Popkova S.Ja. i dr. Proektirovanie e'kspertny'x sistem mediczinskoj diagnostiki na baze nechetkoj logiki s primeneniem metodov sistemnogo modelirovaniya // Upravlenie v slozhny'x sistemax. Ufa. 1999. S. 127 - 134.
- Predstavlenie nechetkix znanij.URL.http://itteach.ru/predstavlenie-znaniy/predstavlenie-nechetkich-znaniy/vse-stranitsi (Data obrashheniya: 9.04.13)
- Nurmatova E.V. Primenenie adaptivnoj nejro-nechyotkoj sistemy' dlya modelirovaniya rejtingovoj oczenki znanij // Sb. dokl. 5-j Mezhdunar. nauchno-praktich. konf. «Integrirovanny'e modeli i myagkie vy'chisleniya v iskusstvennom intellekte». Kolomna. 2009.
- Kroshilin A.V., Kroshilin S.V., Kroshilina S.V. Primenenie semanticheskix setej, postroenny'x na nechetkix otnosheniyax, v sistemax podderzhki prinyatiya reshenij pri analize razvitiya problemny'x situaczij// Informaczionny'e resursy' Rossii. 2010. № 5 URL: http://www.aselibrary.ru/digital_resources/journal/irr/2010/number_5/number_52169/ number_521692170/ (Data obrashheniya 9.04.13)
- Krug P.G. Nejronny'e seti i nejrokomp'yutery'. M.: Izd-vo ME'I. 2002. 176 s.
- D'yakonov V., Kruglov V. Matematicheskie pakety' rasshireniya MATLAB. Speczial'ny'j spravochnik. SPb.: Piter. 2001. 480 s.
- Kopanicza G.D. Matematicheskoe i programmnoe obespechenie intellektual'noj informaczionnoj sistemy' dlya upravleniya set'yu lechebny'x uchrezhdenij: Avtoref. diss. ... kand. texn. nauk. Tomsk: NITPU. 2011. 125 s.
- Pokrovskij V.I., Briko N.I. Obshhaya e'pidemiologiya s osnovami dokazatel'noj medicziny'. Rukovodstvo k prakticheskim zanyatiyam. M.: Geotar-Media. 2008. 400 s.
- Dmitriev A.N., Kotin V.V. Modelirovanie vremenny'x ryadov zabolevaemosti s ispol'zovaniem iskusstvenny'x nejronny'x setej // Mediczinskaya texnika. 2013. № 1. S. 35-38.
- Shtovba S.D. Vvedenie v teoriyu nechetkix mnozhestv i nechetkuyu logiku. http://matlab.exponenta.ru/fuzzylogic/book1/index.php (Data obrashheniya 9.04.13)
- Agupov M.A., E'fendieva E'.M., Germashev I.V. Formirovanie obuchayushhej vy'borki dlya komp'yuternoj sistemy' analiza ximicheskix struktur // Sovremenny'e problemy' informatizaczii v e'konomike i obespechenii bezopasnosti. Voronezh: Nauchnaya kniga. 2012. S. 40-42.
- Mak-Kallok U.S., Pitts V. Logicheskoe ischislenie idej, otnosyashhixsya k nervnoj aktivnosti // V sb.: «Avtomaty'» / pod red. K.E'. Shennona i Dzh. Makkarti. M.: Izd-vo inostr. lit. 1956. S. 363-384.
- Kryuchin O.V., Kondrakov O.V. E'lektronny'j nauchny'j zhurnal «Issledovano v Rossii» http://zhurnal.ape.relarn.ru/articles/2010/082.pdf (Data obrashheniya 9.04.13)
- Bogomolov A.V., Gridin L.A., Kukushkin Ju.A., Ushakov I.B. Diagnostika sostoyaniya cheloveka. Matematicheskie podxody'. M.: Mediczina. 2003. 464 s.