350 rub
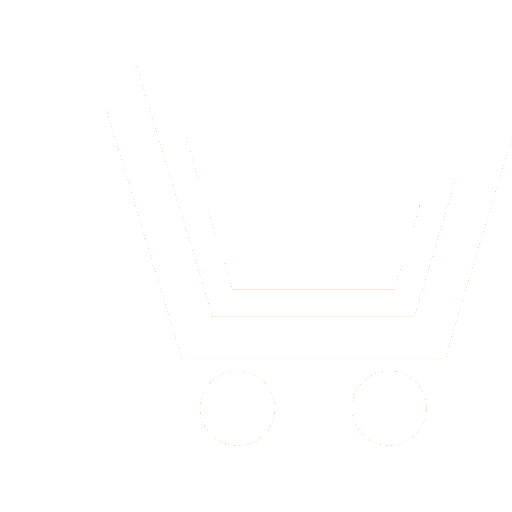
Journal Biomedical Radioelectronics №4 for 2012 г.
Article in number:
Neuronet models with hierarchical space of informative signs for segmentation of badly structured images
Authors:
R.A. Tomakova, A.A. Maslak, N.A. Shvetsova
Abstract:
The aim of work is the design of the hybrid computer technologies of segmentation of badly structured images with the help of structuring the space of the informative signs.
Models of the neural networks intended for segmentation of images on basis of RGB-codes of pixels are researched in present work. In the general case the neural network works as approximator and when the segment from the image is separated it has two exits. Considering that states - belong - not belong - in learning samples are coded by units and zero there are numbers on outputs of approximator laying in a range a zero - one which can be considered as certainty about belonging of the analyzed pixel to segment or «non segment» that is as fuzzy numbers. Thus approximating outputs of neural networks are considered as generators of fuzzy numbers. If these numbers come to aggregators and after to defuzzyficator then hierarchy of signs can be entered into classification system artificially in aggregators or it can be defined during the learning if two-input neural network can be used instead of defuzzyficator. The vector of hierarchical space of signs is dissected to subvectors for increasing of responsivity and specificity. The elements of subvectors do not form the hierarchical structure.
Fuzzy operations in aggregators set on outputs of neural networks are used for aprioristic input of hierarchy in model of a neural network. The experimental researches for separating of segments at images of an eye-ground shew the efficiency of such approach.
It is necessary to expand the space of signs of hierarchical models of neural networks with the help of entering of new subvectors of signs such as shape characteristics or spectral characteristics for the further increase of efficiency.
Pages: 56-62
References
- Кацнельсон Л.А., Лысенко В.С., Балишанская Т.И. Клинический атлас патологии глазного дна. М.: Изд-во ГЭОТАР-Медиа. 2008. 120 с.
- Филист С.А., Пихлап С.В., Томакова Р.А. Нечеткие нейросетевые структуры для сегментации изображений глазного дна // Вестник Воронежского государственного технического университета. 2009. Т.5. №4. С.42 - 45.
- Сафарли Н.Н., Щёголева И.В., Будзинская М.В., Кисе
лёва Т.Н., Киселев Г.Л.метод количественной оценки интенсивности флюоресценции в определении активности хориоидальной неоваскуляризации // Практикующий врач. 2007. № 6. С.101 - 102. - Коскас Г., Коскас Ф., Зурдан А. Комплексная диагностика патологии глазного дна. М.: Практическая медицина. 2007. 469 с.
- Хайкин С.Нейронные сети: полный курс. М.: ООО «И.Д. Вильямс». 2006. 1104 с