350 rub
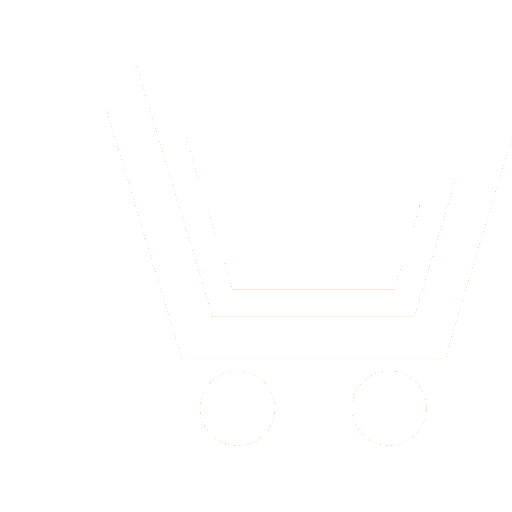
Journal Biomedical Radioelectronics №12 for 2010 г.
Article in number:
Estimation of the Importance of the Short-Term and Long-Term Dependence in Predicting of the Short-Term Dynamics of Physiological Rhythms
Keywords:
predicting
physiological rhythms
short-term dependence
long-term dependence
precursors
interval statistics
Authors:
M. I. Bogachev
Abstract:
The paper discussed two general approaches to estimate the probability that a physiological process consisting of consecutive heartbeat intervals exceeds a certain threshold. The first approach is based on searching for characteristic precursors of such events during the learning procedure, and later giving an alarm whenever a typical precursor appears during the online testing procedure. The learning procedure can be performed either using the available realizations of relevant physiological records, or on the mathematical model representing this process. As a learning model, both available realizations of heartbeat intervals and a well-known multiplicative random cascade model with 1/f power spectrum recently suggested for representation of physiological rhythms are used. This first approach is usually called the precursory pattern recognition technique and exploits solely short-term memory. The second approach is based on the return interval statistics between large events and thus is able to exploit long-term memory. The paper shows that the second approach yields at least of the same efficiency being tested on the simulated records, obtained using the multiplicative random cascade model. In the real 24-hour heartbeat interval records, the second approach is clearly superior to the first approach. It is shown that the same result can be obtained in the simulated records in the presence of measurement noise, due to the better noise robustness of the second approach. Another advantage of the second approach is that it does not require extensive learning or tuning procedures and thus is easier to implement. The paper suggests possible applications of the same techniques to other physiological rhythms, such as blood pressure and some parameters of the ECG like S-T segment level and slope. Furthermore, combining information obtained using both approaches in order to improve the overall predictability is suggested.
Pages: 3-11
References
- Ivanov P.C., Rosenblum M.G., Amaral L.A., Struzik Z., Havlin S., Golberger A.L., Stanley H.E. Multifractality in human heartbeat dynamics // Nature. 1996. V. 399. P. 461.
- Ashkenazy Y., Lewkowicz M., Levitan J., Havlin S., Saermark K., Moelgaard H., Bloch Thomsen P.E., Moller M., Hintzel U., Huikuri H.V. Scale specific and scale independent measures of heart rate variability as risk indicators // Europhys. Lett. 2001. V. 53. P. 709.
- Pikkujamsa S.M., Makikallio T.H., Sourander L.B., Raiha I.J., Puukka P., Skytta J., Peng C.-K., Goldberger A.L, Huikuri H.V., Huikuri H.V. Cardiac heartbeat intervals from childhood to senescence: Comparison of conventional and new measures based on fractals and chaos theory // Circulation. 1999. V. 100. P. 303.
- Turcott R.G., Teich M.C. Fractal character of the electrocardiogram: Distinguishing between heart-failure and normal patients // Ann. Biomed. Eng. V. 24. 1996. P. 269.
- Hausdorff J.M., Purdon P.L., Peng C.-K., Ladin Z., Weil J.Y., Goldberger A.L. Fractal dynamics of human gait: Stability of long-range correlations in stride universal fluctuations // J. Appl. Physiol. V. 80. 1996. P. 1448.
- Goldberger A.L., Amaral L.A., Hausdorff J.M., Ivanov P.C., Peng C.-K., Stanley H.E. Fractal dynamics in physiology: Alternations with disease and aging // Proc. Nat. Acad. Sci. U.S.A. 2002. V. 99. P. 2466.
- Ivanov P.C., Amaral L.A.N., Goldberger A.L., Havlin S., Rosenblum M.G., Stanley H.E., Struzik Z.R. From 1/f noise to multifractal cascades in heartbeat dynamics // Chaos. 2001. V. 11. P. 641.
- Hallerberg S., AltmannE.G., HolsteinD., KantzH. Precursors of extreme increments // Phys. Rev. E. 2007. V. 75. Р. 016706.
- Bunde A., Eichner J.F., Kantelhardt J.W., Havlin S. Return intervals of rare event in records with long-term persistence // Physica A. 2004. V. 342. P. 308 - 314.
- Eichner J.F., Kantelhardt J.W., Bunde A., Havlin S. Statistics of return intervals in long-term correlated records // Phys. Rev. E. 2007. V. 75. P. 011128.
- Stefanovska A., Lotric M. B., Strle S., Haken H. The cardiovascular system as coupled oscillators // Physiol. Meas. 2001. V. 22. P. 535-550.
- Bogachev M.I., Eichner J.F., Bunde A. Effect of nonlinear correlations on the statistics of return intervals in multifractal data sets // Phys. Rev. Lett. 2007. V. 99. P. 240601.
- Bogachev M.I., Eichner J.F., Bunde A. Effect of multifractality on the statistics of return intervals // Eur. Phys. J. - Spec. Topics. 2008. V. 161. P. 181-193.
- Bogachev M.I., Eichner J.F., Bunde A. On the occurrence of extreme events in long-term correlated and multifractal data sets // Pure Appl. Geophys. 2008. V. 165. P. 1195-1207.
- Kantelhardt J.W., Zschiegner S.A., Koscielny-Bunde E., Havlin S., Bunde A., Stanley H.E. Multifractal detrended fluctuation analysis of nonstationary time series // Physica A. 2002. V. 316. P. 87-114.
- Богачев М.И. Статистический анализ и прогнозирование динамики случайных процессов в телекоммуникационных сетях с использованием мультифрактальных моделей трафика // Известия вузов России. Сер. Радиоэлектроника. 2008. №2. С. 34-45.